Ensemble Detection of Single & Multiple Events at Sentence-Level.
CoRR(2014)
摘要
Event classification at sentence level is an important Information Extraction task with applications in several NLP, IR, and personalization systems. Multi-label binary relevance (BR) are the state-of-art methods. In this work, we explored new multi-label methods known for capturing relations between event types. These new methods, such as the ensemble Chain of Classifiers, improve the F1 on average across the 6 labels by 2.8% over the Binary Relevance. The low occurrence of multi-label sentences motivated the reduction of the hard imbalanced multi-label classification problem with low number of occurrences of multiple labels per instance to an more tractable imbalanced multiclass problem with better results (+ 4.6%). We report the results of adding new features, such as sentiment strength, rhetorical signals, domain-id (source-id and date), and key-phrases in both single-label and multi-label event classification scenarios.
更多查看译文
关键词
ensemble detection,multiple events,sentence-level
AI 理解论文
溯源树
样例
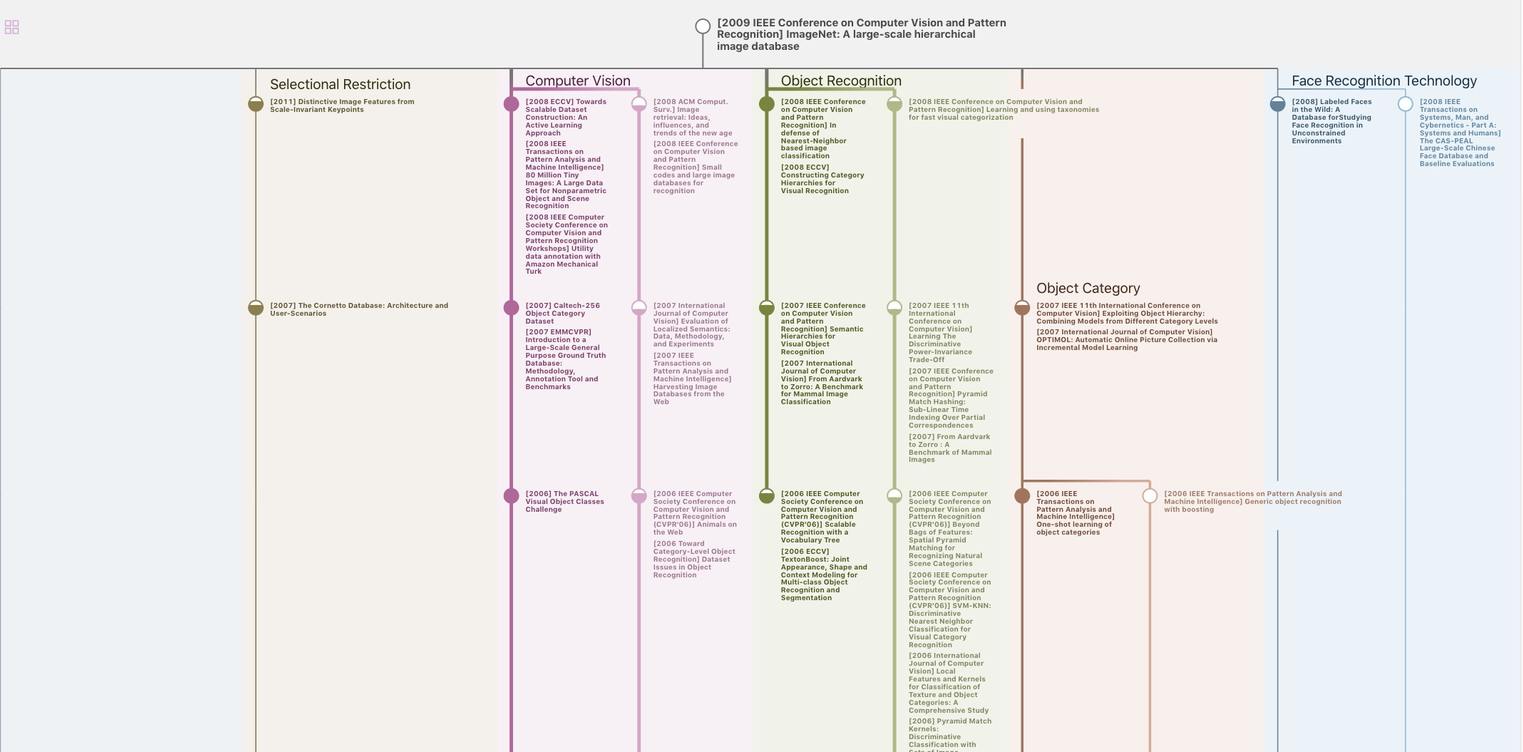
生成溯源树,研究论文发展脉络
Chat Paper
正在生成论文摘要