DNA and peptide sequences and chemical processes multivariately modelled by principal component analysis and partial least-squares projections to latent structures
Analytica Chimica Acta(1993)
摘要
Biopolymer sequences (e.g., DNA, RNA, proteins and polysaccharides) and chemical processes (e.g., a batch or continuous polymer synthesis run in a chemical plant) have close similarities from the modelling point of view. When a set of sequences or processes is characterized by multivariate data, a three-way data matrix is obtained. With sequences the position and with processes the time is one direction in this matrix. The multivariate modelling of this matrix by principal component analysis (PCA) or partial least-squares (PLS) methods for the following purposes is discussed: classification of sequences; quantitative relationships between sequence and biological activity or chemical properties; optimizing a sequence with respect to selected properties; process diagnostics; and quantitative relationships between process variables and product quality variables. To obtain good models, a number of problems have to be adequately dealt with: appropriate characterization of the sequence or process; experimental design (selecting sequences or process settings); transforming the three-way into a two-way matrix; and appropriate modelling and validation (modelling interactions, periodicities, “time series” structures and “neighbour effects”). A multivariate approach to sequence and process modelling using PCA and PLS projections to latent structures is discussed and illustrated with several sets of peptide and DNA promoter data.
更多查看译文
关键词
Process analysis / on-line analysis,Pattern recognition,Biopolymer sequences,DNA sequences,Multivariate modelling,Partial least squares,Peptide sequences,Principal component analysis
AI 理解论文
溯源树
样例
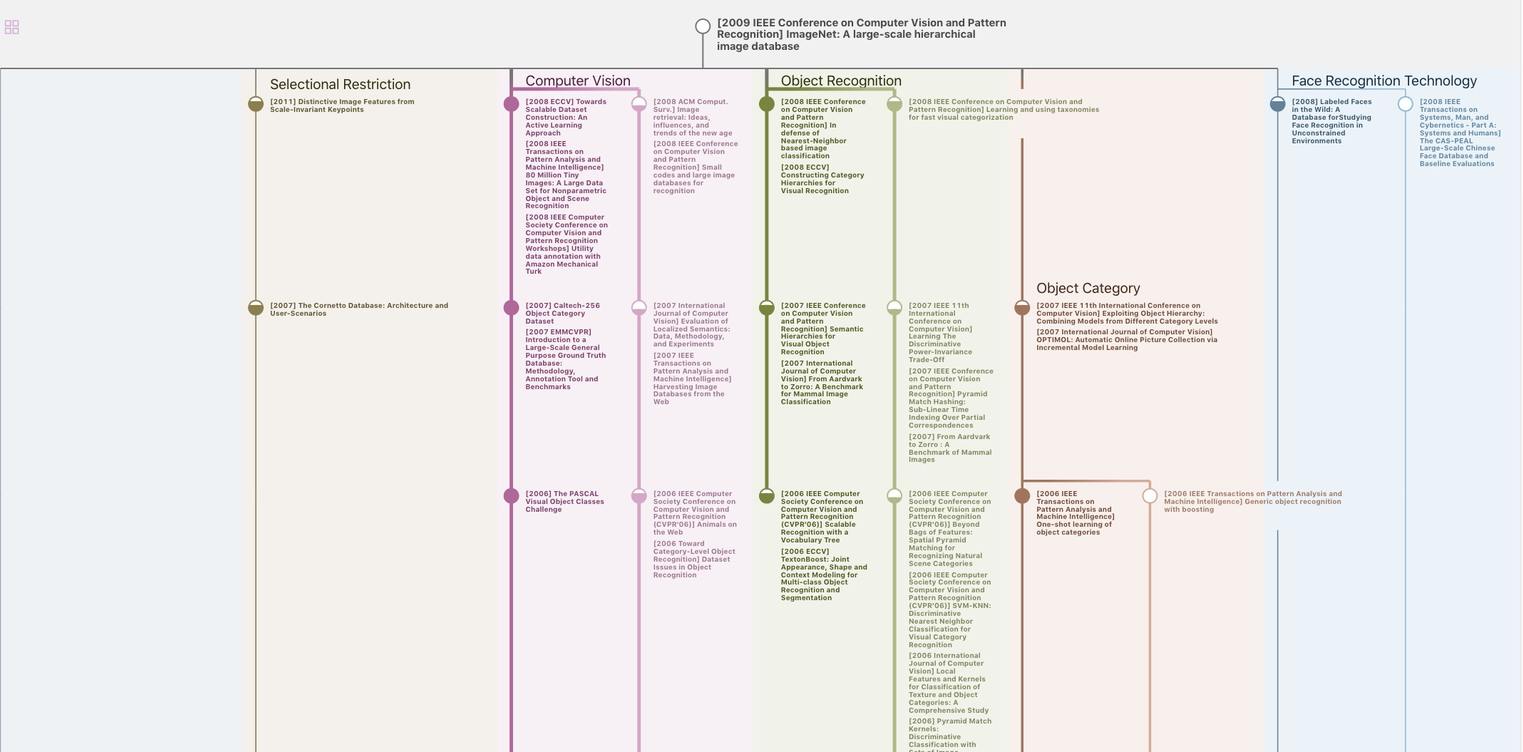
生成溯源树,研究论文发展脉络
Chat Paper
正在生成论文摘要