Sequential Learning for Optimal Monitoring of Multi-channel Wireless Networks
INFOCOM(2011)
摘要
We consider the problem of optimally assigning p sniffers to K
channels to monitor the transmission activities in a multi-channel wireless
network. The activity of users is initially unknown to the sniffers and is to
be learned along with channel assignment decisions while maximizing the benifits of this assignment,
resulting in the fundamental trade-off between exploration versus exploitation. We formulate it as the
linear partial monitoring problem, a super-class of multi-armed bandits. As the number of
arms (sniffer-channel assignments) is exponential, novel techniques are called for, to allow efficient learning.
We use the linear bandit model to capture the dependency amongst the arms and develop two policies that take advantage of this dependency.
Both policies enjoy logarithmic regret bound of time-slots with a term that is sub-linear in the number of
arms.
更多查看译文
关键词
multi armed bandit,resource management,uncertainty,channel allocation,learning artificial intelligence,resource manager,wireless networks,wireless network,sequential learning
AI 理解论文
溯源树
样例
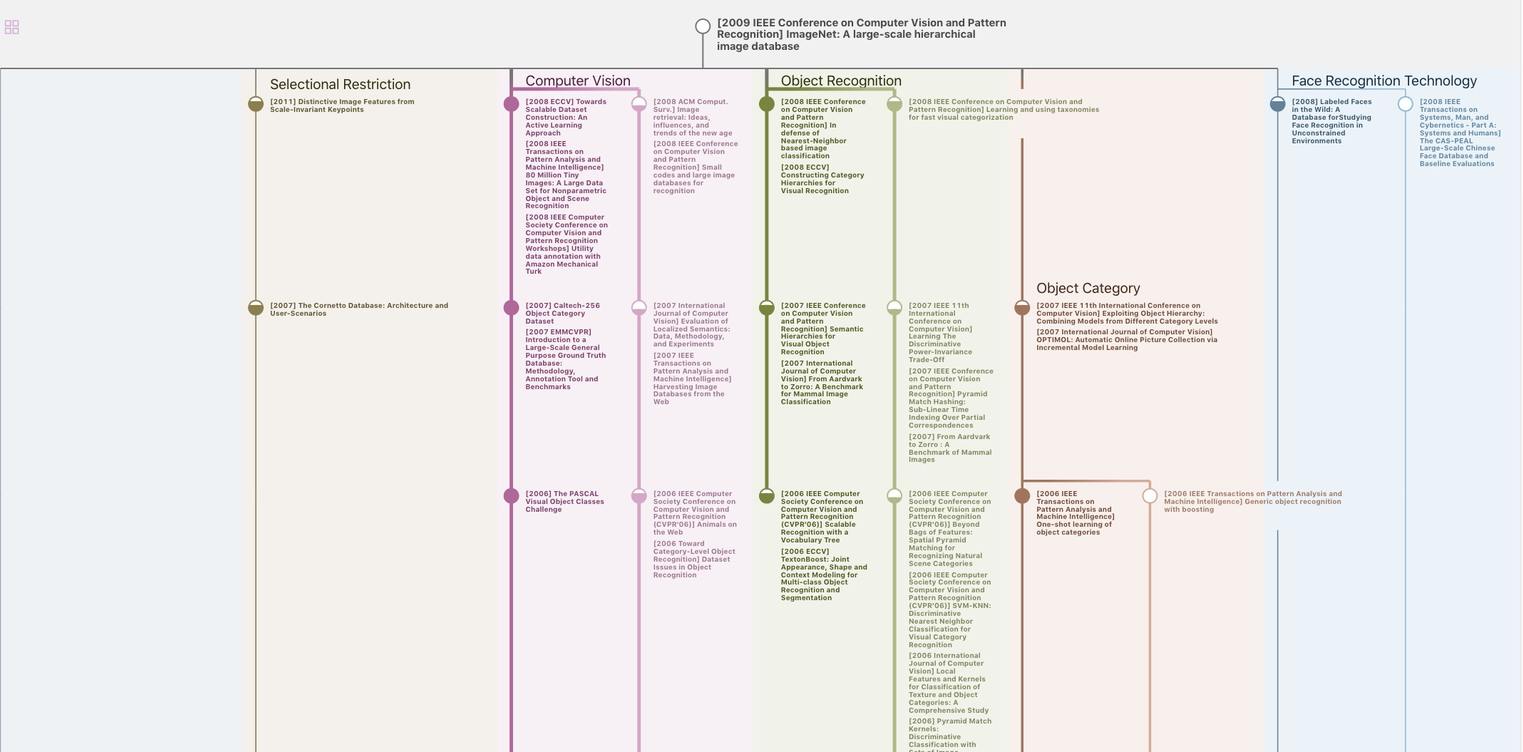
生成溯源树,研究论文发展脉络
Chat Paper
正在生成论文摘要