Learning individual human activities from short binary shape sequences
msra(2011)
摘要
We present a new algorithm capable of classifying individual human activities from very short sequences. Our method is based on a multi-stage architecture which rst produces binary shape sequences with background subtraction. Low-level shape features are extracted from these short sequences and fed to a probabilistic multi-layer model (a conditional deep belief network), which learns the evolution of the low-level features through time through interactions with binary latent variables. No appearance model is needed. Actions are classied using an SVM trained on the posterior probabilities of the latent features extracted by the motion model. We tested the algo- rithm on two dierent databases. We achieve a 100% classication rate (per video using a voting strategy) on the well known Weizmann database, and a near perfect 99.9% classication rate per short sequence.
更多查看译文
关键词
video processing,conditional boltzmann machines,activity recognition
AI 理解论文
溯源树
样例
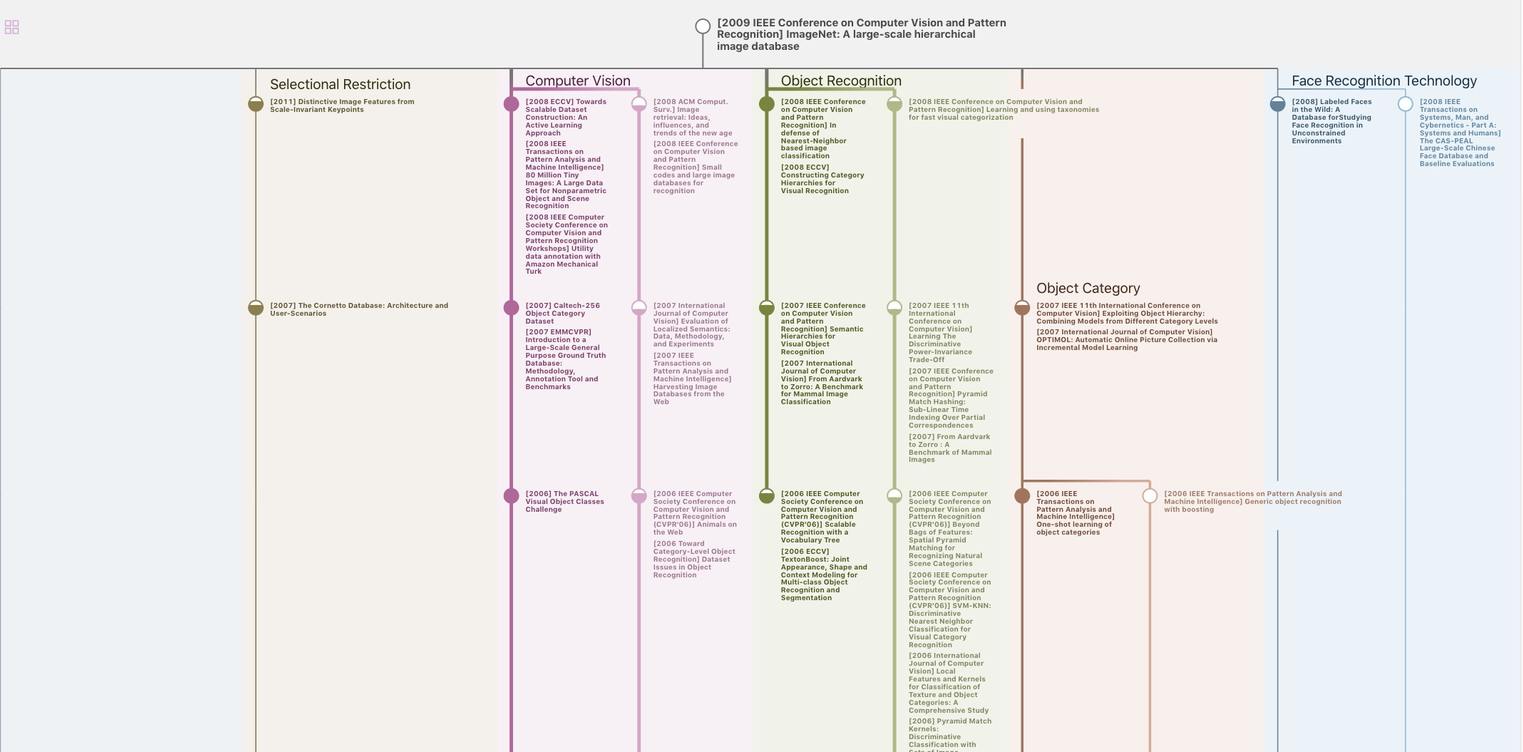
生成溯源树,研究论文发展脉络
Chat Paper
正在生成论文摘要