Biclustering-Based Classification of Clinical Expression Time Series: A Case Study in Patients with Multiple Sclerosis.
5TH INTERNATIONAL CONFERENCE ON PRACTICAL APPLICATIONS OF COMPUTATIONAL BIOLOGY & BIOINFORMATICS (PACBB 2011)(2011)
摘要
In the last years the constant drive towards a more personalized medicine led to an increasing interest in temporal gene expression analyses. In fact, considering a temporal aspect represents a great advantage to better understand disease progression and treatment results at a molecular level. In this work, we analyse multiple gene expression time series in order to classify the response of Multiple Sclerosis patients to the standard treatment with Interferon-beta, to which nearly half of the patients reveal a negative response. In this context, obtaining a highly predictive model of a patient's response would definitely improve his quality of life, avoiding useless and possibly harmful therapies for the non-responder group. We propose new strategies for time series classification based on biclustering. Preliminary results achieved a prediction accuracy of 94.23% and reveal potentialities to be further explored in classification problems involving other (clinical) time series.
更多查看译文
关键词
Prediction Accuracy, Good Responder, Score Matrix, Expression Time Series, Gene Expression Time Series
AI 理解论文
溯源树
样例
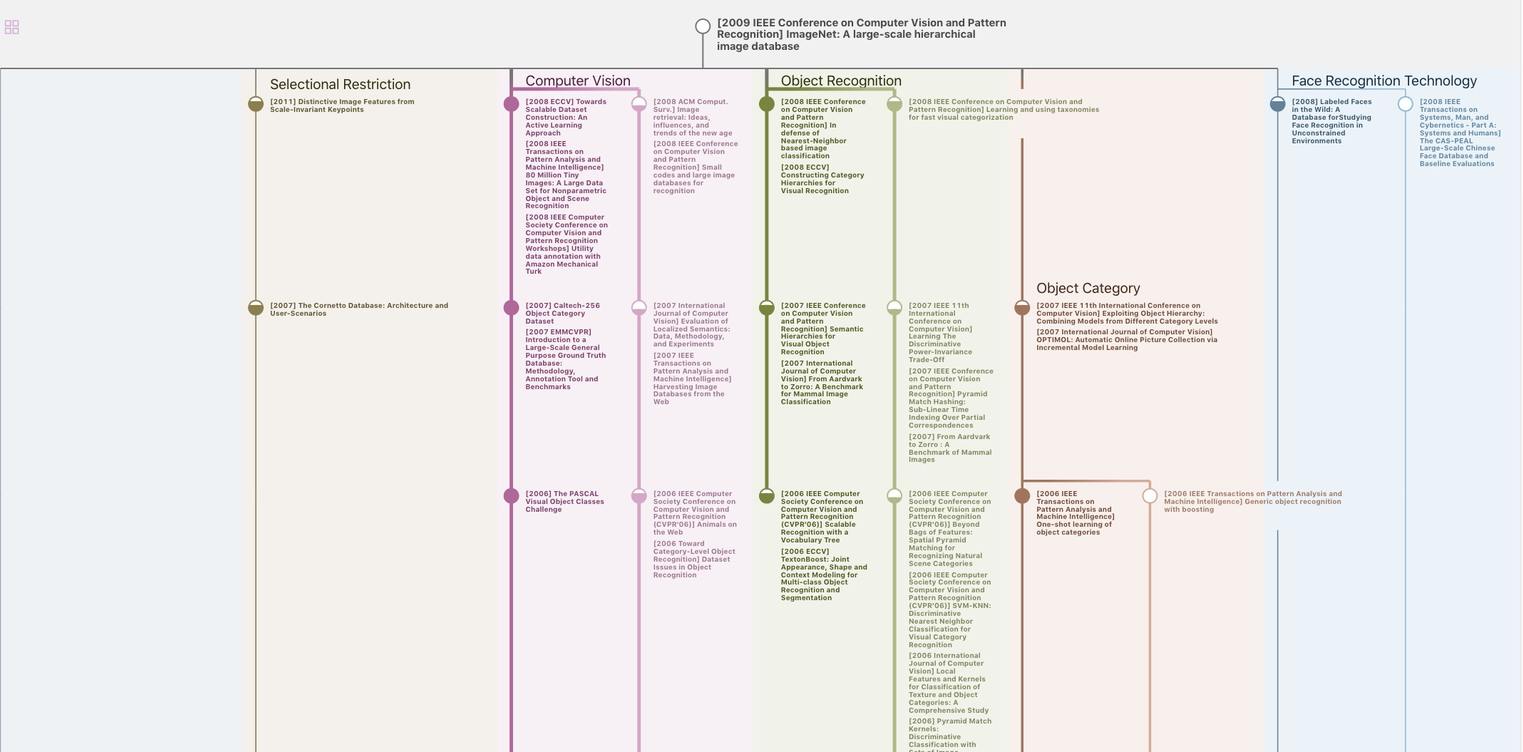
生成溯源树,研究论文发展脉络
Chat Paper
正在生成论文摘要