Adaptive Kalman filtering for histogram-based appearance learning in infrared imagery.
IEEE Transactions on Image Processing(2012)
摘要
Targets of interest in video acquired from imaging infrared sensors often exhibit profound appearance variations due to a variety of factors, including complex target maneuvers, ego-motion of the sensor platform, background clutter, etc., making it difficult to maintain a reliable detection process and track lock over extended time periods. Two key issues in overcoming this problem are how to represent the target and how to learn its appearance online. In this paper, we adopt a recent appearance model that estimates the pixel intensity histograms as well as the distribution of local standard deviations in both the foreground and background regions for robust target representation. Appearance learning is then cast as an adaptive Kalman filtering problem where the process and measurement noise variances are both unknown. We formulate this problem using both covariance matching and, for the first time in a visual tracking application, the recent autocovariance least-squares (ALS) method. Although convergence of the ALS algorithm is guaranteed only for the case of globally wide sense stationary process and measurement noises, we demonstrate for the first time that the technique can often be applied with great effectiveness under the much weaker assumption of piecewise stationarity. The performance advantages of the ALS method relative to the classical covariance matching are illustrated by means of simulated stationary and nonstationary systems. Against real data, our results show that the ALS-based algorithm outperforms the covariance matching as well as the traditional histogram similarity-based methods, achieving sub-pixel tracking accuracy against the well-known AMCOM closure sequences and the recent SENSIAC automatic target recognition dataset.
更多查看译文
关键词
infrared imagery,infrared tracking,nonstationary system,pixel intensity histograms,histogram similarity based method,kalman filters,sensiac automatic target recognition dataset,simulated stationary,infrared imaging,imaging infrared sensors,target maneuvers,adaptive kalman filtering,measurement noise variances,adaptive kalman filter,autocovariance least squares,visual tracking application,piecewise stationarity,appearance model,background clutter,image sensors,sensor platform,subpixel tracking accuracy,covariance matching,reliable detection process,histogram-based appearance learning,appearance learning,robust target representation,histogram-based appearance model
AI 理解论文
溯源树
样例
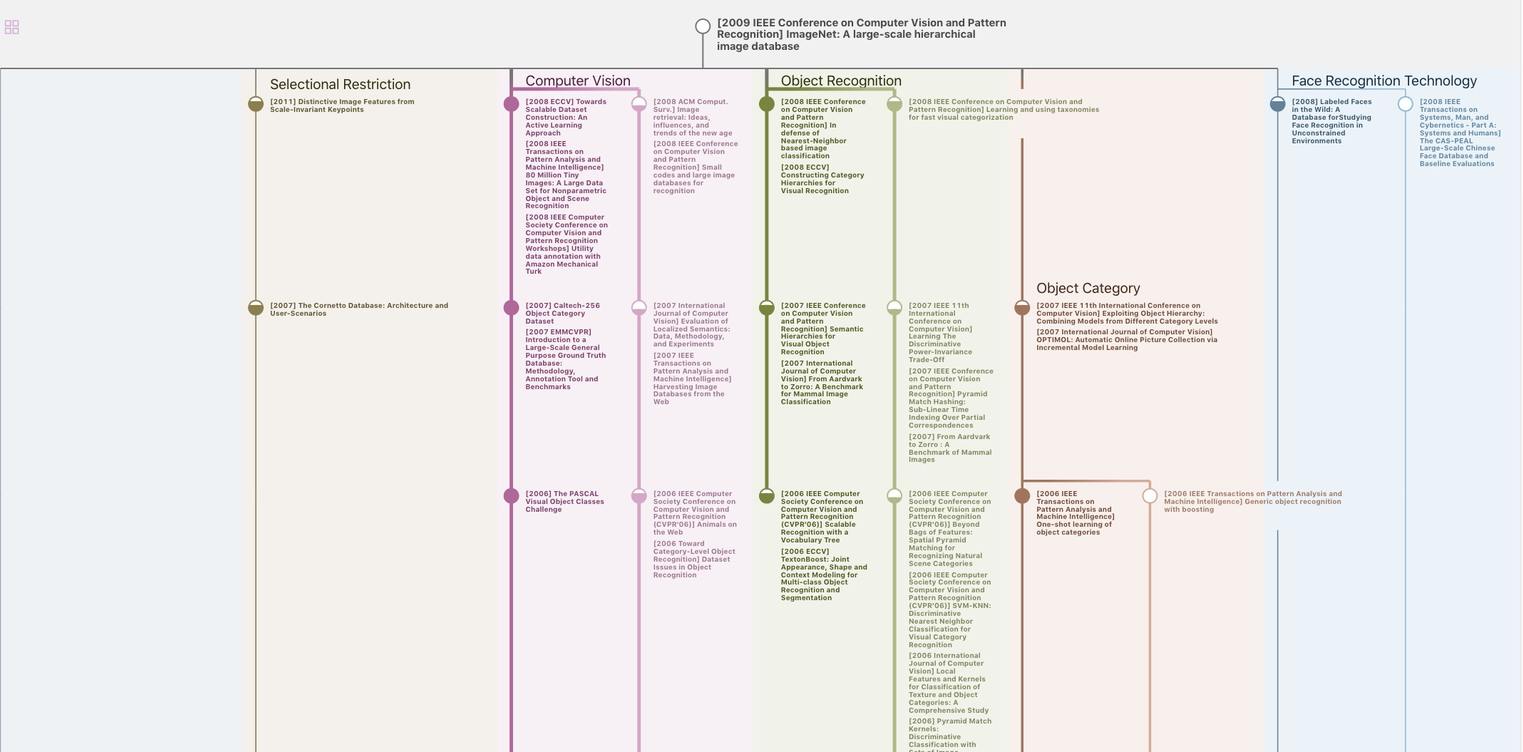
生成溯源树,研究论文发展脉络
Chat Paper
正在生成论文摘要