Tied-state based discriminative training of context-expanded region-dependent feature transforms for LVCSR
ICASSP(2013)
摘要
We present a new discriminative feature transform approach to large vocabulary continuous speech recognition (LVCSR) using Gaussian mixture density hidden Markov models (GMM-HMMs) for acoustic modeling. The feature transform is formulated with a set of context-expanded region-dependent linear transforms (RDLTs) utilizing both long-span features and contextual weight expansion. The RDLTs are estimated by lattice-free, tied-state based discriminative training using maximum mutual information (MMI) criterion, while the GMM-HMMs are trained by conventional lattice-based, boosted MMI training. Compared with two baseline systems, which use RDLTs with either long-span features or weight expansion only and are trained using the conventional lattice-based discriminative training for both RDLTs and HMMs, the proposed approach achieves a relative word error rate reduction of 10% and 6% respectively on Switchboard-1 conversational telephone speech transcription task.
更多查看译文
关键词
maximum mutual information,lvcsr,acoustic modeling,speech recognition,large vocabulary continuous speech recognition,region-dependent linear transforms,switchboard-1 conversational telephone speech transcription task,gaussian mixture density hidden markov models,hmm,discriminative feature transform,vocabulary,discriminative training,gaussian processes,gmm-hmm,tied-state,context-expanded region-dependent feature transforms,tied-state based discriminative training,region-dependent linear transform,hidden markov models,acoustics,vectors,speech
AI 理解论文
溯源树
样例
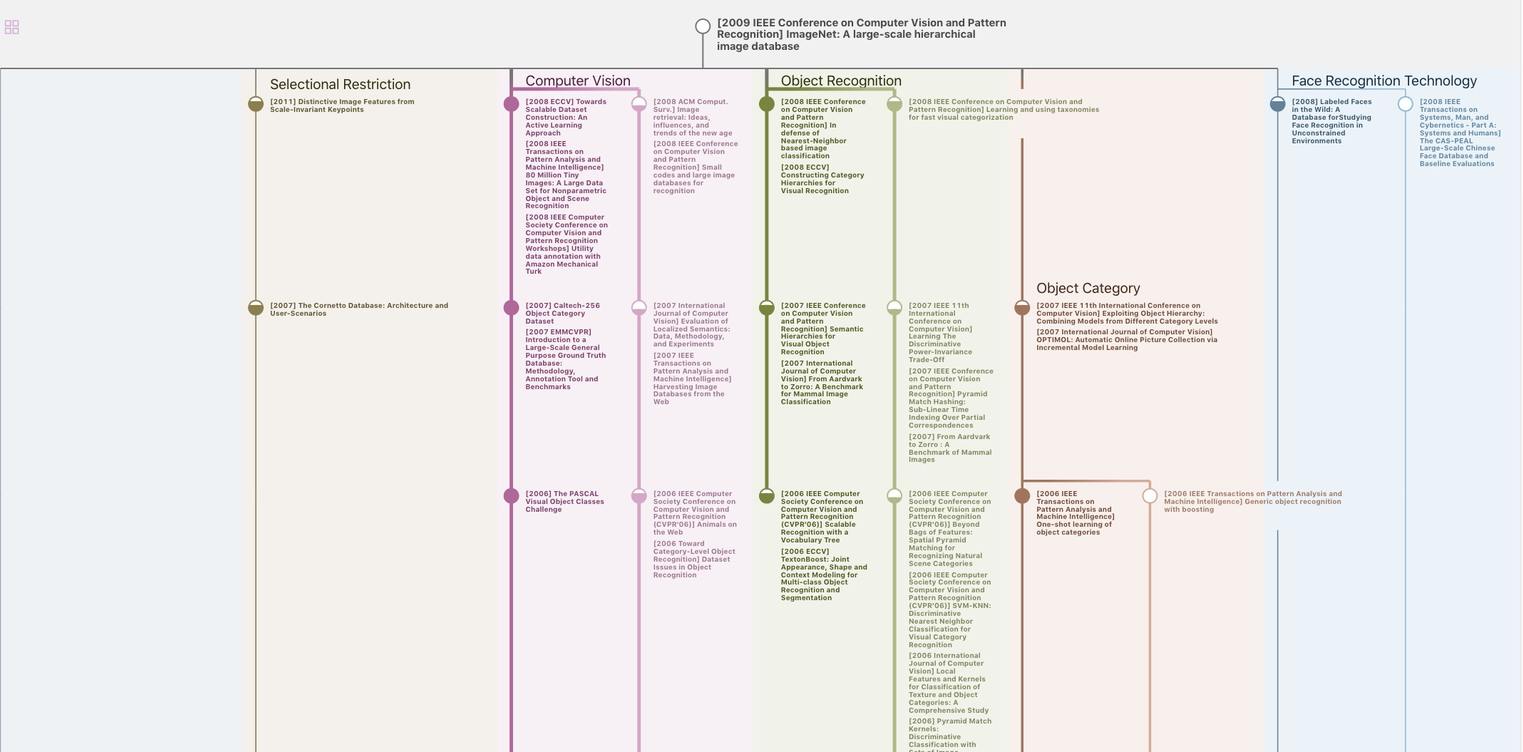
生成溯源树,研究论文发展脉络
Chat Paper
正在生成论文摘要