Similarity preserving analysis based on sparse representation for image feature extraction and classification
ICIP(2013)
摘要
Sparse representation has been a very active research area in recent years. Similarity analysis is an attractive research topic in the field of pattern recognition. In this paper, we take advantage of sparse representation in similarity analysis, and propose a novel unsupervised feature extraction approach, named similarity preserving analysis based on sparse representation (SPASR). SPASR projects samples from a high-dimensional space into a low-dimensional subspace, where the sparse reconstructive similarity relations among samples and the similarities of original samples and sparsely reconstructed samples are preserved. Experiments on the AR face database and COIL-20 object database demonstrate that the proposed SPASR approach outperforms several representative unsupervised subspace learning methods.
更多查看译文
关键词
image representation,pattern recognition,similarity preserving analysis,image feature extraction,subspace learning,subspace learning methods,feature extraction,image classification,high-dimensional space,sparse representation
AI 理解论文
溯源树
样例
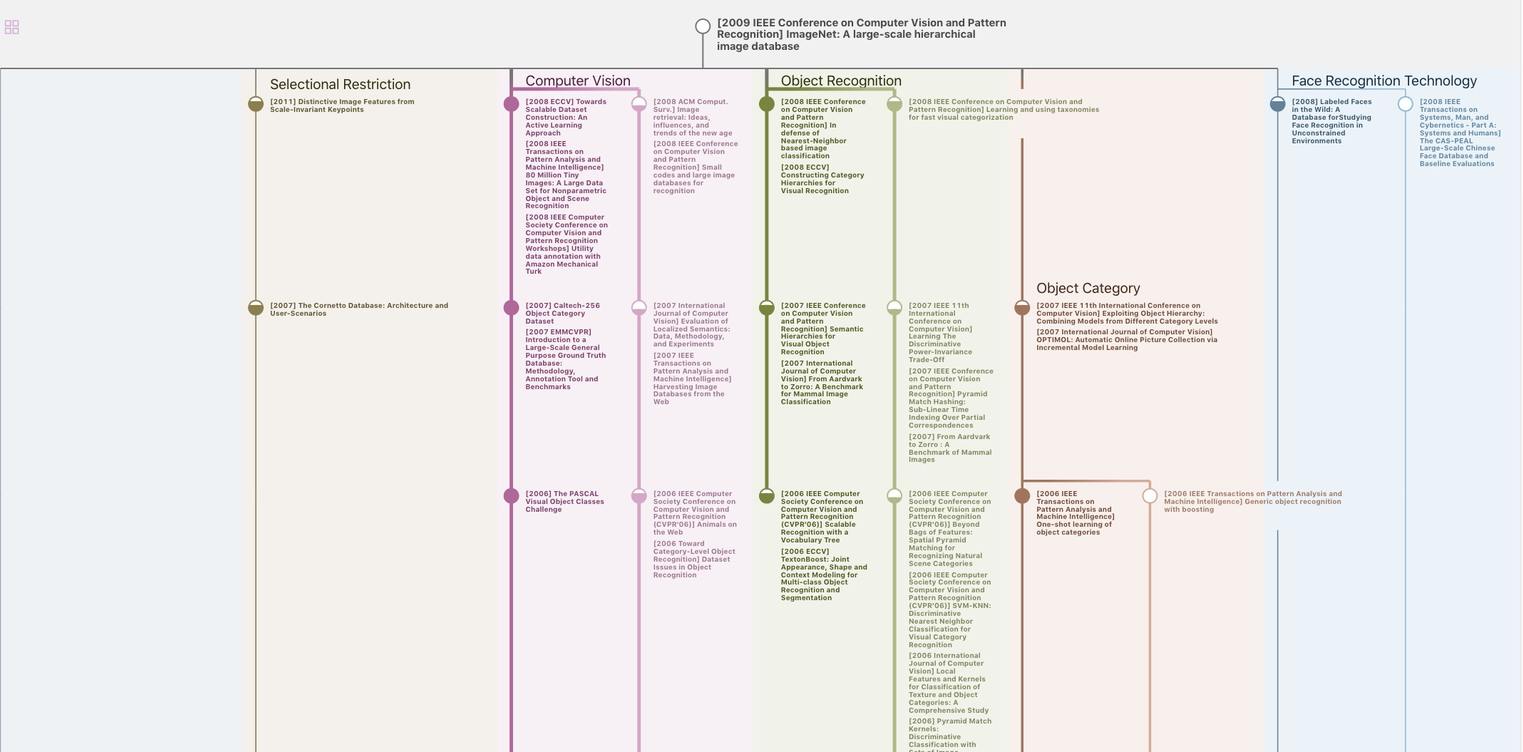
生成溯源树,研究论文发展脉络
Chat Paper
正在生成论文摘要