Robustness Analysis Of Neural Networks With An Application To System Identification
JOURNAL OF GUIDANCE CONTROL AND DYNAMICS(1999)
摘要
The robustness characteristics of multilayered feedforward neural networks (NNs) are analyzed using linear systems theory. Robustness of NN is defined as the NN's ability to perform within certain bounds of its nominal (without uncertainty) performance in the presence of bounded uncertainty. An induced Euclidean matrix norm is used to derive error bounds for NN with activation functions that exhibit predominantly linear behavior. Lyapunov stability theory is used to derive bounds on the nonlinear variations in NNs with activation functions that exhibit nonlinear behavior. A Monte Carlo simulation analysis is conducted to examine the robustness characteristics of fully and sparsely connected networks. The following conclusions are drawn on the basis of the analysis: 1) sparsity in the NN connection topology is highly desirable to achieve robustness; 2) two-hidden-layer networks with an equal number of neurons in each layer exhibit very poor robustness; 3) a fully forward connected network with sparsity is the most robust and accurate for a given number of neurons; and 4) for NNs with many neurons, activation functions with highly nonlinear regions exhibit poor robustness. A system identification of a nonlinear model is presented to reinforce the results of the robustness analysis.
更多查看译文
关键词
neural network,system identification
AI 理解论文
溯源树
样例
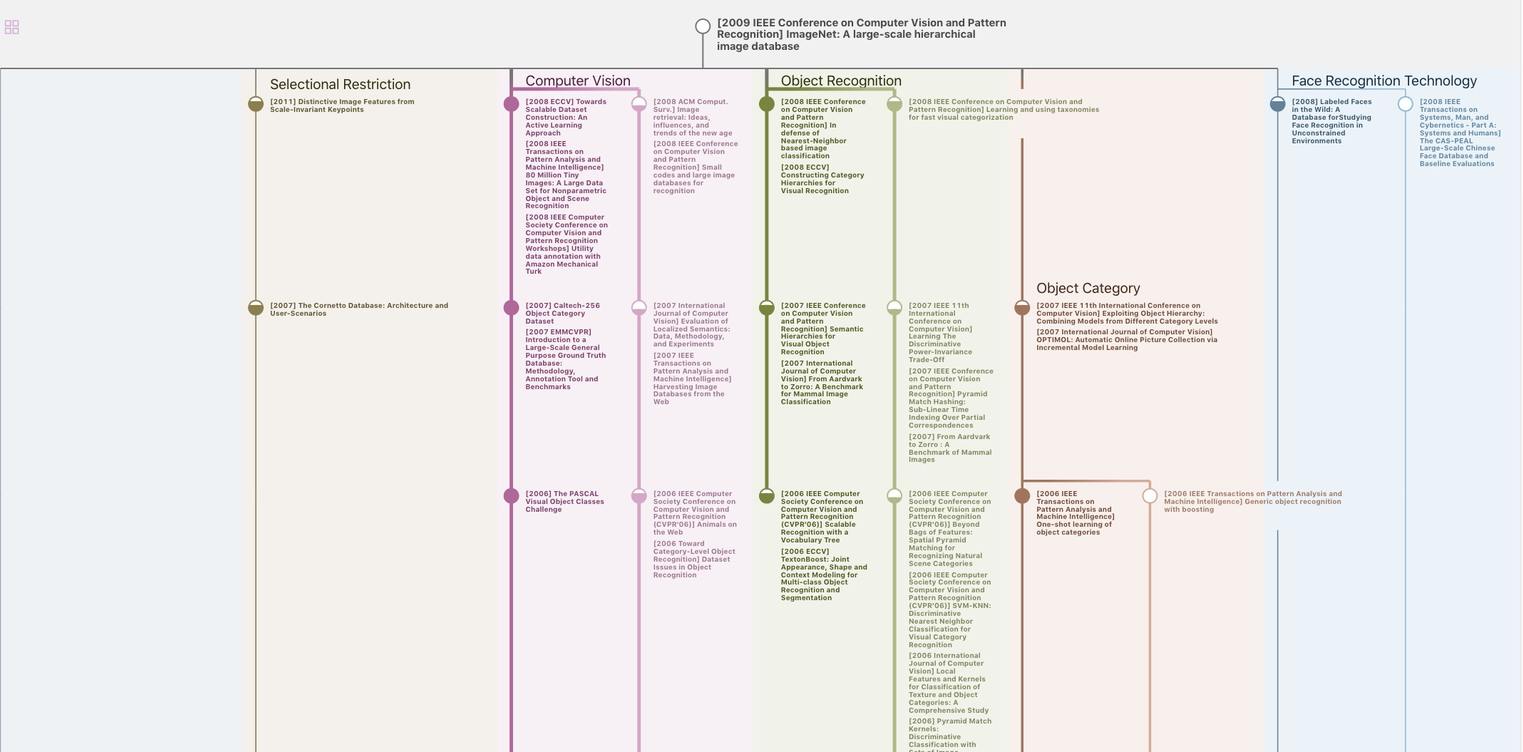
生成溯源树,研究论文发展脉络
Chat Paper
正在生成论文摘要