Minimum Relative Entropy State Transitions In Discrete Time Systems With Statistically Uncertain Noise
49TH IEEE CONFERENCE ON DECISION AND CONTROL (CDC)(2010)
摘要
We develop a stochastic dissipativity theory for discrete-time systems driven by an uncertain random noise. The deviation of the unknown probability law of the noise from a nominal white noise distribution is quantified by the conditional relative entropy given the initial state of the system. We establish a dissipation inequality and superadditivity property for the conditional relative entropy supply. The problem of minimizing the supply required to drive the system between given state distributions over a specified time horizon is considered. We obtain a dynamic programming Bellman equation for the minimum required relative entropy supply and show that the optimal noise is Markov with respect to the state of the system. For linear systems with Gaussian nominal noise and Gaussian initial and terminal state distributions, computing the minimum required supply is reduced to solving an algebraic Riccati equation.
更多查看译文
关键词
state transition,distributed computing,relative entropy,statistical analysis,bellman equation,probability distribution,covariance matrix,dynamic programming,linear system,gaussian distribution,markov processes,algebraic riccati equation,entropy,noise,linear systems,white noise
AI 理解论文
溯源树
样例
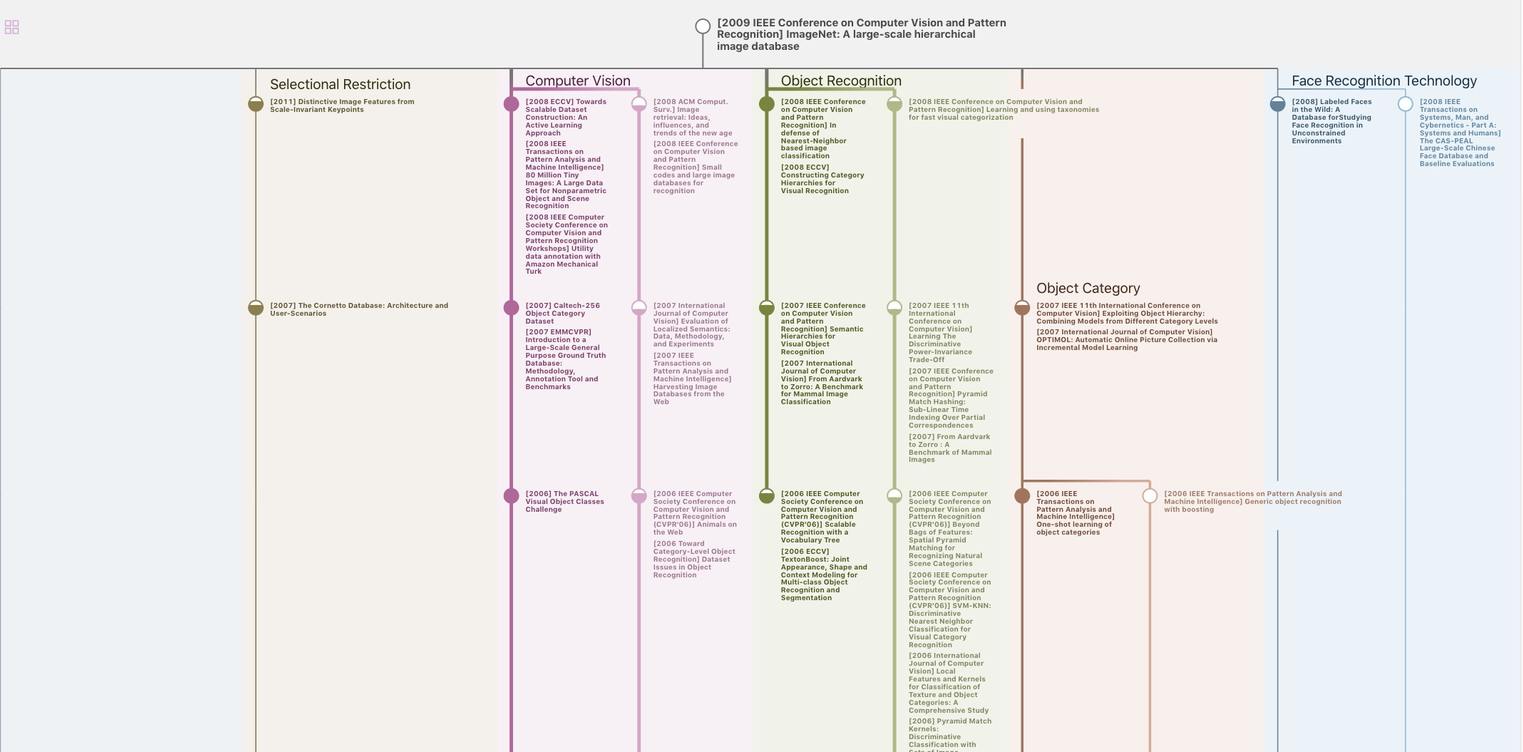
生成溯源树,研究论文发展脉络
Chat Paper
正在生成论文摘要