High-dimensional sparse covariance estimation for random signals.
ICASSP(2013)
摘要
This paper considers the problem of covariance matrix estimation from the viewpoint of statistical signal processing for high-dimensional or wideband random processes. Due to limited sensing resources, it is often desired to accurately estimate the covariance matrix from a small number of sample observations. To make up for the lack of observations, this paper leverages the structural characteristics of the random processes by considering the interplay of three widely-available signal structures: stationarity, sparsity and the underlying probability distribution of the observed random signal. New problem formulations are developed that incorporate both compressive sampling and sparse covariance estimation strategies. Tradeoff study is provided to illustrate the design choices when estimating the covariance matrices using a handful of sample observations. © 2013 IEEE.
更多查看译文
关键词
compressed sensing,covariance matrices,estimation theory,random processes,signal sampling,sparse matrices,statistical distributions,compressive sampling,covariance matrix estimation,high dimensional sparse covariance estimation,probability distribution,random signal estimation,sparsity signal structure,stationarity signal structure,statistical signal processing,wideband random process,compressed sensing,convex optimization,high-dimensional data,sparse covariance estimation
AI 理解论文
溯源树
样例
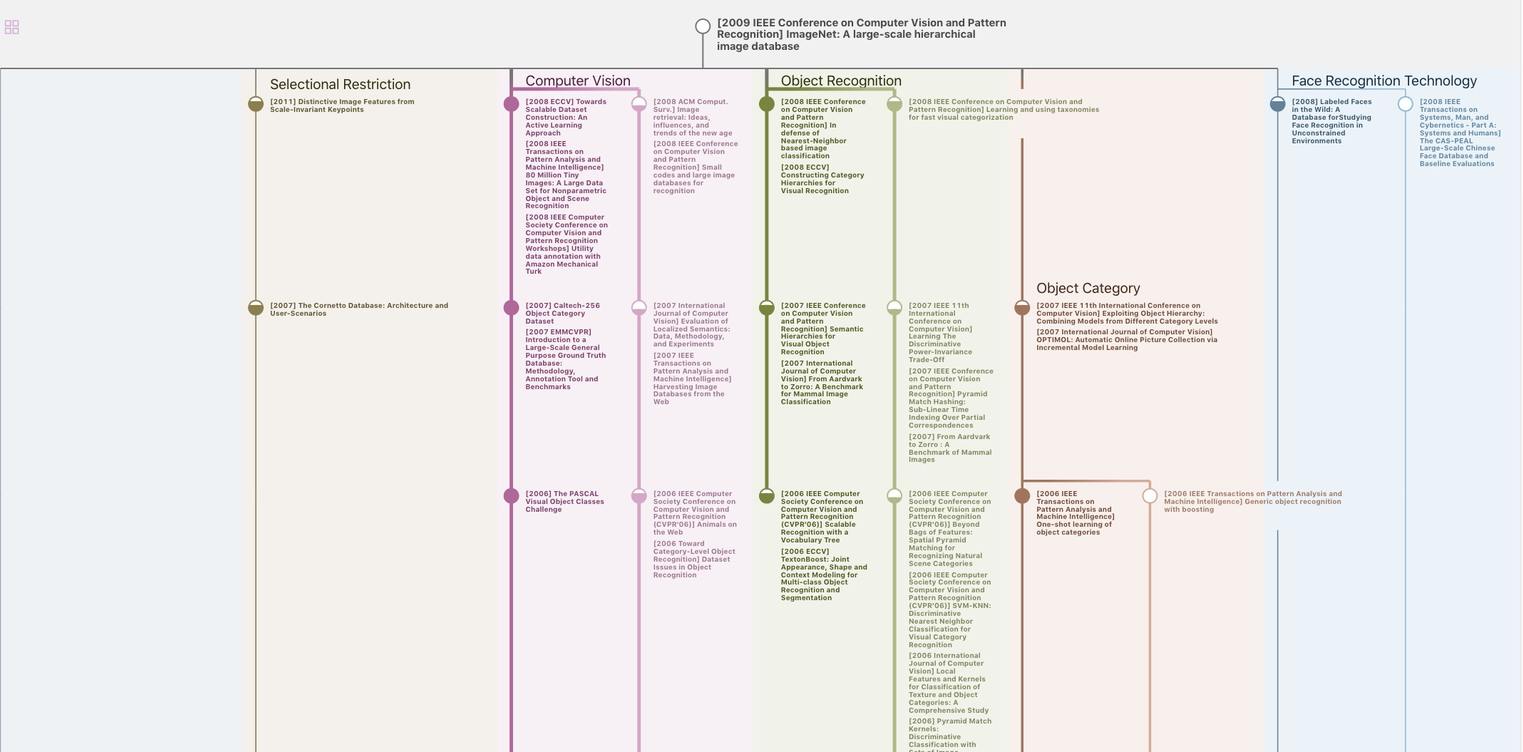
生成溯源树,研究论文发展脉络
Chat Paper
正在生成论文摘要