Hybrid Metaheuristics with Deep Learning Enabled Automated Deception Detection and Classification of Facial Expressions
CMC-COMPUTERS MATERIALS & CONTINUA(2023)
摘要
Automatic deception recognition has received considerable atten-tion from the machine learning community due to recent research on its vast application to social media, interviews, law enforcement, and the mil-itary. Video analysis-based techniques for automated deception detection have received increasing interest. This study develops a new self-adaptive population-based firefly algorithm with a deep learning-enabled automated deception detection (SAPFF-DLADD) model for analyzing facial cues. Ini-tially, the input video is separated into a set of video frames. Then, the SAPFF-DLADD model applies the MobileNet-based feature extractor to produce a useful set of features. The long short-term memory (LSTM) model is exploited for deception detection and classification. In the final stage, the SAPFF technique is applied to optimally alter the hyperparameter values of the LSTM model, showing the novelty of the work. The experimental validation of the SAPFF-DLADD model is tested using the Miami University Deception Detection Database (MU3D), a database comprised of two classes, namely, truth and deception. An extensive comparative analysis reported a better performance of the SAPFF-DLADD model compared to recent approaches, with a higher accuracy of 99%.
更多查看译文
关键词
automated deception detection,facial expressions,deep learning,classification
AI 理解论文
溯源树
样例
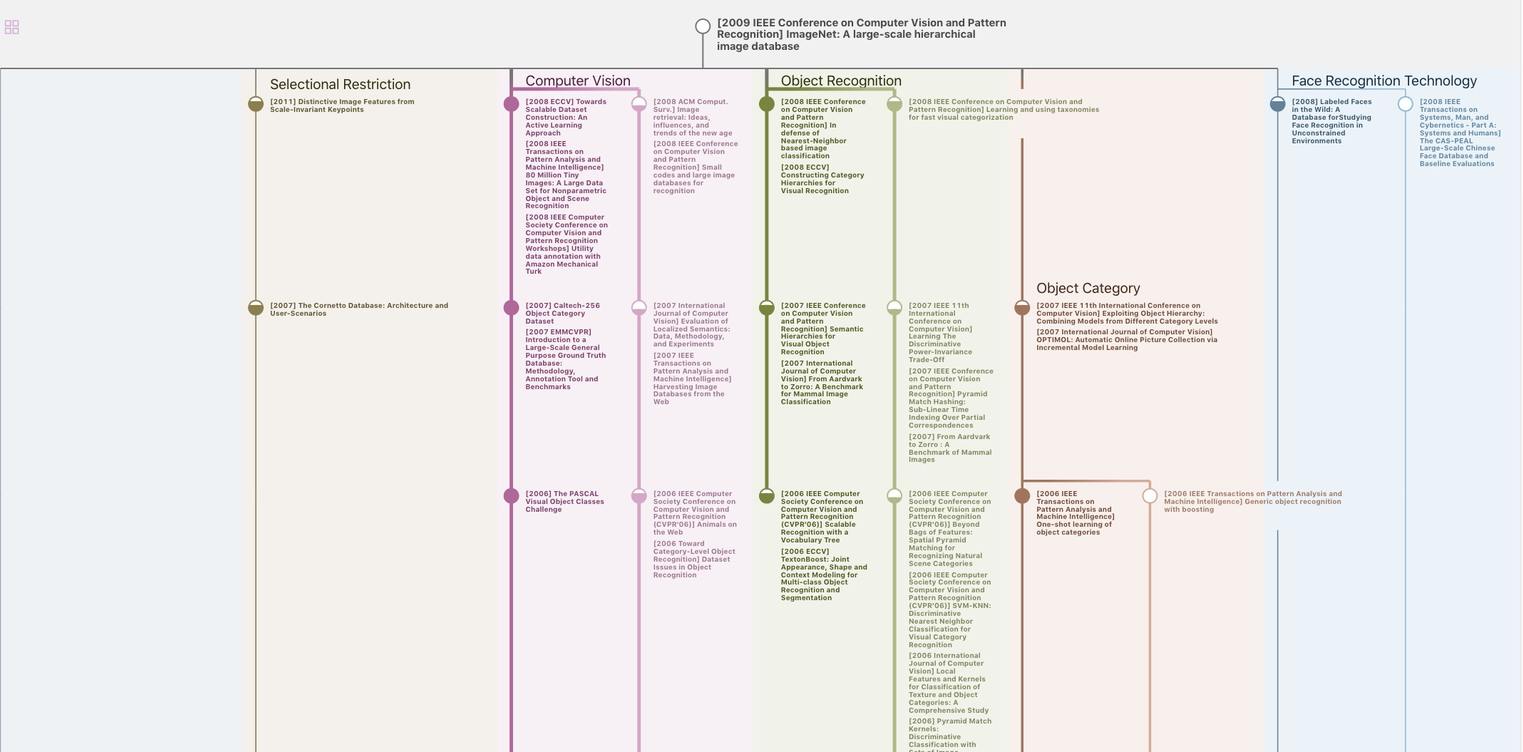
生成溯源树,研究论文发展脉络
Chat Paper
正在生成论文摘要