Sentiment summarization: evaluating and learning user preferences
EACL(2009)
摘要
We present the results of a large-scale, end-to-end human evaluation of various sentiment summarization models. The evaluation shows that users have a strong preference for summarizers that model sentiment over non-sentiment baselines, but have no broad overall preference between any of the sentiment-based models. However, an analysis of the human judgments suggests that there are identifiable situations where one summarizer is generally preferred over the others. We exploit this fact to build a new summarizer by training a ranking SVM model over the set of human preference judgments that were collected during the evaluation, which results in a 30% relative reduction in error over the previous best summarizer.
更多查看译文
关键词
strong preference,user preference,previous best summarizer,human preference judgment,model sentiment,new summarizer,sentiment-based model,end-to-end human evaluation,broad overall preference,ranking svm model,sentiment summarization,human judgment
AI 理解论文
溯源树
样例
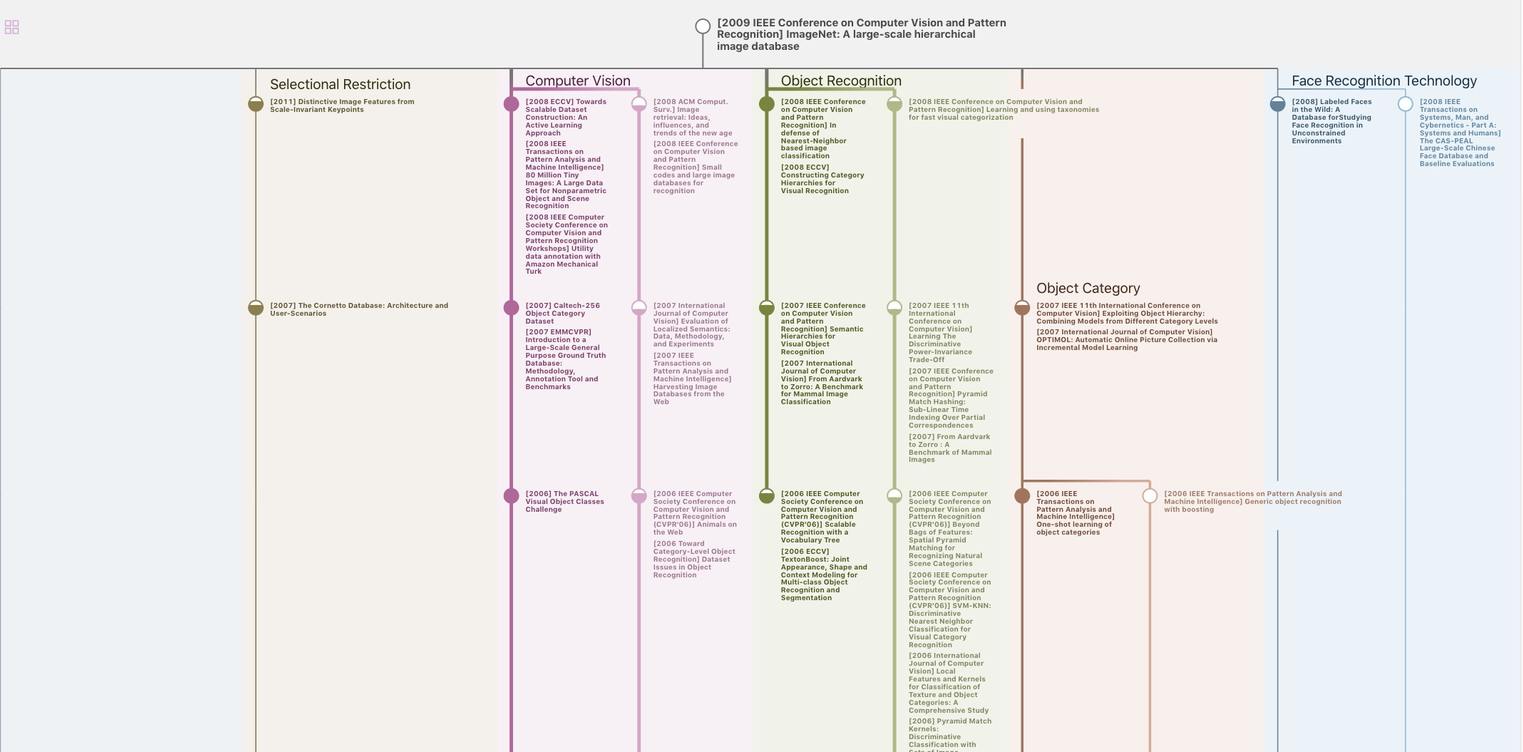
生成溯源树,研究论文发展脉络
Chat Paper
正在生成论文摘要