Unsupervised Texture Segmentation And Labeling Using Biologically Inspired Features
2008 IEEE 10TH WORKSHOP ON MULTIMEDIA SIGNAL PROCESSING, VOLS 1 AND 2(2008)
摘要
Due to the semantic gap, describing high-level semantic concepts with low-level visual features is a very challenging task. The classification of textures in scene images is intricate because of the high variation of the data. Therefore, the application of appropriate features is of utter importance. This paper presents biologically inspired features for texture segmentation and an unsupervised method to link those texture features with semantic concepts. The calculation of the features is inspired by the human visual system and corresponds to cell outputs in the first stage of the visual cortex. Analogously to the processing principles of the cortex, self-organizing maps are employed for classification. The performance of the texture segmentation and labeling is evaluated on textures from the Brodatz album and on a real-life scenery image dataset. For both methods, a high percentage of pixels is correctly classified.
更多查看译文
关键词
image segmentation,image classification,self organizing maps,image texture,labeling,visualization,feature extraction,pixel,semantic gap,human visual system
AI 理解论文
溯源树
样例
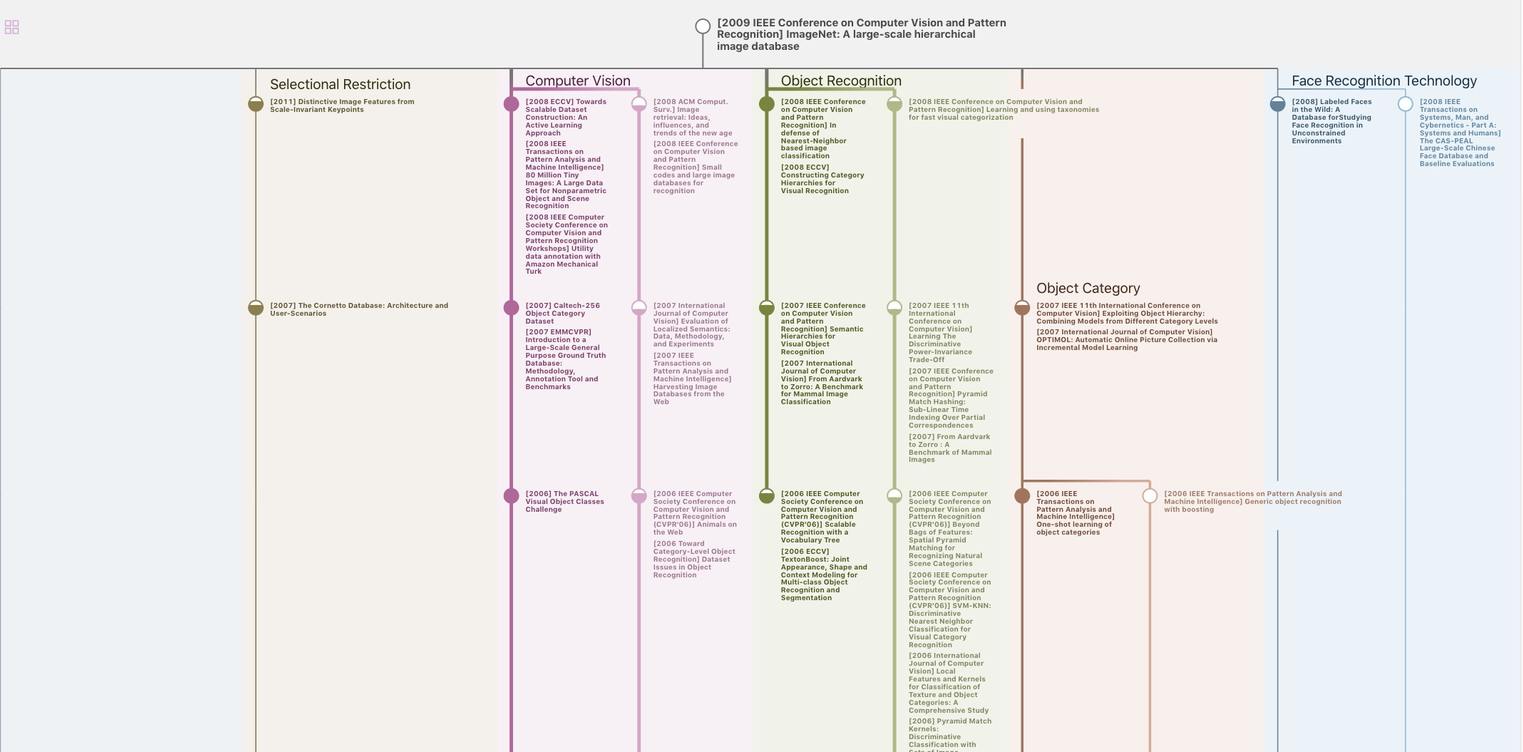
生成溯源树,研究论文发展脉络
Chat Paper
正在生成论文摘要