Binary Constrained Deep Hashing Network for Image Retrieval without Manual Annotation
2019 IEEE Winter Conference on Applications of Computer Vision (WACV)(2018)
摘要
Learning compact binary codes for image retrieval task using deep neural networks has attracted increasing attention recently. However, training deep hashing networks for the task is challenging due to the binary constraints on the hash codes, the similarity preserving property, and the requirement for a vast amount of labelled images. To the best of our knowledge, none of the existing methods has tackled all of these challenges completely in a unified framework. In this work, we propose a novel end-to-end deep learning approach for the task, in which the network is trained to produce binary codes directly from image pixels without the need of manual annotation. In particular, to deal with the non-smoothness of binary constraints, we propose a novel pairwise constrained loss function, which simultaneously encodes the distances between pairs of hash codes, and the binary quantization error. In order to train the network with the proposed loss function, we propose an efficient parameter learning algorithm. In addition, to provide similar / dissimilar training images to train the network, we exploit 3D models reconstructed from unlabelled images for automatic generation of enormous training image pairs. The extensive experiments on image retrieval benchmark datasets demonstrate the improvements of the proposed method over the state-of-the-art compact representation methods on the image retrieval problem.
更多查看译文
关键词
Image retrieval,Binary codes,Training,Three-dimensional displays,Task analysis,Training data,Feature extraction
AI 理解论文
溯源树
样例
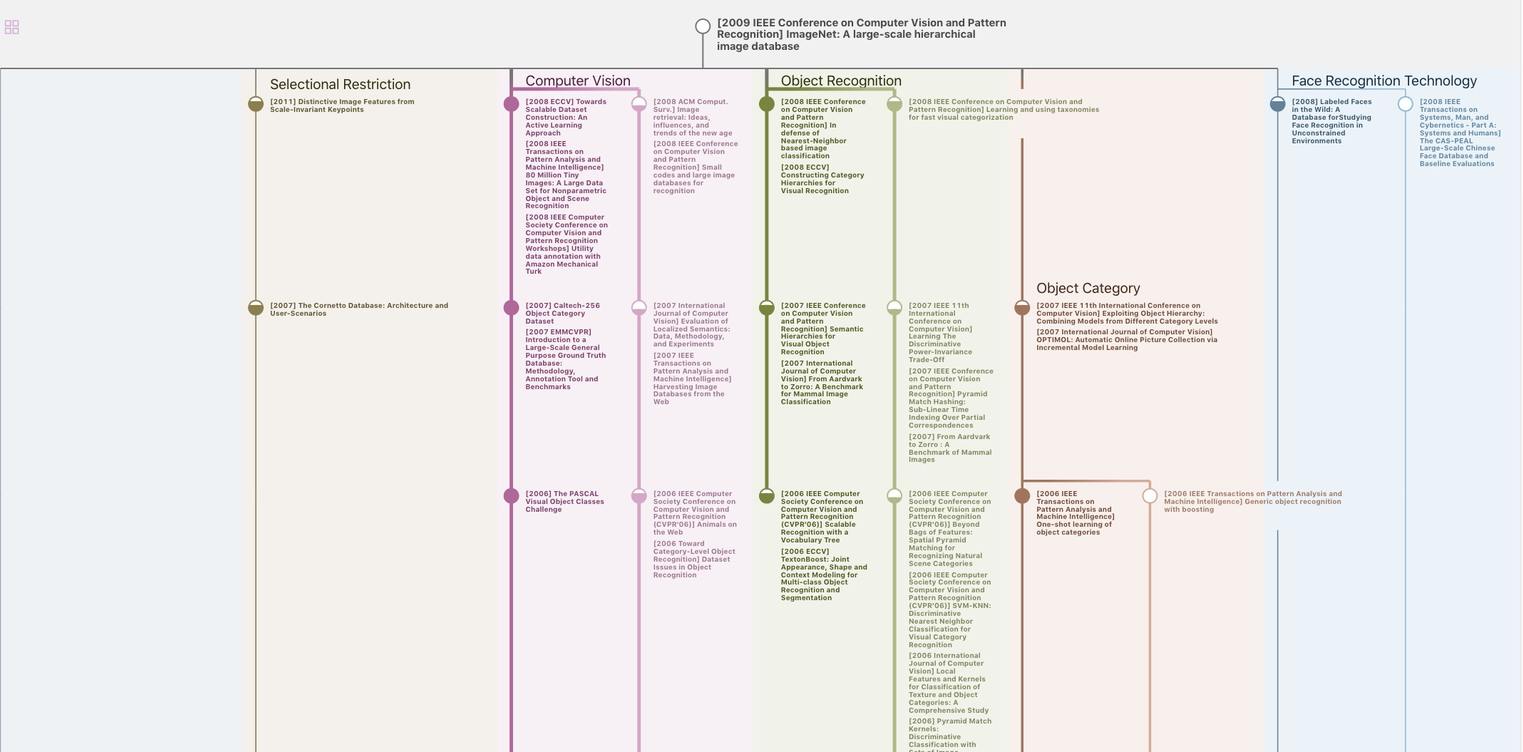
生成溯源树,研究论文发展脉络
Chat Paper
正在生成论文摘要