Log-Linear Convergence and Divergence of the Scale-Invariant (1+1)-ES in Noisy Environments
Algorithmica(2010)
摘要
Noise is present in many real-world continuous optimization problems. Stochastic search algorithms such as Evolution Strategies (ESs) have been proposed as effective search methods in such contexts. In this paper, we provide a mathematical analysis of the convergence of a (1+1)-ES on unimodal spherical objective functions in the presence of noise. We prove for a multiplicative noise model that for a positive expected value of the noisy objective function, convergence or divergence happens depending on the infimum of the support of the noise. Moreover, we investigate convergence rates and show that log-linear convergence is preserved in presence of noise. This result is a strong theoretical foundation of the robustness of ESs with respect to noise.
更多查看译文
关键词
Numerical optimization,Noisy optimization,Stochastic optimization algorithms,Evolution strategies,Convergence,Convergence rates,Markov chains,Borel-Cantelli lemma
AI 理解论文
溯源树
样例
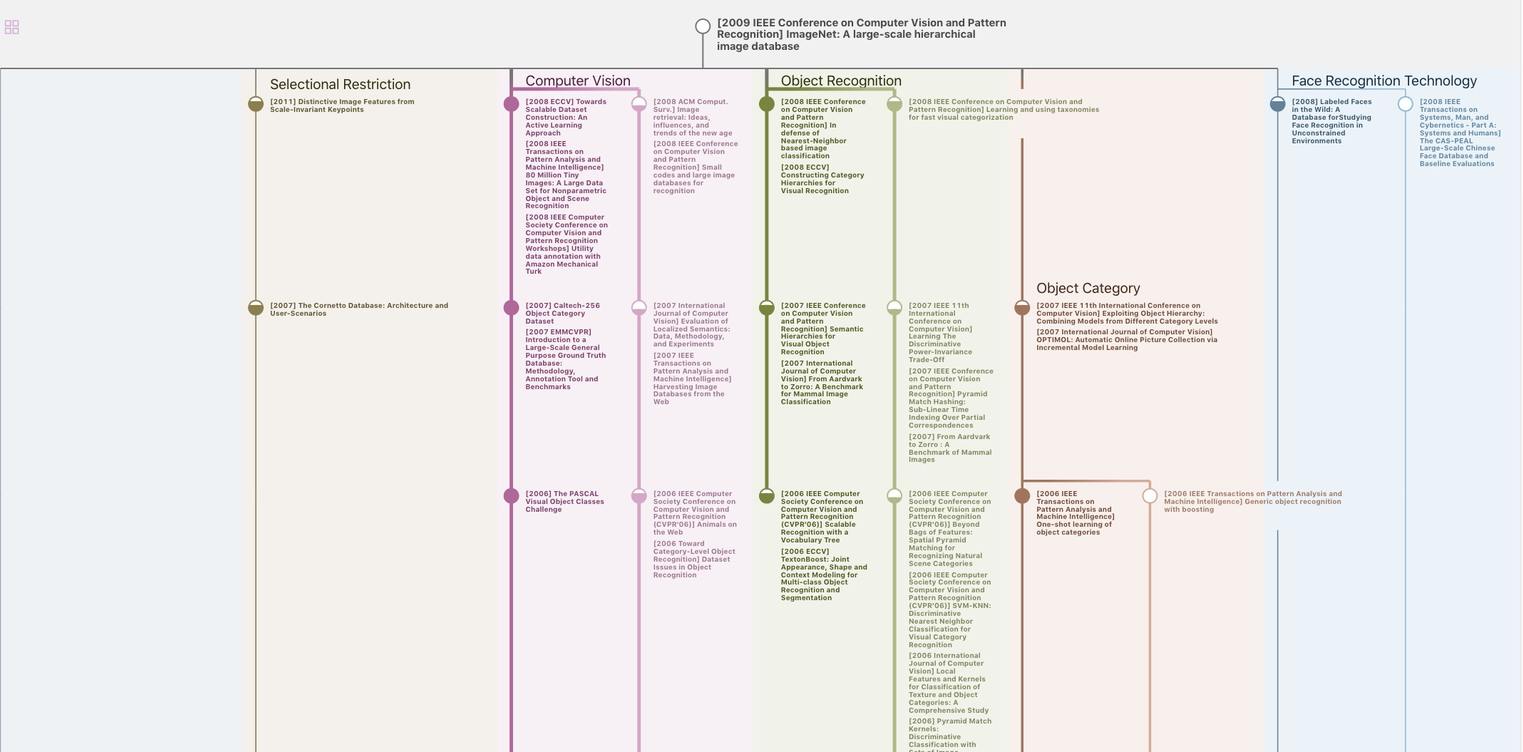
生成溯源树,研究论文发展脉络
Chat Paper
正在生成论文摘要