Classifying Learner Engagement through Integration of Multiple Data Sources.
AAAI'06: Proceedings of the 21st national conference on Artificial intelligence - Volume 1(2006)
摘要
Intelligent tutoring systems (ITS) can provide effective instruction, but learners do not always use such systems effectively. In the present study, high school students' action sequences with a mathematics ITS were machine-classified into five finite-state machines indicating guessing strategies, appropriate help use, and independent problem solving; over 90% of problem events were categorized. Students were grouped via cluster analyses based on self reports of motivation. Motivation grouping predicted ITS strategic approach better than prior math achievement (as rated by classroom teachers). Learners who reported being disengaged in math were most likely to exhibit appropriate help use while working with the ITS, relative to average and high motivation learners. The results indicate that learners can readily report their motivation state and that these data predict how learners interact with the ITS.
更多查看译文
关键词
appropriate help use,learners interact,high motivation learner,motivation grouping,motivation state,high school student,independent problem,prior math achievement,problem event,action sequence,learner engagement,multiple data source
AI 理解论文
溯源树
样例
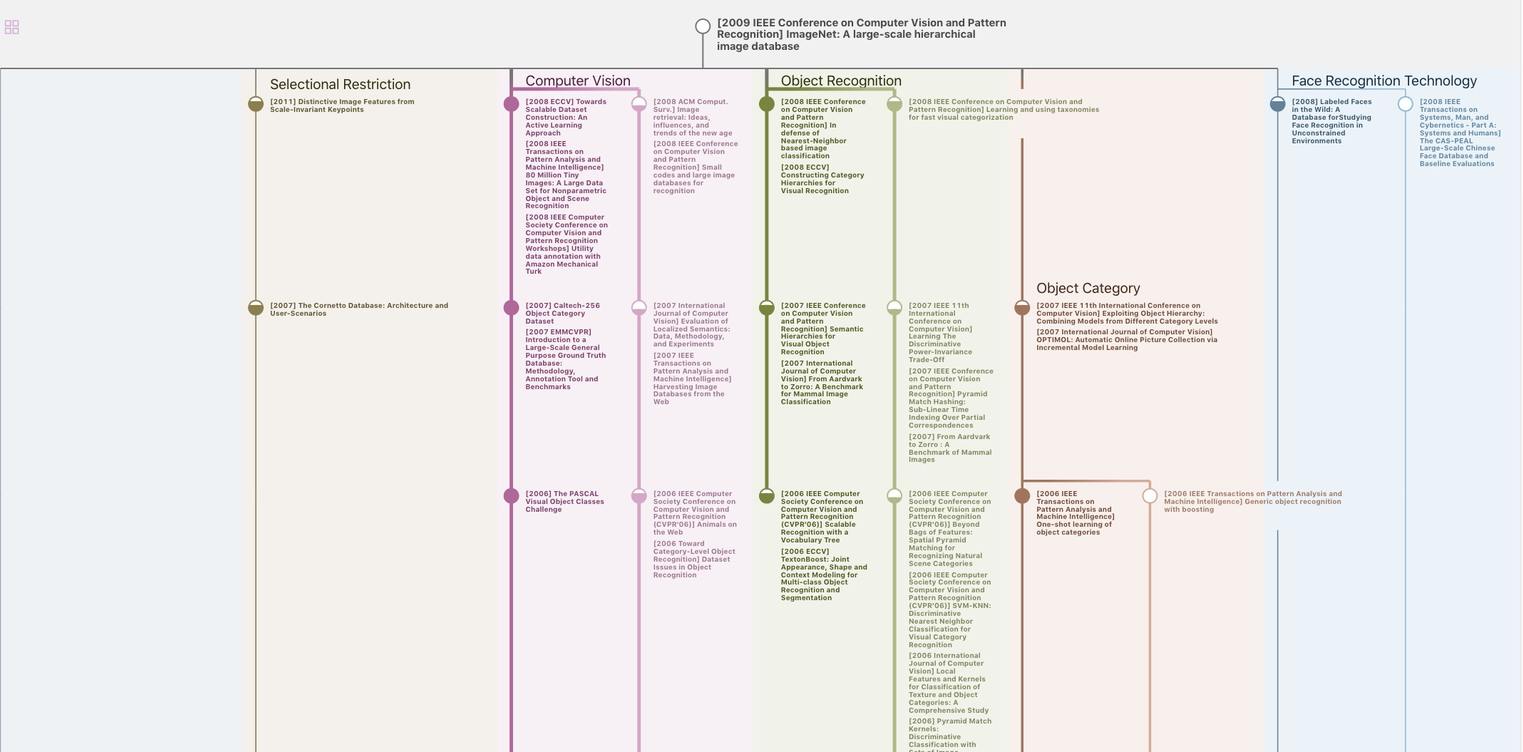
生成溯源树,研究论文发展脉络
Chat Paper
正在生成论文摘要