Efficient Topic-Based Unsupervised Name Disambiguation
JCDL(2007)
摘要
Name ambiguity is a special case of identity uncertainty where one person can be referenced by multiple name variations in different situations or even share the same name with other people. In this paper, we focus on the problem of disambiguating person names within web pages and scientific documents. We present an efficient and effective two-stage approach to disambiguate names. lit the first stage, two novel topic-based models are proposed by extending two hierarchical Bayesian text models, namely Probabilistic Latent Semantic Analysis (PLSA) and Latent Dirichlet Allocation (LDA). Our models explicitly introduce a new variable for persons and learn the distribution of topics with regard to persons and words. After learning an initial model, the topic distributions are treated as feature sets and names are disambiguated by leveraging a hierarchical agglomerative clustering method. Experiments on web data and scientific documents from CiteSeer indicate that our approach consistently outperforms other unsupervised learning methods such as spectral clustering and DBSCAN clustering and could be extended to other research fields. We empirically addressed the issue of scalability by disambiguating authors in over 750,000 papers from the entire CiteSeer dataset.
更多查看译文
关键词
Unsupervised Machine Learning,Bayesian Models,Name
AI 理解论文
溯源树
样例
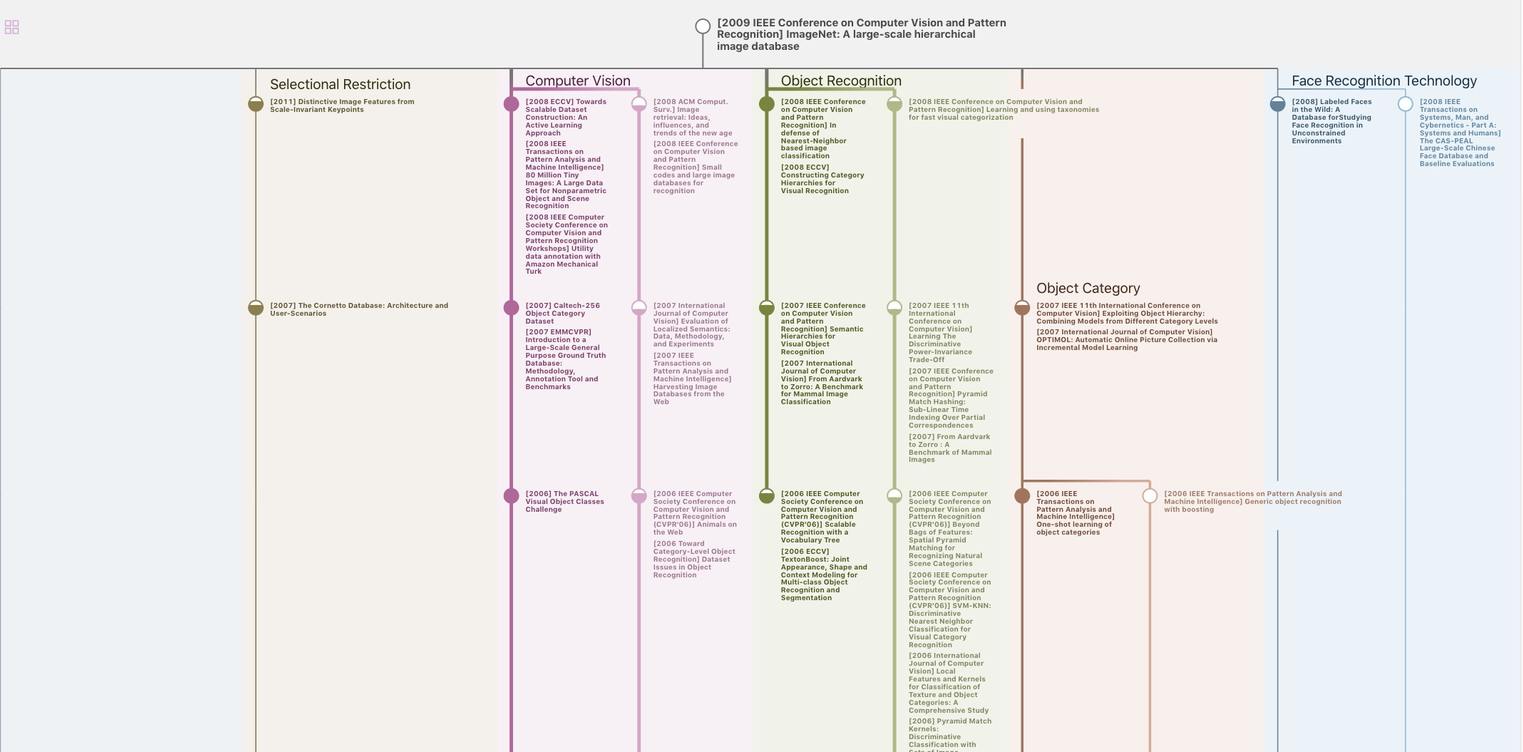
生成溯源树,研究论文发展脉络
Chat Paper
正在生成论文摘要