Cascaded parallel crowd counting network with multi-resolution collaborative representation
Applied Intelligence(2022)
摘要
Accurately estimating the size and density distribution of a crowd from images is of great importance to public safety and crowd management during the COVID-19 pandemic, but it is very challenging as it is affected by many complex factors, including perspective distortion and background noise information. In this paper, we propose a novel multi-resolution collaborative representation framework called the cascaded parallel network (CP-Net), consisting of three parallel scale-specific branches connected in a cascading mode. In the framework, the three cascaded multi-resolution branches efficiently capture multi-scale features through their specific receptive fields. Additionally, multi-level feature fusion and information filtering are performed continuously on each branch to resist noise interference and perspective distortion. Moreover, we design an information exchange module across independent branches to refine the features extracted by each specific branch and deal with perspective distortion by using complementary information of multiple resolutions. To further improve the robustness of the network to scale variance and generate high-quality density maps, we construct a multi-receptive field fusion module to aggregate multi-scale features more comprehensively. The performance of our proposed CP-Net is verified on the challenging counting datasets (UCF_CC_50, UCF-QNRF, Shanghai Tech A&B, and WorldExpo’10), and the experimental results demonstrate the superiority of the proposed method.
更多查看译文
关键词
Crowd counting, Density map estimation, Cascaded multi-resolution CNN, Multi-scale fusion
AI 理解论文
溯源树
样例
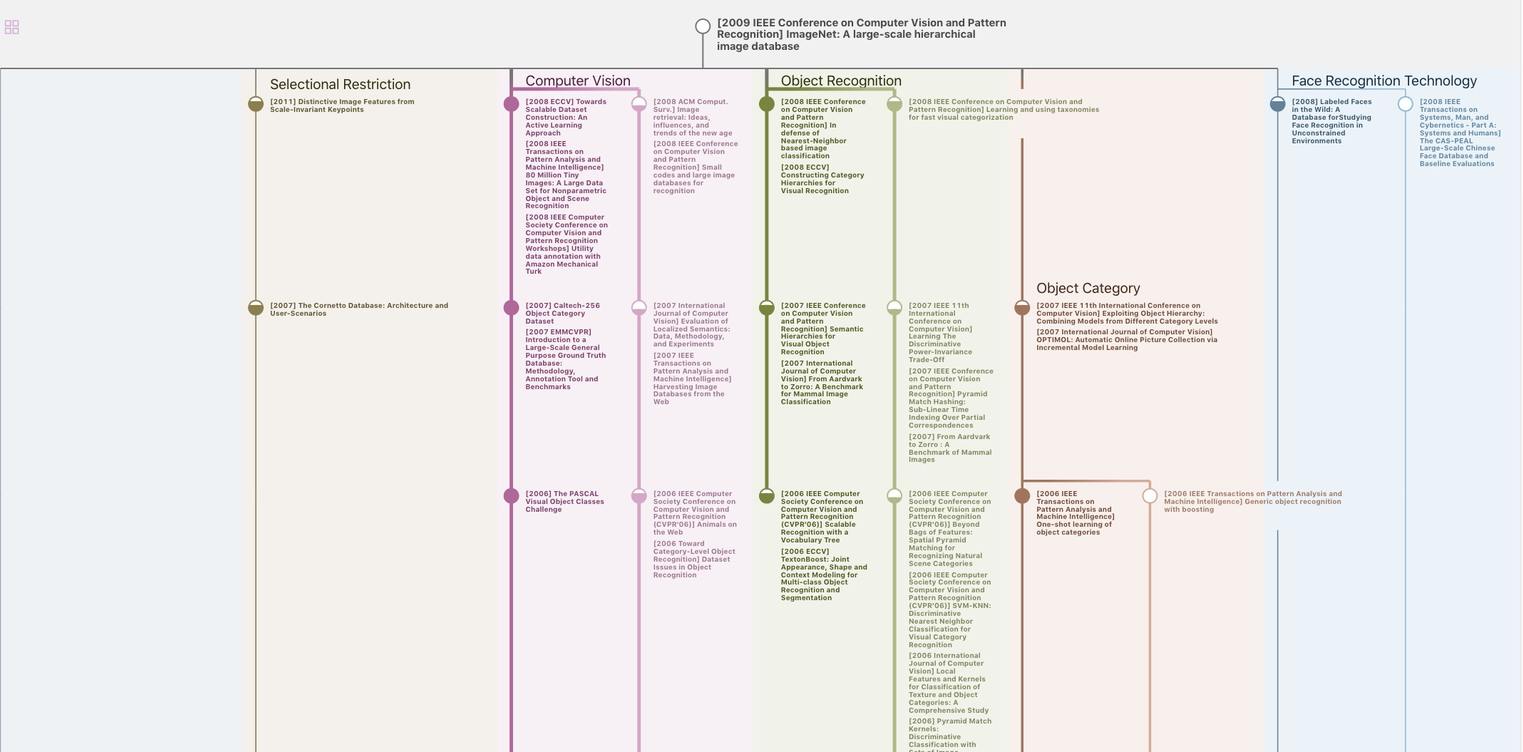
生成溯源树,研究论文发展脉络
Chat Paper
正在生成论文摘要