Comparison of methods for language-dependent and language-independent query-by-example spoken term detection
ACM Trans. Inf. Syst.(2012)
摘要
This article investigates query-by-example (QbE) spoken term detection (STD), in which the query is not entered as text, but selected in speech data or spoken. Two feature extractors based on neural networks (NN) are introduced: the first producing phone-state posteriors and the second making use of a compressive NN layer. They are combined with three different QbE detectors: while the Gaussian mixture model/hidden Markov model (GMM/HMM) and dynamic time warping (DTW) both work on continuous feature vectors, the third one, based on weighted finite-state transducers (WFST), processes phone lattices. QbE STD is compared to two standard STD systems with text queries: acoustic keyword spotting and WFST-based search of phone strings in phone lattices. The results are reported on four languages (Czech, English, Hungarian, and Levantine Arabic) using standard metrics: equal error rate (EER) and two versions of popular figure-of-merit (FOM). Language-dependent and language-independent cases are investigated; the latter being particularly interesting for scenarios lacking standard resources to train speech recognition systems. While the DTW and GMM/HMM approaches produce the best results for a language-dependent setup depending on the target language, the GMM/HMM approach performs the best dealing with a language-independent setup. As far as WFSTs are concerned, they are promising as they allow for indexing and fast search.
更多查看译文
关键词
term detection,standard metrics,gaussian mixture model,hmm approach,phone lattice,different qbe detector,standard resource,language-independent query-by-example,standard std system,phone string,wfst-based search,qbe std,query by example
AI 理解论文
溯源树
样例
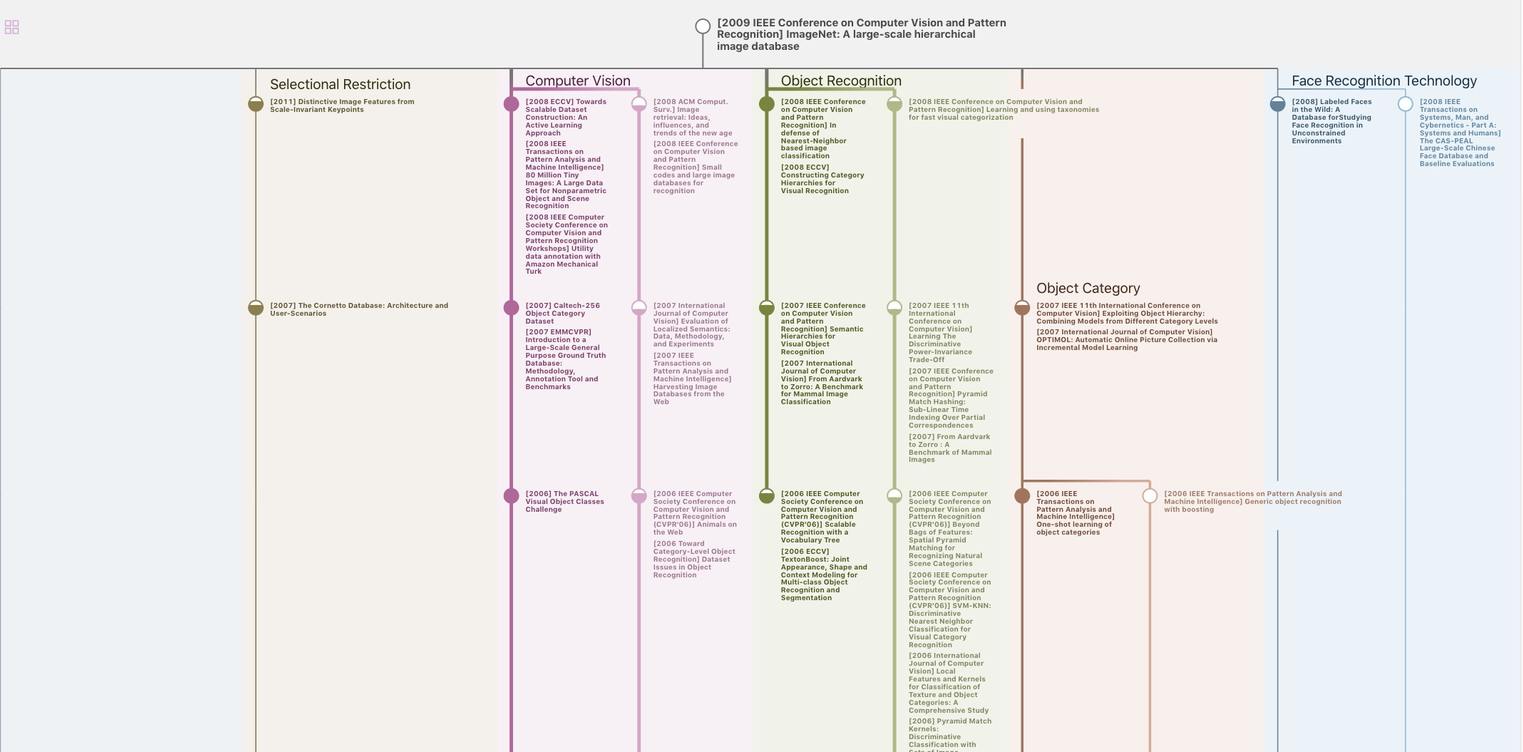
生成溯源树,研究论文发展脉络
Chat Paper
正在生成论文摘要