Satisfiability advancements enabled by state machines
Satisfiability advancements enabled by state machines(2012)
摘要
This dissertation focuses on research for state-based Satisfiability (SAT), a variant of SAT that uses state machines (Smurfs) to represent constraints. Using this constraint representation allows for compact representations of SAT problem instances that retain more ungarbled user-domain information than other more common representations such as Conjunctive Normal Form (CNF). State-base SAT also supports earlier inference deduction during search, the use of powerful search heuristics, and the integration of special purpose constraints and solvers.SBSAT, a state-based SAT research platform, was used and enhanced for both researching the new techniques presented here and gathering experimental data. Since the power of state-based SAT is diminished on problems naturally represented in CNF, the benchmarks used to collect results focus on domains with rich constraints such as verification and model checking.
更多查看译文
关键词
State-base SAT,powerful search heuristics,Satisfiability advancement,constraint representation,SAT problem instance,state machine,common representation,compact representation,Conjunctive Normal Form,state-based Satisfiability,state-based SAT,state-based SAT research platform
AI 理解论文
溯源树
样例
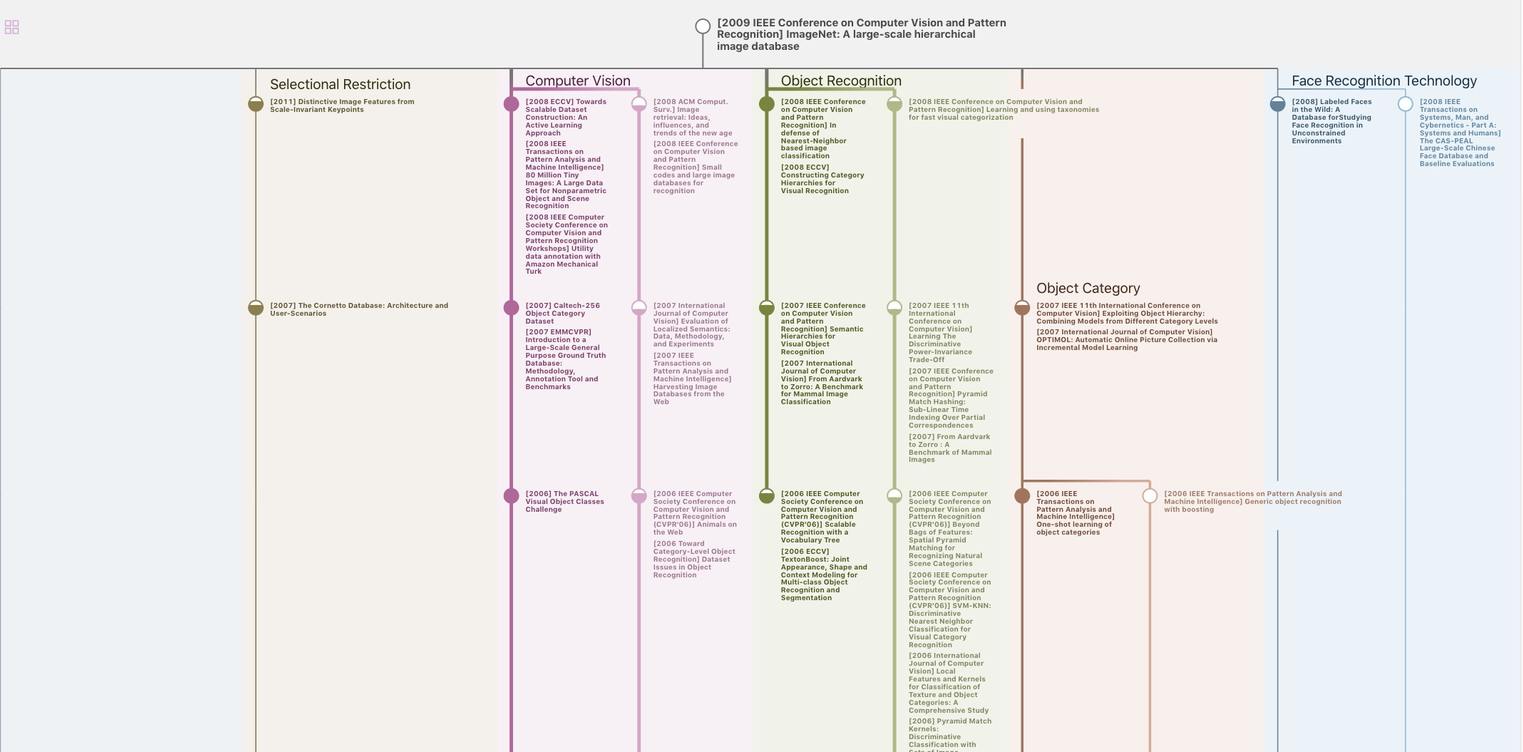
生成溯源树,研究论文发展脉络
Chat Paper
正在生成论文摘要