Symbol acquisition for task-level planning
AAAIWS'13-12: Proceedings of the 12th AAAI Conference on Learning Rich Representations from Low-Level Sensors(2013)
摘要
We consider the problem of how to plan efficiently in low-level, continuous state spaces with temporally abstract actions (or skills), by constructing abstract representations of the problem suitable for task-level planning. The central question this effort poses is which abstract representations are required to express and evaluate plans composed of sequences of skills. We show that classifiers can be used as a symbolic representation system, and that the ability to represent the preconditions and effects of an agent's skills is both necessary and sufficient for task-level planning. The resulting representations allow a reinforcement learning agent to acquire a symbolic representation appropriate for planning from experience.
更多查看译文
AI 理解论文
溯源树
样例
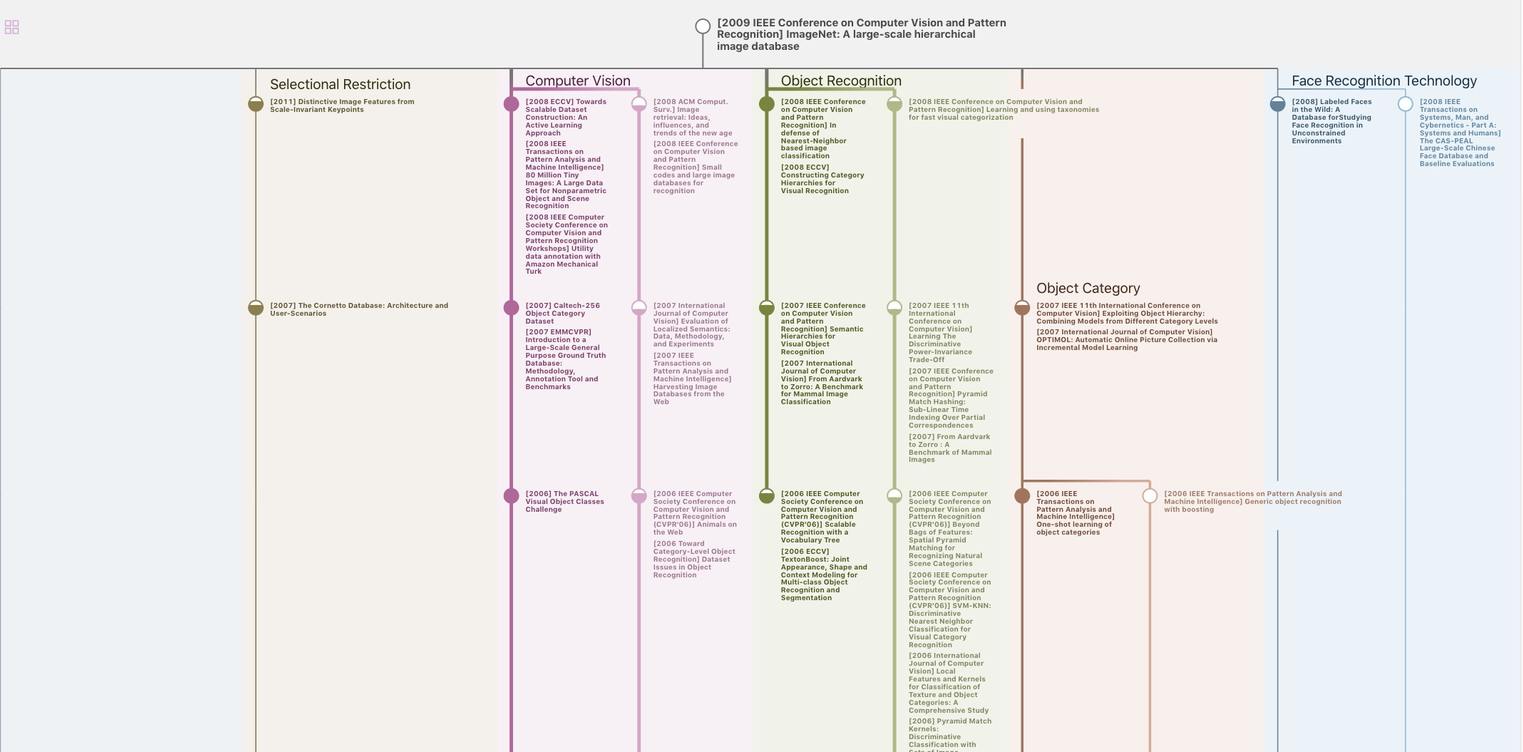
生成溯源树,研究论文发展脉络
Chat Paper
正在生成论文摘要