Exploration in Model-based Reinforcement Learning by Empirically Estimating Learning Progress.
NIPS'12: Proceedings of the 25th International Conference on Neural Information Processing Systems - Volume 1(2012)
摘要
Formal exploration approaches in model-based reinforcement learning estimate the accuracy of the currently learned model without consideration of the empirical prediction error. For example, PAC-MDP approaches such as R-MAX base their model certainty on the amount of collected data, while Bayesian approaches assume a prior over the transition dynamics. We propose extensions to such approaches which drive exploration solely based on empirical estimates of the learner's accuracy and learning progress. We provide a "sanity check" theoretical analysis, discussing the behavior of our extensions in the standard stationary finite state-action case. We then provide experimental studies demonstrating the robustness of these exploration measures in cases of non-stationary environments or where original approaches are misled by wrong domain assumptions.
更多查看译文
AI 理解论文
溯源树
样例
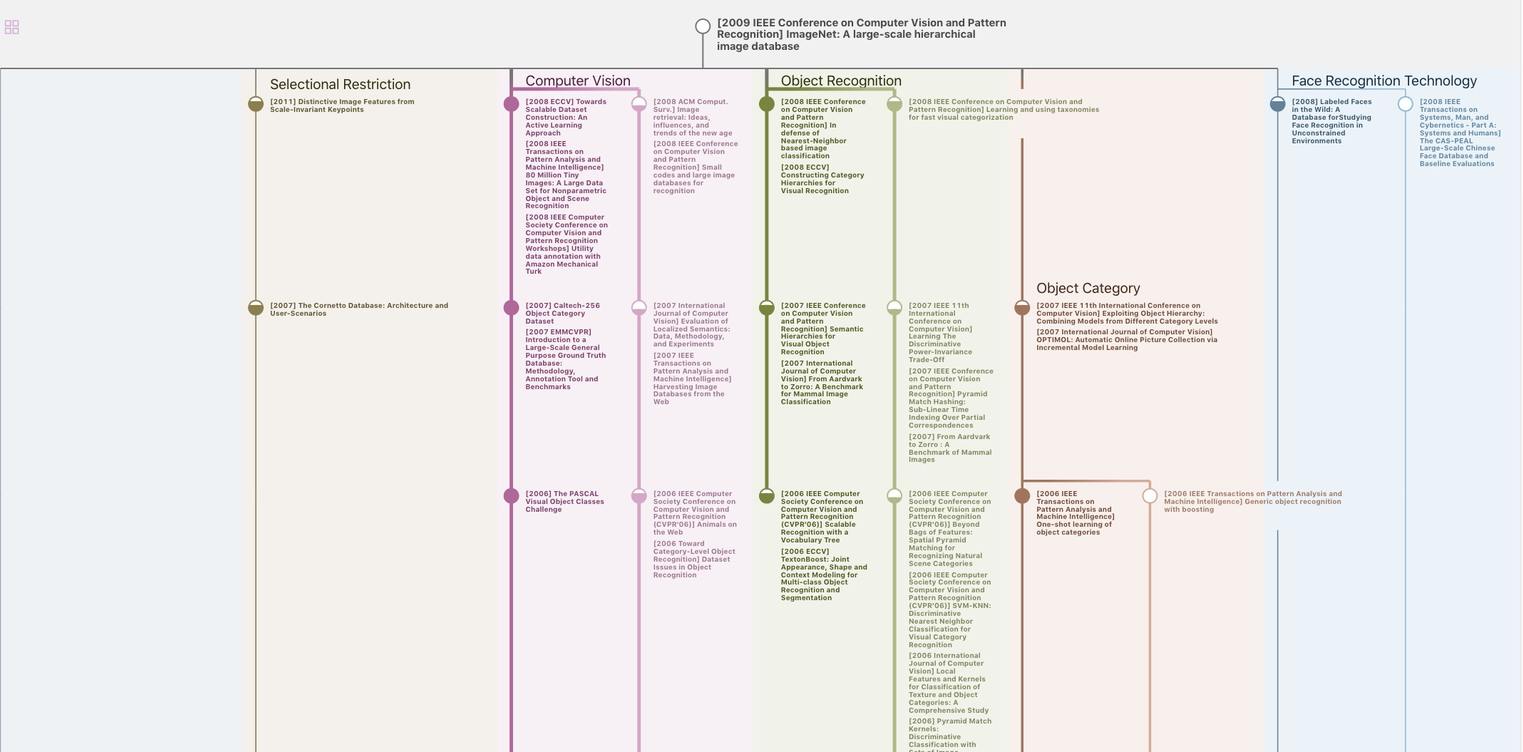
生成溯源树,研究论文发展脉络
Chat Paper
正在生成论文摘要