Tracking Revisited using RGBD Camera: Baseline and Benchmark
CoRR(2012)
摘要
Although there has been significant progress in the past decade,tracking is still a very challenging computer vision task, due to problems such as occlusion and model drift.Recently, the increased popularity of depth sensors e.g. Microsoft Kinect has made it easy to obtain depth data at low cost.This may be a game changer for tracking, since depth information can be used to prevent model drift and handle occlusion.In this paper, we construct a benchmark dataset of 100 RGBD videos with high diversity, including deformable objects, various occlusion conditions and moving cameras. We propose a very simple but strong baseline model for RGBD tracking, and present a quantitative comparison of several state-of-the-art tracking algorithms.Experimental results show that including depth information and reasoning about occlusion significantly improves tracking performance. The datasets, evaluation details, source code for the baseline algorithm, and instructions for submitting new models will be made available online after acceptance.
更多查看译文
AI 理解论文
溯源树
样例
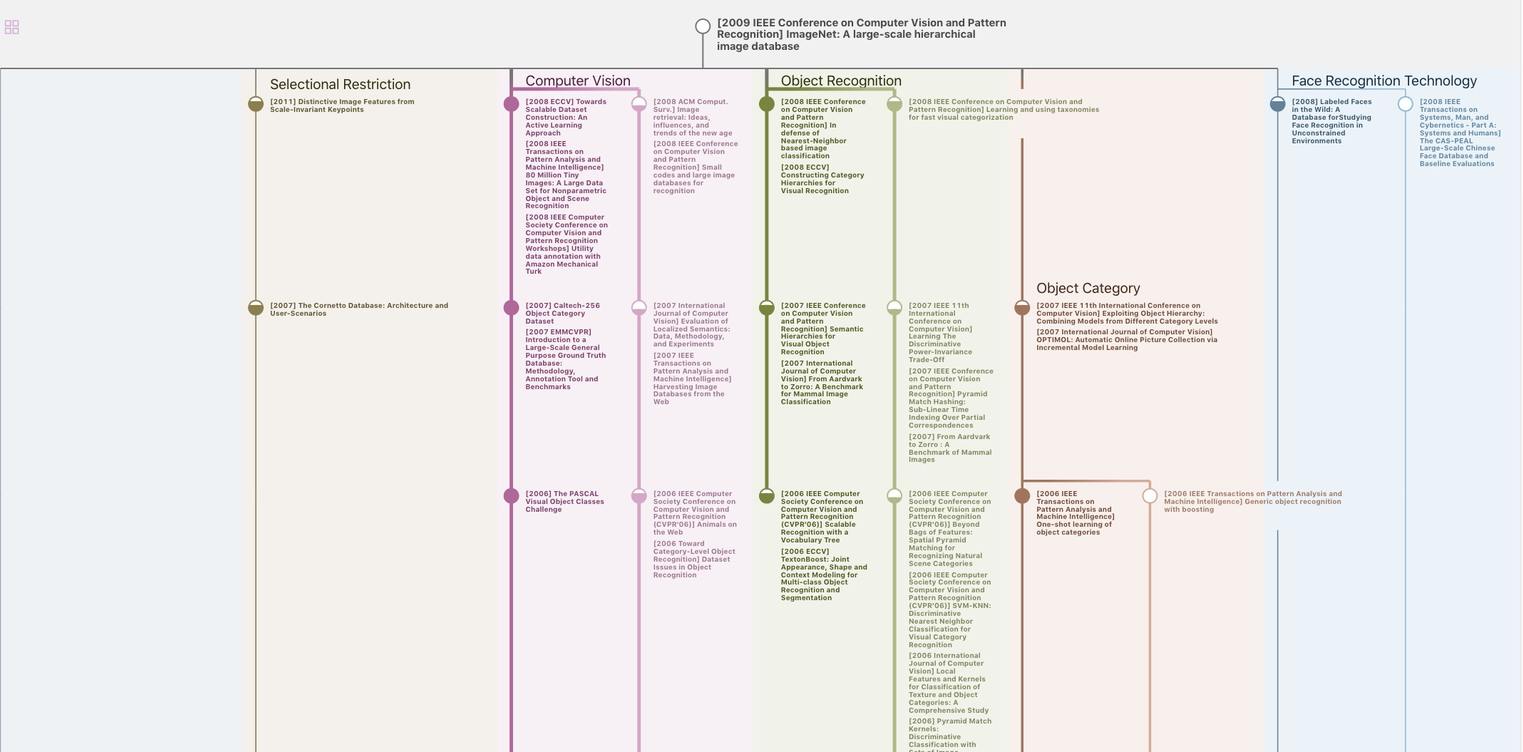
生成溯源树,研究论文发展脉络
Chat Paper
正在生成论文摘要