Second-Order Cyclostationarity of BT-SCLD Signals: Theoretical Developments and Applications to Signal Classification and Blind Parameter Estimation
Wireless Communications, IEEE Transactions(2013)
摘要
This paper investigates the second-order cyclostationarity of block transmitted-single carrier linearly digitally modulated (BT-SCLD) signals, and its applications to signal classification and blind (non-data aided) parameter estimation. Analytical closed-form expressions are derived for the cyclic autocorrelation function (CAF), cyclic spectrum (CS), complementary CAF (CCAF), complementary CS (CCS), and corresponding cycle frequencies (CFs). Furthermore, the conditions for avoiding aliasing in the cycle and spectral frequency domains are obtained. Based on these findings, we propose algorithms for classifying BTSCLD, orthogonal frequency division multiplexing (OFDM), and SCLD signals, and for the blind estimation of the BT-SCLD block transmission parameters. Simulation and laboratory experiments demonstrate the effectiveness of the proposed algorithms under low signal-to-noise ratios (SNRs), short sensing times, and various channel conditions. Furthermore, these algorithms have the advantage of not requiring the recovery of carrier, waveform, and symbol timing information, or the estimation of signal and noise powers.
更多查看译文
关键词
OFDM modulation,parameter estimation,signal classification,BT-SCLD block transmission parameters,BT-SCLD signals,OFDM,SNR,analytical closed-form expressions,blind parameter estimation,block transmitted-single carrier linearly digitally modulated signals,channel conditions,complementary CAF,cycle frequencies,cyclic autocorrelation function,cyclic spectrum,noise powers,nondata aided parameter estimation,orthogonal frequency division multiplexing,second-order cyclostationarity,signal classification,signal estimation,signal-to-noise ratios,spectral frequency domains,theoretical developments,Alamouti space-time block code,Signal classification,fourth-order statistics,spatial multiplexing
AI 理解论文
溯源树
样例
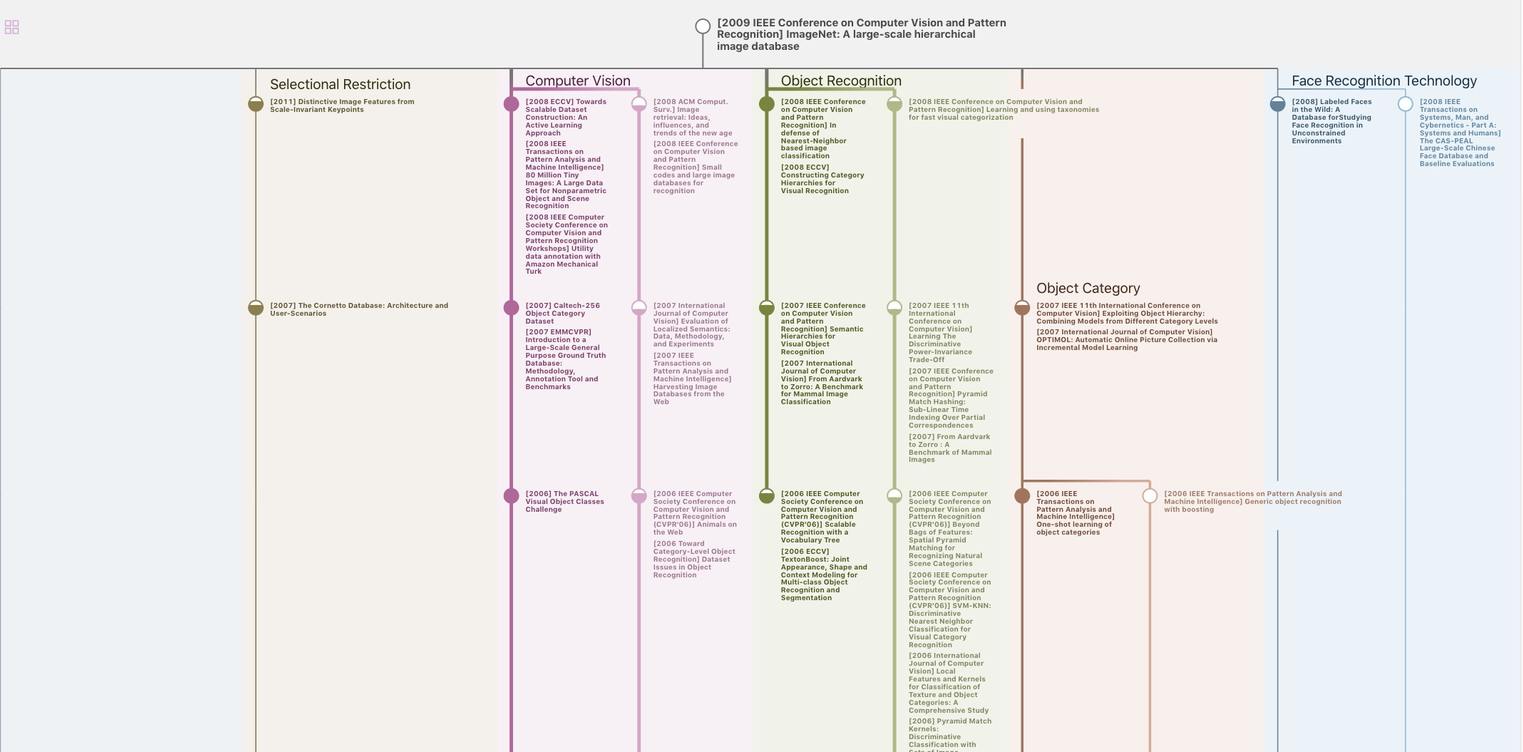
生成溯源树,研究论文发展脉络
Chat Paper
正在生成论文摘要