Kernel-based distance metric learning in the output space
IJCNN(2013)
摘要
In this paper we present two related, kernel-based Distance Metric Learning (DML) methods. Their respective models non-linearly map data from their original space to an output space, and subsequent distance measurements are performed in the output space via a Mahalanobis metric. The dimensionality of the output space can be directly controlled to facilitate the learning of a low-rank metric. Both methods allow for simultaneous inference of the associated metric and the mapping to the output space, which can be used to visualize the data, when the output space is 2-or 3-dimensional. Experimental results for a collection of classification tasks illustrate the advantages of the proposed methods over other traditional and kernel-based DML approaches.
更多查看译文
关键词
nonlinearly map data,learning (artificial intelligence),low-rank metric,mahalanobis metric,subsequent distance measurement,dml method,classification task,kernel-based distance metric learning,learning artificial intelligence
AI 理解论文
溯源树
样例
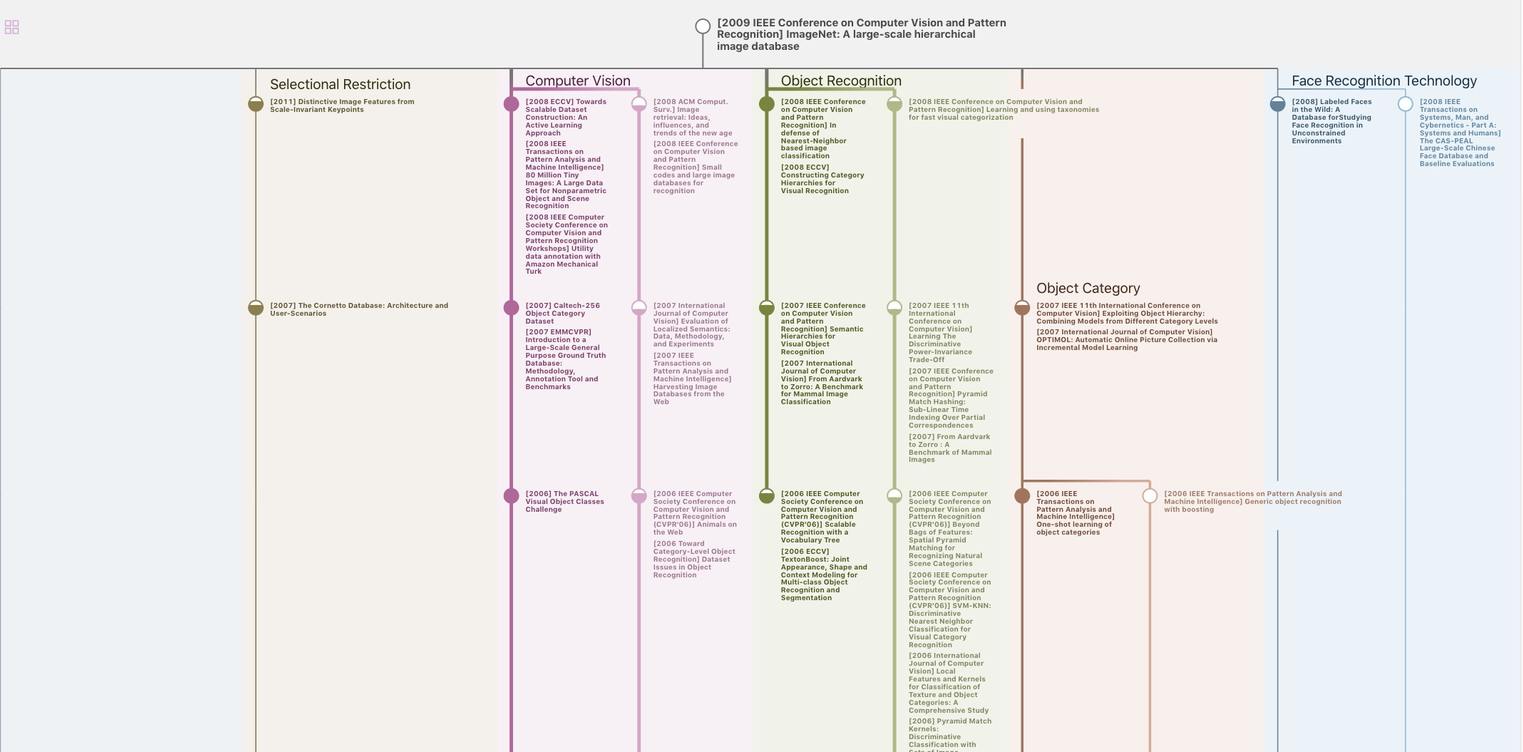
生成溯源树,研究论文发展脉络
Chat Paper
正在生成论文摘要