Automatic correction of annotation boundaries in activity datasets by class separation maximization.
UbiComp '13: The 2013 ACM International Joint Conference on Pervasive and Ubiquitous Computing Zurich Switzerland September, 2013(2013)
摘要
t is challenging to precisely identify the boundary of activities in order to annotate the activity datasets required to train activity recognition systems. This is the case for experts, as well as non-experts who may be recruited for crowd-sourcing paradigms to reduce the annotation effort or speed up the process by distributing the task over multiple annotators. We present a method to automatically adjust annotation boundaries, presuming a correct annotation label, but imprecise boundaries, otherwise known as "label jitter". The approach maximizes the Fukunaga Class-Separability, applied to time series. Evaluations on a standard benchmark dataset showed statistically significant improvements from the initial jittery annotations.
更多查看译文
AI 理解论文
溯源树
样例
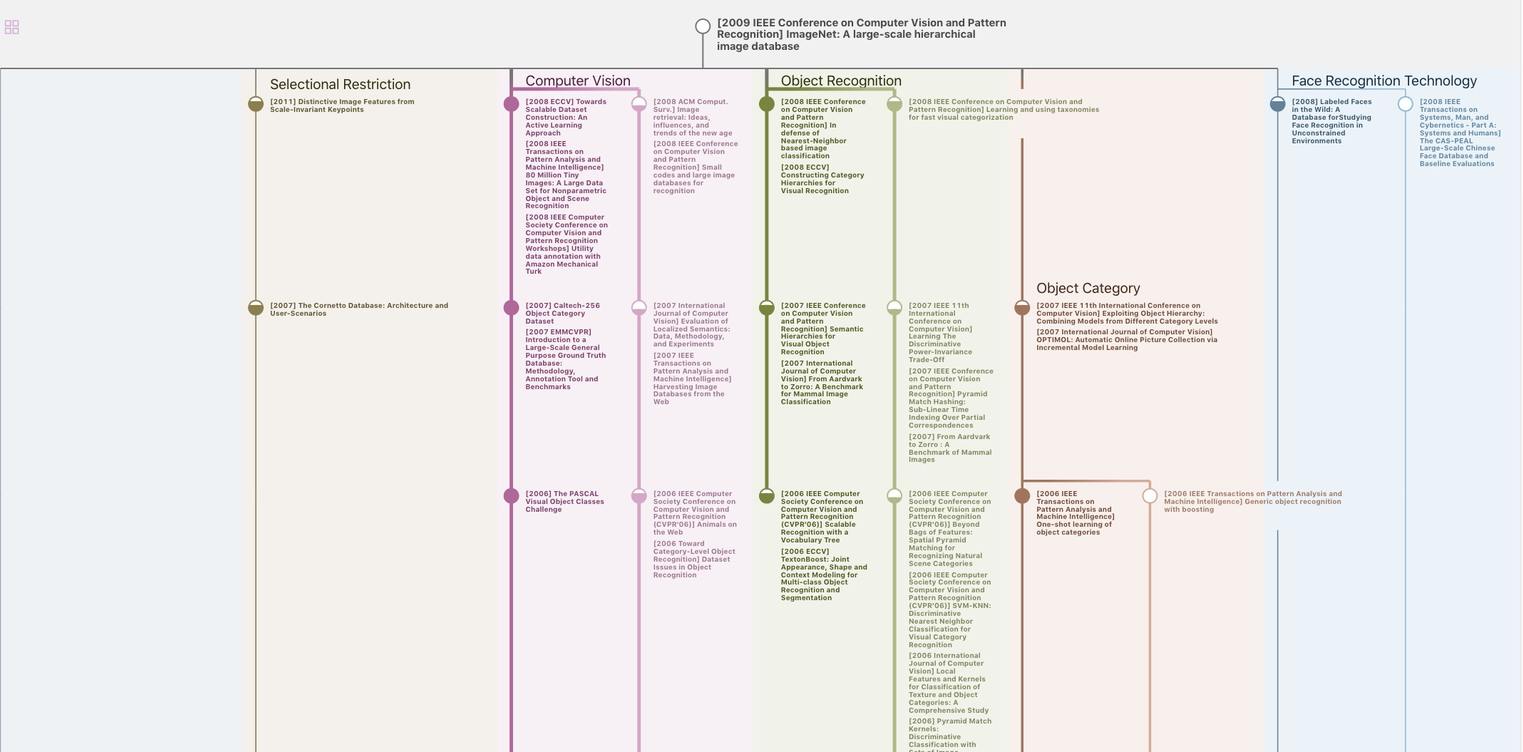
生成溯源树,研究论文发展脉络
Chat Paper
正在生成论文摘要