Seeing stars: {E}xploiting class relationships for sentiment categorization with respect to rating scales
msra(2005)
摘要
We address the rating-inference problem, wherein rather than simply decide
whether a review is "thumbs up" or "thumbs down", as in previous sentiment
analysis work, one must determine an author's evaluation with respect to a
multi-point scale (e.g., one to five "stars"). This task represents an
interesting twist on standard multi-class text categorization because there are
several different degrees of similarity between class labels; for example,
"three stars" is intuitively closer to "four stars" than to "one star". We
first evaluate human performance at the task. Then, we apply a meta-algorithm,
based on a metric labeling formulation of the problem, that alters a given
n-ary classifier's output in an explicit attempt to ensure that similar items
receive similar labels. We show that the meta-algorithm can provide significant
improvements over both multi-class and regression versions of SVMs when we
employ a novel similarity measure appropriate to the problem.
更多查看译文
关键词
rating-inference problem,similar item,different degree,interesting twist,class label,novel similarity measure,rating scale,explicit attempt,human performance,sentiment categorization,standard multi-class text categorization,similar label,class relationship
AI 理解论文
溯源树
样例
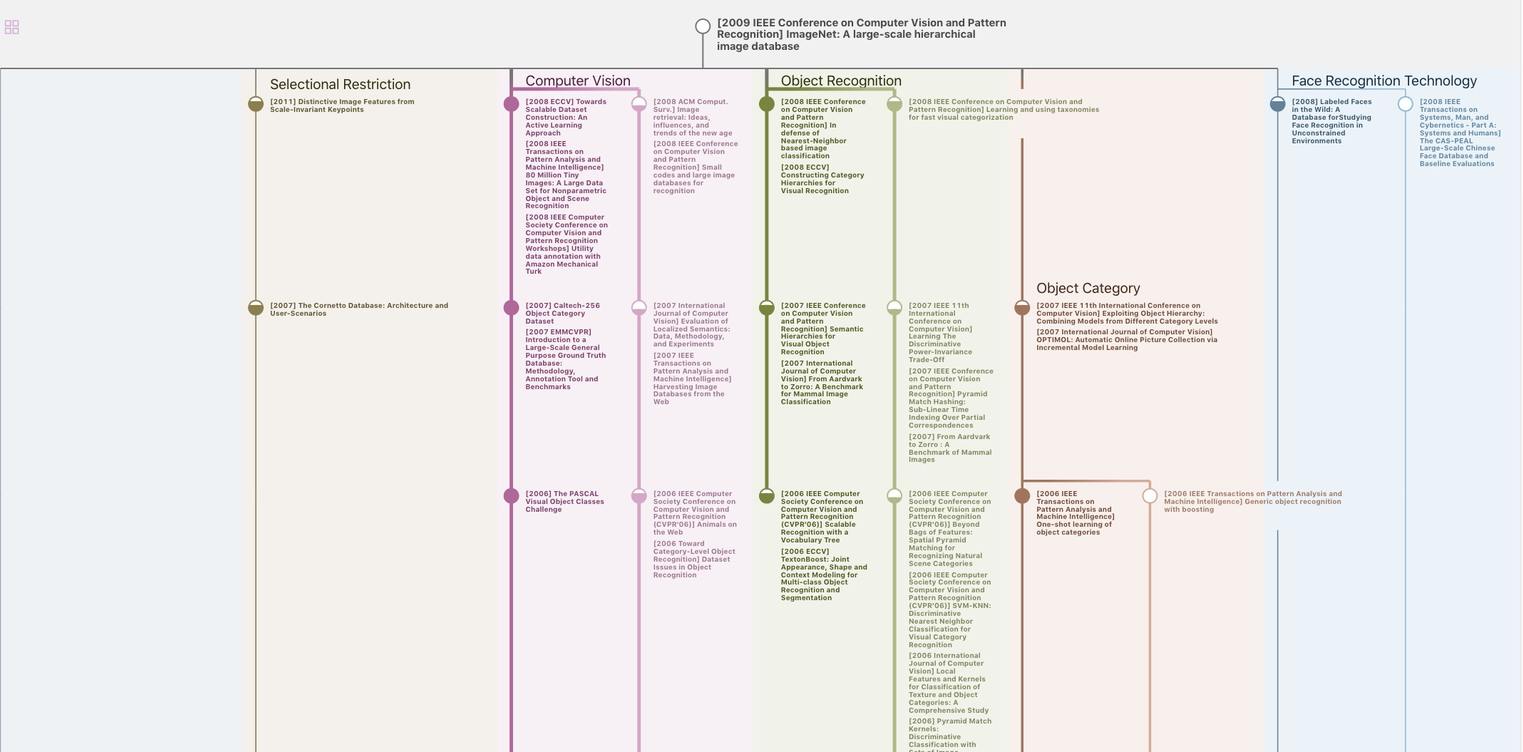
生成溯源树,研究论文发展脉络
Chat Paper
正在生成论文摘要