Local Discriminative Orthogonal Rank-One Tensor Projection for image feature extraction
ACPR(2011)
摘要
This paper develops a Local Discriminative Orthogonal Rank-One Tensor Projection (LDOROTP) technique for image feature extraction. The goal of LDOROTP is to learn a compact feature for images meanwhile endow the feature with prominent discriminative ability. LDOROTP achieves the goal through a serial of rank-one tensor projections with orthogonal constraints. To seek the optimal projections, LDOROTP carries out local discriminant analysis, but differs from the previous works on two aspects: (1)the local neighborhood consists of all the samples of the same class and partial local samples from different classes; (2)a novel weighting function is designed to encode the local discriminant information. The criterion of LDOROTP is built on the trace differences of matrices rather than the trace ratio, so the awkward problem of singular matrix do not emerges. Besides, LDOROTP benefits from an efficient and stable iterative scheme of solution and a data preprocessing called GLOCAL tensor representation. LDOROTP is evaluated on face recognition application on two benchmark databases: Yale and PIE, and compared with several popular projection techniques. Experimental results suggest that the proposed LDOROTP provides a supervised image feature extraction approach of powerful pattern revealing capability.
更多查看译文
关键词
local discriminant analysis,face recognition,glocal tensor representation,local discriminative orthogonal rank-one tensor projection,image feature extraction,feature extraction,orthogonal constraints,ldorotp,popular projection techniques,face recognition application,stable iterative scheme,novel weighting function,tensors,iterative methods,principal component analysis,delta modulation,weight function,data preprocessing,image features,databases,strain,discriminant analysis
AI 理解论文
溯源树
样例
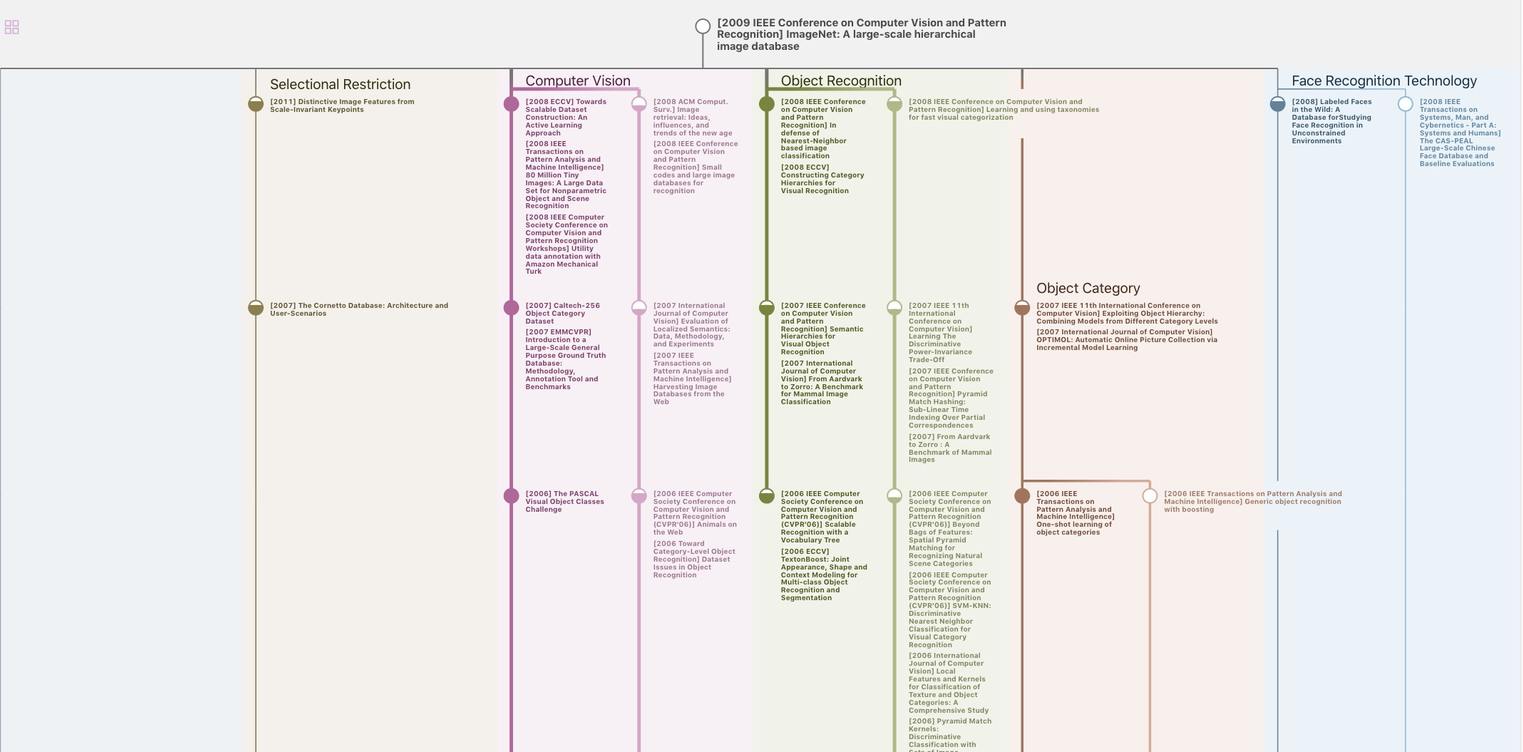
生成溯源树,研究论文发展脉络
Chat Paper
正在生成论文摘要