Kernel methods in system identification, machine learning and function estimation: A survey.
Automatica(2014)
摘要
Most of the currently used techniques for linear system identification are based on classical estimation paradigms coming from mathematical statistics. In particular, maximum likelihood and prediction error methods represent the mainstream approaches to identification of linear dynamic systems, with a long history of theoretical and algorithmic contributions. Parallel to this, in the machine learning community alternative techniques have been developed. Until recently, there has been little contact between these two worlds. The first aim of this survey is to make accessible to the control community the key mathematical tools and concepts as well as the computational aspects underpinning these learning techniques. In particular, we focus on kernel-based regularization and its connections with reproducing kernel Hilbert spaces and Bayesian estimation of Gaussian processes. The second aim is to demonstrate that learning techniques tailored to the specific features of dynamic systems may outperform conventional parametric approaches for identification of stable linear systems.
更多查看译文
关键词
Linear system identification,Prediction error methods,Model complexity selection,Bias-variance trade-off,Kernel-based regularization,Inverse problems,Reproducing kernel Hilbert spaces,Gaussian processes
AI 理解论文
溯源树
样例
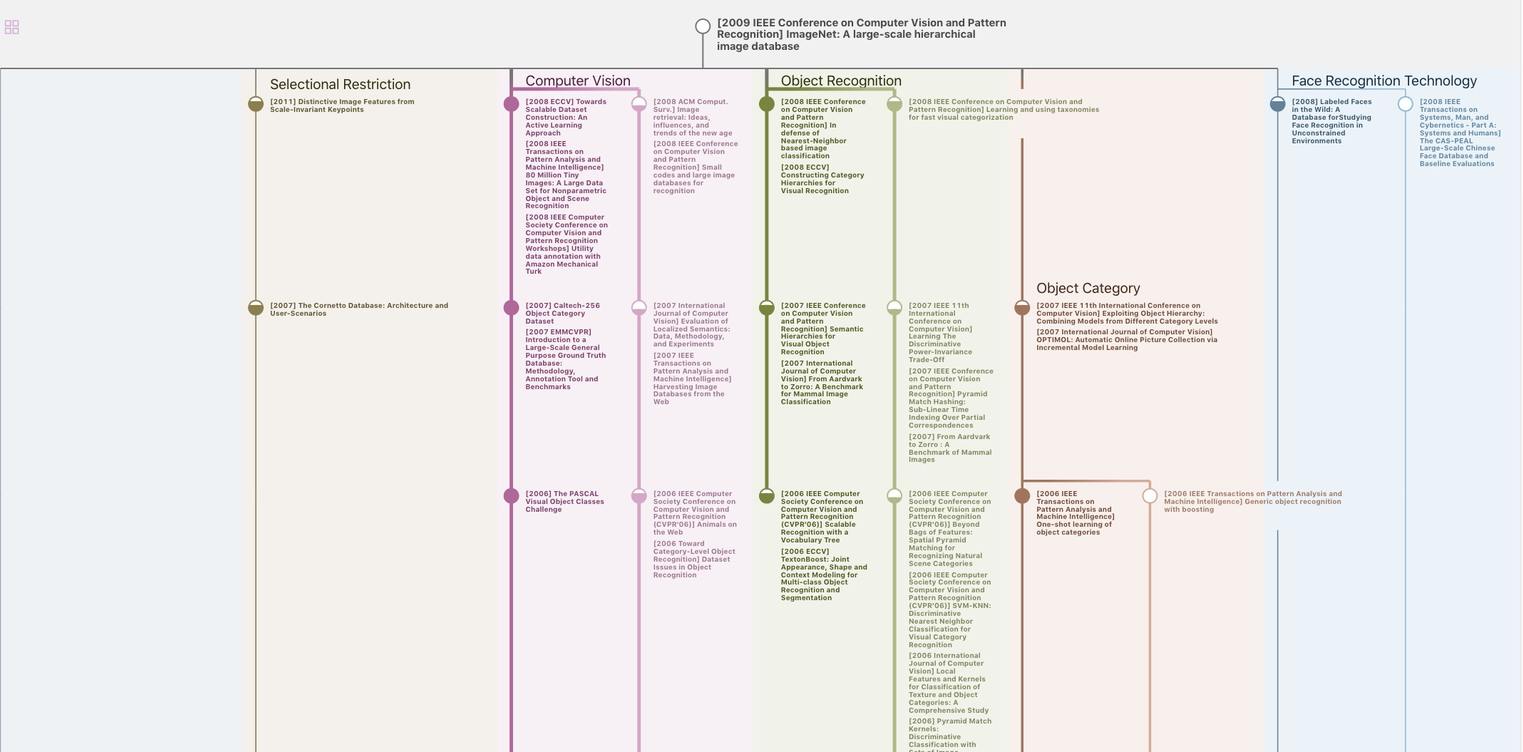
生成溯源树,研究论文发展脉络
Chat Paper
正在生成论文摘要