Robust modelling of solubility in supercritical carbon dioxide using Bayesian methods
Journal of Molecular Graphics and Modelling(2010)
摘要
Two sparse Bayesian methods were used to derive predictive models of solubility of organic dyes and polycyclic aromatic compounds in supercritical carbon dioxide (scCO2), over a wide range of temperatures (285.9–423.2K) and pressures (60–1400bar): a multiple linear regression employing an expectation maximization algorithm and a sparse prior (MLREM) method and a non-linear Bayesian Regularized Artificial Neural Network with a Laplacian Prior (BRANNLP). A randomly selected test set was used to estimate the predictive ability of the models. The MLREM method resulted in a model of similar predictivity to the less sparse MLR method, while the non-linear BRANNLP method created models of substantially better predictivity than either the MLREM or MLR based models. The BRANNLP method simultaneously generated context-relevant subsets of descriptors and a robust, non-linear quantitative structure–property relationship (QSPR) model for the compound solubility in scCO2. The differences between linear and non-linear descriptor selection methods are discussed.
更多查看译文
关键词
Bayesian methods,Structure–property relationships,Dyes,Solubility,Supercritical carbon dioxide
AI 理解论文
溯源树
样例
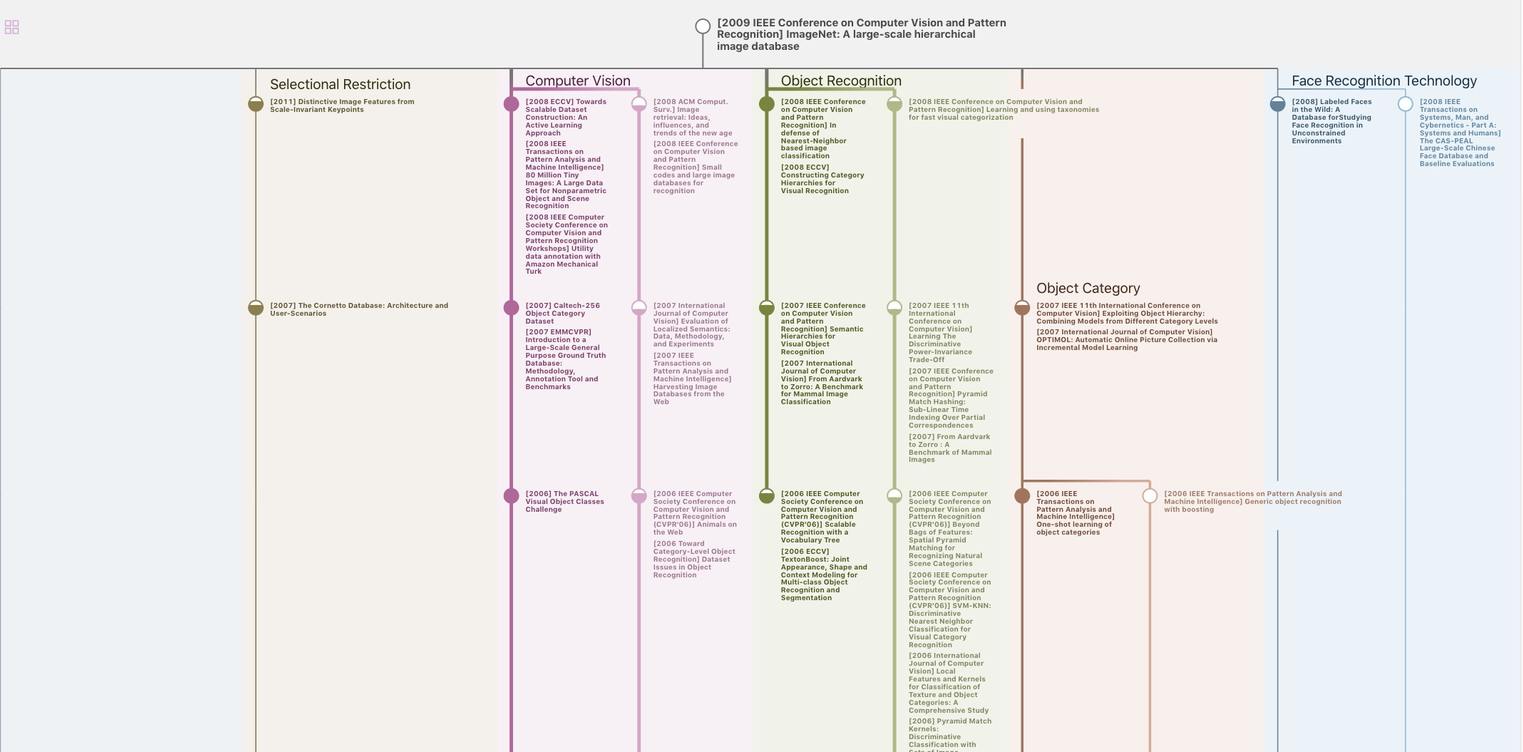
生成溯源树,研究论文发展脉络
Chat Paper
正在生成论文摘要