Nonparametric segmentation and classification of small size irregularly shaped stem cell nuclei using adjustable windowing
ISBI(2010)
摘要
We present nonparametric methods for segmenting and classifying stem cell nuclei so as to enable the automatic monitoring of stem cell growth and development. The approach is based on combining level set methods, multiresolution wavelet analysis, and non-parametric estimation of the density functions of the wavelet coefficients from the decomposition. Additionally, to deal with small size textures where the largest inscribed rectangular window may not contain a sufficient number of pixels for multiresolution analysis, we propose an adjustable windowing method that enables the multiresolution analysis of elongated and irregularly shaped nuclei. We illustrate cases where the adjustable windowing approach combined with non-parametric density models yields better classification for cases where parametric density modeling of wavelet coefficients may not applicable.
更多查看译文
关键词
cellular biophysics,image classification,image segmentation,image texture,medical image processing,multiprogramming,wavelet transforms,adjustable windowing,automatic monitoring,density function,irregularly shaped stem cell nuclei,level set method,multiresolution wavelet analysis,nonparametric classification,nonparametric estimation,nonparametric segmentation,small size stem cell nuclei,stem cell development,stem cell growth,wavelet coefficient,adaptive,adjustable,divergence,non-parametric,stem cell,texture,wavelet,window
AI 理解论文
溯源树
样例
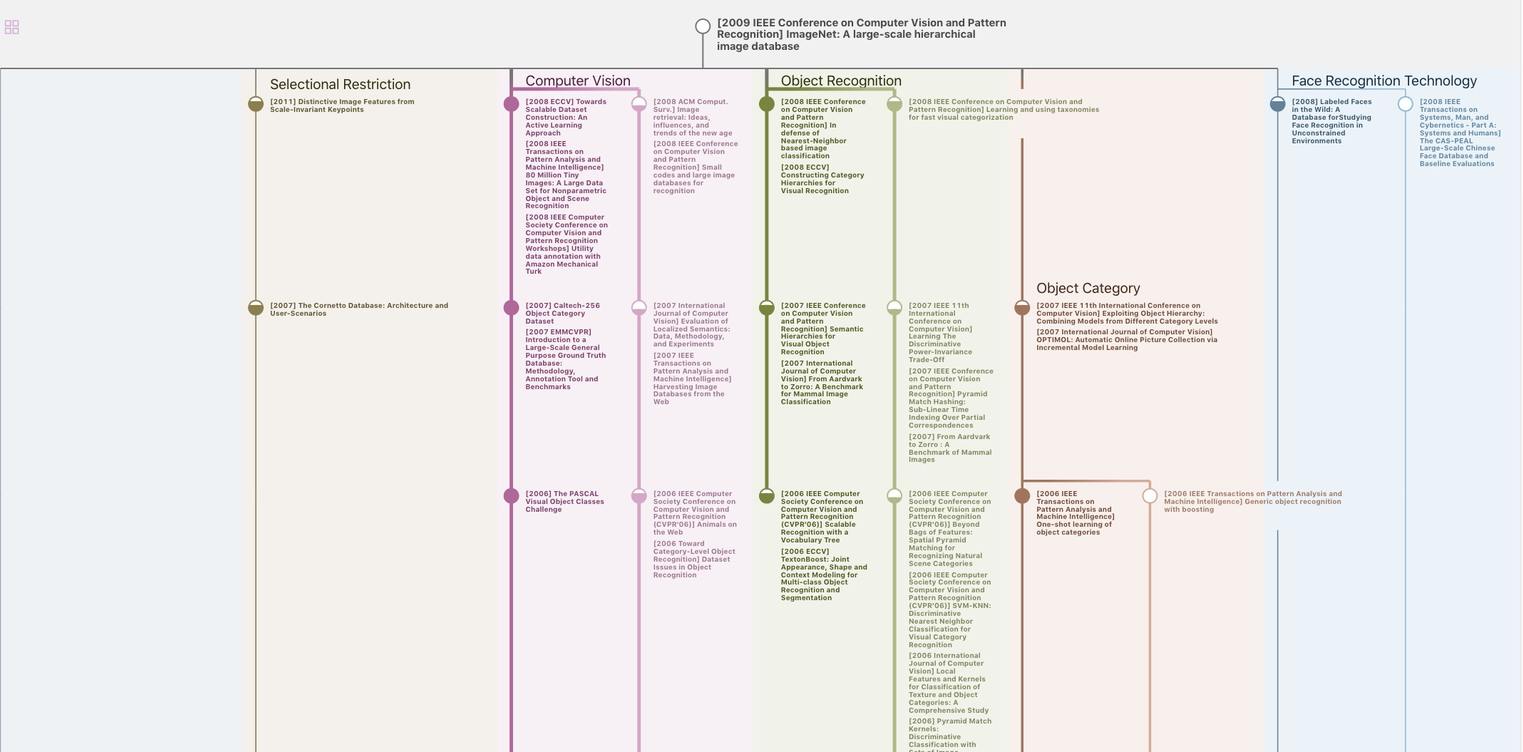
生成溯源树,研究论文发展脉络
Chat Paper
正在生成论文摘要