Content-based filtering for semi-structured documents
Content-based filtering for semi-structured documents(2013)
摘要
Unlike unstructured documents which only consist of plain text, semi-structured documents contain plenty of structured information including metadata, document fields, annotations, etc. Content-based filtering is to identify user-interested documents from a stream of documents based on the analysis of document content. When dealing with semi-structured documents, many existing filtering approaches either ignore the structured information, or simply use them as features. This dissertation focuses on the better use of document structured information for content-based filtering. We find that structured information is useful in the following problems. First, structured information is useful for user profile initialization in topic tracking tasks. At the early stage of a topic tracking task, the system performance tends to be low due to the limited number of labeled documents from the user. To deal with this problem, we propose two new user feedback mechanisms based on document structured information (facet-value pairs and Wikipedia concepts respectively). The new feedback mechanisms allow the system to quickly get some feedback from the user and refine the user profile. Our experiment results show that the new user feedback can significantly improve the filtering performance in topic tracking tasks. Second, structured information is useful for semi-structured document summarization in retrieval/filtering tasks with user quries. In a retrieval/filtering task where many documents are delivered, the user selects documents to read based on the short summaries of documents. In this sense, document summaries should be informative enough so that the user can make right decisions on which documents to read. To achieve this goal, we propose a new document-summarization method that can generate better summaries for semi-structured documents with rich metadata in filtering/retrieval scenarios. Third, structured information can be easily incorporated into discriminative models for personalized recommendation. We propose two flexible Bayesian hierarchical models for joint user profile learning. The proposed models are discriminative, thus can easily incorporate various types of document structured information. They also have the advantages of being able to borrow discriminative information from similar users and capture multiple interests of individual users.
更多查看译文
关键词
new user feedback,user profile,new user feedback mechanism,discriminative information,joint user profile learning,individual user,semi-structured document,similar user,structured information,user profile initialization
AI 理解论文
溯源树
样例
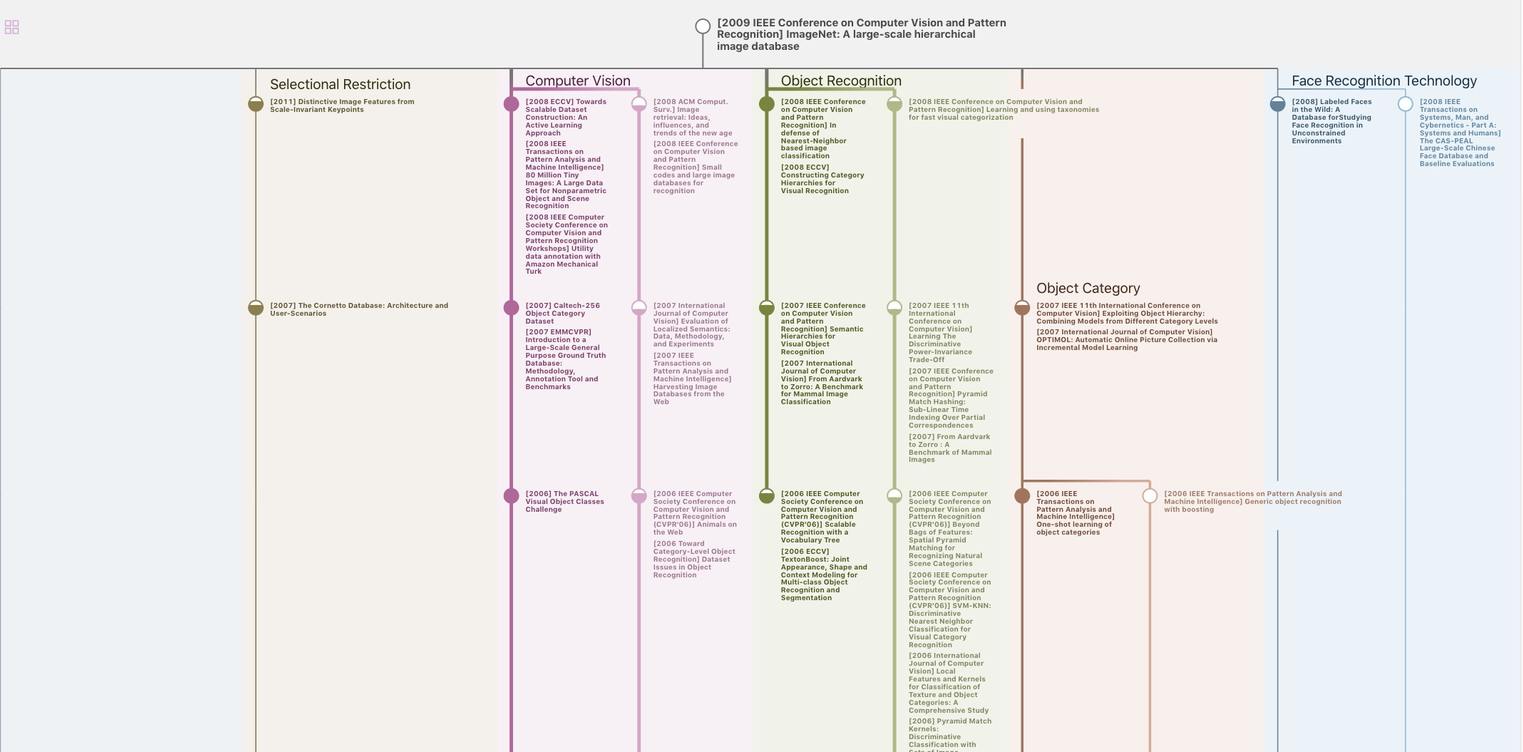
生成溯源树,研究论文发展脉络
Chat Paper
正在生成论文摘要