Complete fully automatic model-based segmentation of normal and pathological lymph nodes in CT data
International Journal of Computer Assisted Radiology and Surgery(2010)
摘要
Purpose Exact and reproducible knowledge regarding the position, size, and type of the lymph nodes is often needed for tumor computer-aided diagnosis, treatment planning, and follow-up. An automatic segmentation method for CT data was developed that can identify and delineate normal as well as pathologically altered lymph nodes to satisfy this requirement. Methods A semi-automatic lymph node segmentation method was developed using a 3D Stable Mass-Spring Model (SMSM), based on parallel simulation of the shape model on CT scan images. The models are started across the whole dataset at all potential lymph node positions but will only adapt to the data where a lymph node is found. The node positions can be determined by an evaluation of the model’s quality of fit. Results Systematically chosen lymph nodes in 5 CT datasets, including enlarged, necrotic, fuzzy-bounded, and deformed lymph nodes, were used to evaluate the segmentation algorithm performance. A test set of 29 lymph nodes taken from 4 typical lymph node regions were included. All lymph nodes were detected automatically, while an additional 31% false-positive ( n = 9) candidates were detected. The average calculation time was 2 min per dataset. The segmentation accuracy was comparable to the inter-observer variance of human experts. Conclusions Clinically relevant lymph nodes were detected within a few minutes and provided sufficient accuracy to demonstrate the feasibility of a new segmentation method. The test data were diverse, and the robust results suggest potential applicability to many kinds of lymph node abnormalities, except for extremely degenerated lymph nodes.
更多查看译文
关键词
Dynamic models,Stable mass-spring models,Lymph node segmentation,Lymph node detection
AI 理解论文
溯源树
样例
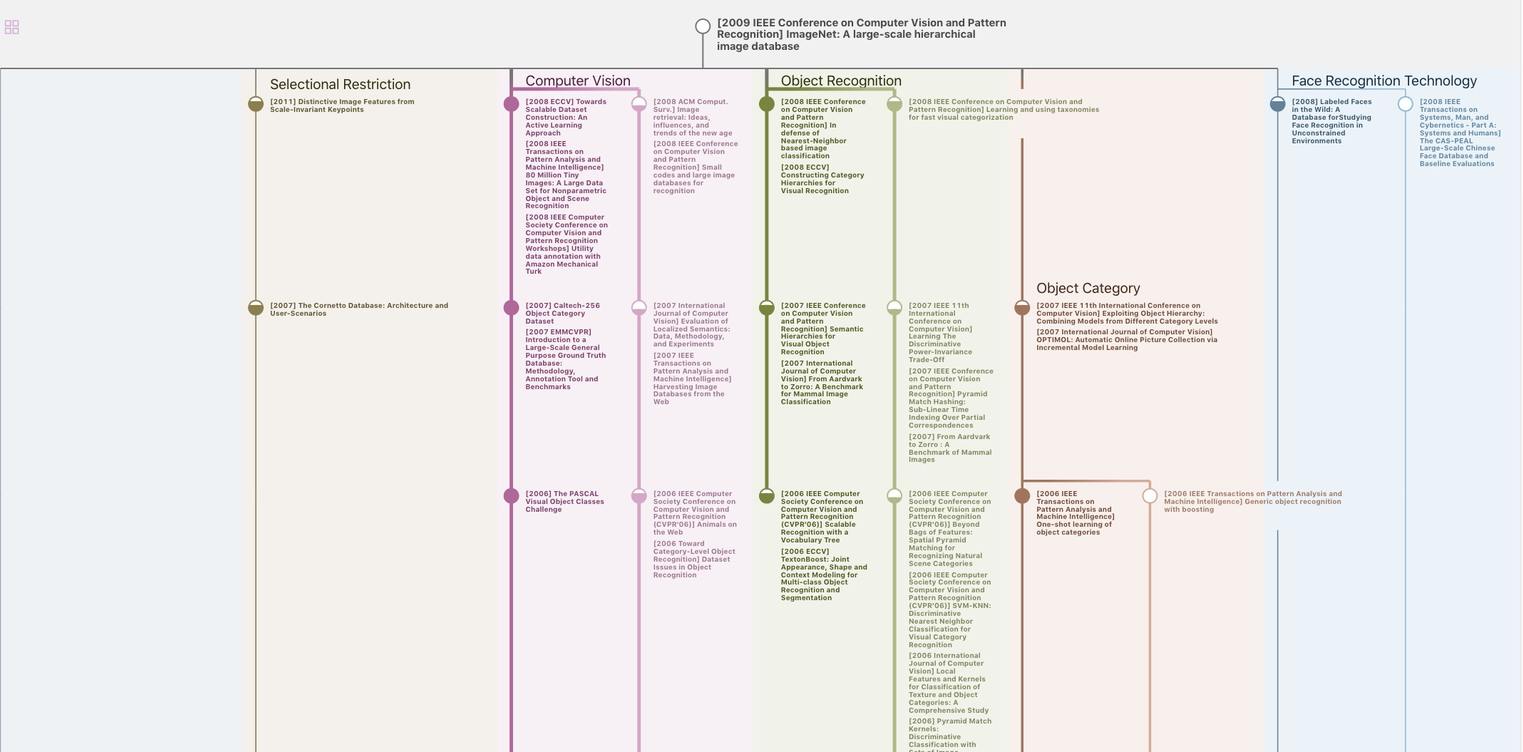
生成溯源树,研究论文发展脉络
Chat Paper
正在生成论文摘要