Unsupervised Combination Of Metrics For Semantic Class Induction
2006 IEEE SPOKEN LANGUAGE TECHNOLOGY WORKSHOP(2006)
摘要
In this paper, unsupervised algorithms for combining semantic similarity metrics are proposed for the problem of automatic class induction. The automatic class induction algorithm is based on the work of Pargellis et al [1]. The semantic similarity metrics that are evaluated and combined are based on narrow- and wide-context vector-product similarity. The metrics are combined using linear weights that are computed 'on the fly' and are updated at each iteration of the class induction algorithm, forming a corpus-independent metric. Specifically, the weight of each metric is selected to be inversely proportional to the inter-class similarity of the classes induced by that metric and for the current iteration of the algorithm. The proposed algorithms are evaluated on two corpora: a semantically heterogeneous news domain (HR-Net) and an application-specific travel reservation corpus (ATIS). It is shown, that the (unsupervised) adaptive weighting scheme outperforms the (supervised) fixed weighting scheme. Up to 50% relative error reduction is achieved by the adaptive weighting scheme.
更多查看译文
关键词
text processing,information retrieval,ontology creation
AI 理解论文
溯源树
样例
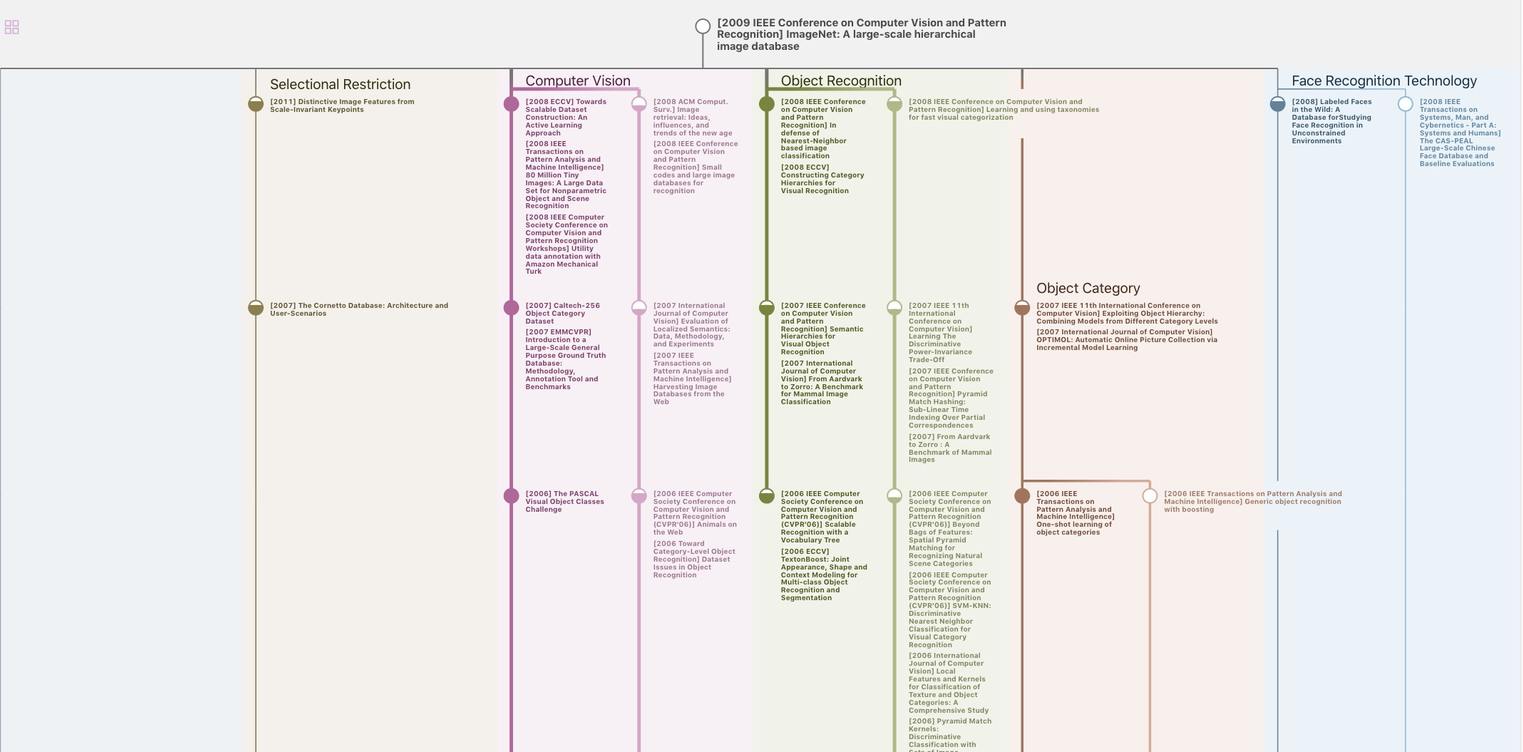
生成溯源树,研究论文发展脉络
Chat Paper
正在生成论文摘要