Probabilistic inference for solving (PO)MDPs
msra(2006)
摘要
The development of probabilistic inference techniques has made considerable progress in re- cent years, in particular with respect to exploiting the structure (e.g., factored, hierarchical or relational) of discrete and continuous problem domains. We show that these techniques can be used also for solving Markov Decision Processes (MDPs) or partial observable MDPs (POMDPs) when formulated in terms of a structured dynamic Bayesian network (DBN). The approach is based on an equivalence between maxi- mization of the expected future return in the time-unlimited MDP and likelihood maximization in a related mixture of finite-time MDPs. This allows us to use expectation maximization (EM) for computing optimal policies, using arbitrary inference techniques in the E-step. Unlike previous approaches we can show that this actually optimizes the discounted expected future return for arbitrary reward functions and without assuming an ad hoc finite total time. We first develop the approach for standard MDPs and demonstrate it using exact inference on a discrete maze and Gaussian belief state propagation in non-linear stochastic optimal control problems. Then we present an extension for solving POMDPs. We consider an agent model that includes an internal memory variable used for gating reactive behaviors. Using exact inference on the respective DBN, the EM-algorithm solves complex maze problems by learning appropriate internal memory representations.
更多查看译文
关键词
: markov decision process,planning,pomdp,probabilistic inference
AI 理解论文
溯源树
样例
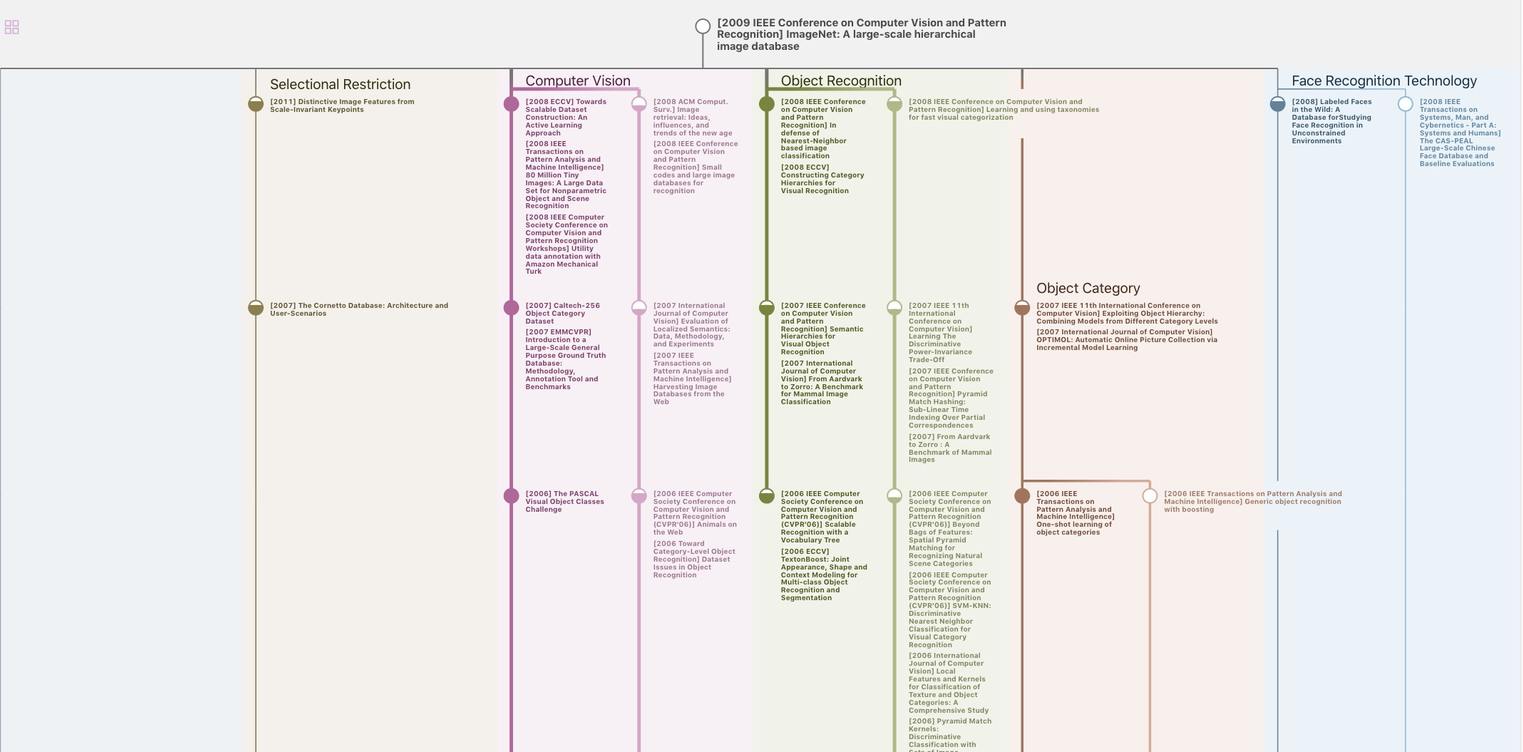
生成溯源树,研究论文发展脉络
Chat Paper
正在生成论文摘要