Sharpening Wald-type inference in robust regression for small samples
Computational Statistics & Data Analysis(2013)
摘要
The datasets used in statistical analyses are often small in the sense that the number of observations n is less than 5 times the number of parameters p to be estimated. In contrast, methods of robust regression are usually optimized in terms of asymptotics with an emphasis on efficiency and maximal bias of estimated coefficients. Inference, i.e., determination of confidence and prediction intervals, is proposed as complementary criteria. An analysis of MM-estimators leads to the development of a new scale estimate, the Design Adaptive Scale Estimate, and to an extension of the MM-estimate, the SMDM-estimate, as well as a suitable @j-function. A simulation study shows and a real data example illustrates that the SMDM-estimate has better performance for small n/p and that the use the new scale estimate and of a slowly redescending @j-function is crucial for adequate inference.
更多查看译文
关键词
design adaptive scale estimate,robust inference,mm-estimator,adequate inference,small sample,robust regression,sharpening wald-type inference,complementary criterion,prediction interval,new scale estimate,maximal bias,estimated coefficient,small n,better performance,observations n,marketing
AI 理解论文
溯源树
样例
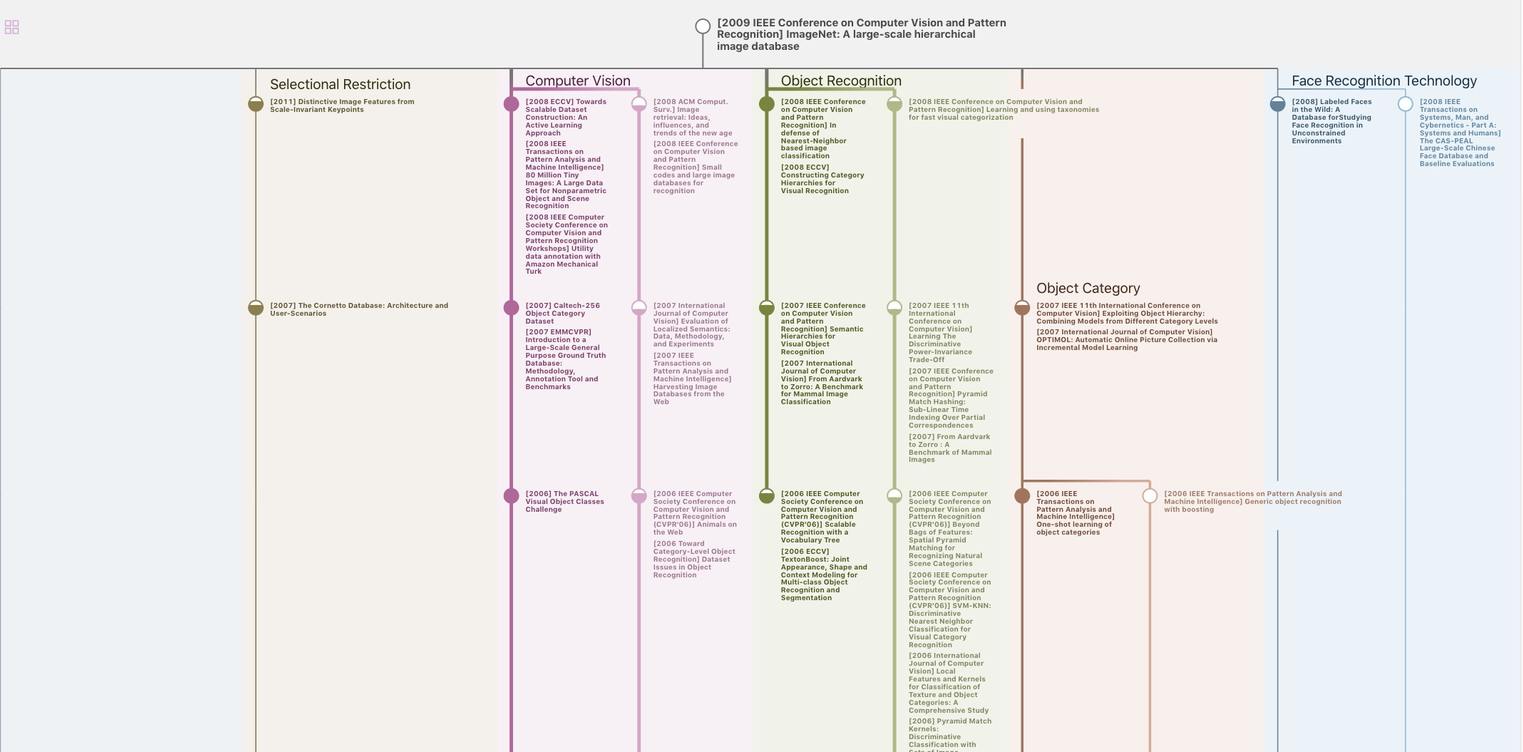
生成溯源树,研究论文发展脉络
Chat Paper
正在生成论文摘要