Recursive Neural Networks Applied to Discourse Representation Theory
ICANN(2002)
摘要
Connectionist semantic modeling in natural language processing (a typical symbolic domain) is still a challenging problem. This paper introduces a novel technique, combining Discourse Representation Theory (DRT) with Recursive Neural Networks (RNN) in order to yield a neural model capable to discover properties and relationships among constituents of a knowledge-base expressed by natural language sentences. DRT transforms sequences of sentences into directed ordered acyclic graphs, while RNNs are trained to deal with such structured data. The acquired information allows the network to reply on questions, the answers of which are not directly expressed into the knowledge-base. A simple experimental demonstration, drawn from the context of a fairy tales is presented. Finally, on-going research direction are pointed-out.
更多查看译文
关键词
acquired information,acyclic graph,natural language sentence,fairy tale,recursive neural networks applied,connectionist semantic modeling,natural language processing,challenging problem,discourse representation theory,recursive neural networks,neural model,natural language,semantic model,knowledge base,structured data
AI 理解论文
溯源树
样例
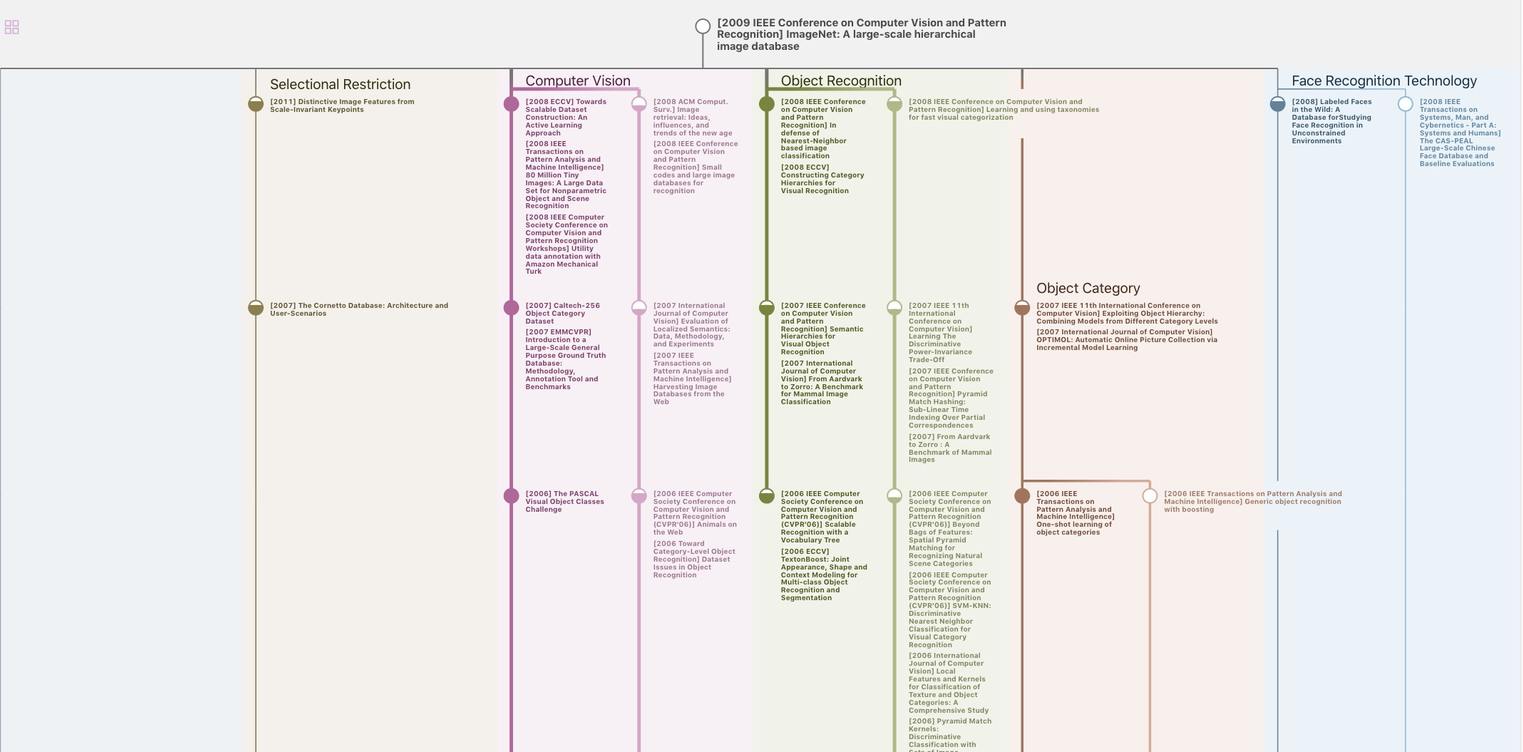
生成溯源树,研究论文发展脉络
Chat Paper
正在生成论文摘要