Detection-based speech recognition with sparse point process models
ICASSP(2010)
摘要
We present a bottom-up approach to connected digit recognition in which (i) the speech signal is transformed into a sparse set of acoustic events in time, (ii) point process models (PPM) of these events are used to detect candidate digit occurrences, and (iii) the candidate digit detections are reduced to a single digit sequence prediction by using a previously proposed graph-based optimization. We find the performance of this detection-based system on the AURORA2 evaluation matches that of an HTK baseline in clean speech and provides improved robustness to non-stationary noise. A similar robustness to stationary noise sources is achieved with unsupervised PPM adaptation using small amounts of the noisy data.
更多查看译文
关键词
optimisation,speech recognition,AURORA2 evaluation matches,HTK baseline,acoustic events,connected digit recognition,detection-based speech recognition,detection-based system,graph-based optimization,noisy data,sparse point process models,speech signal,stationary noise sources,unsupervised PPM adaptation,speech processing,speech recognition
AI 理解论文
溯源树
样例
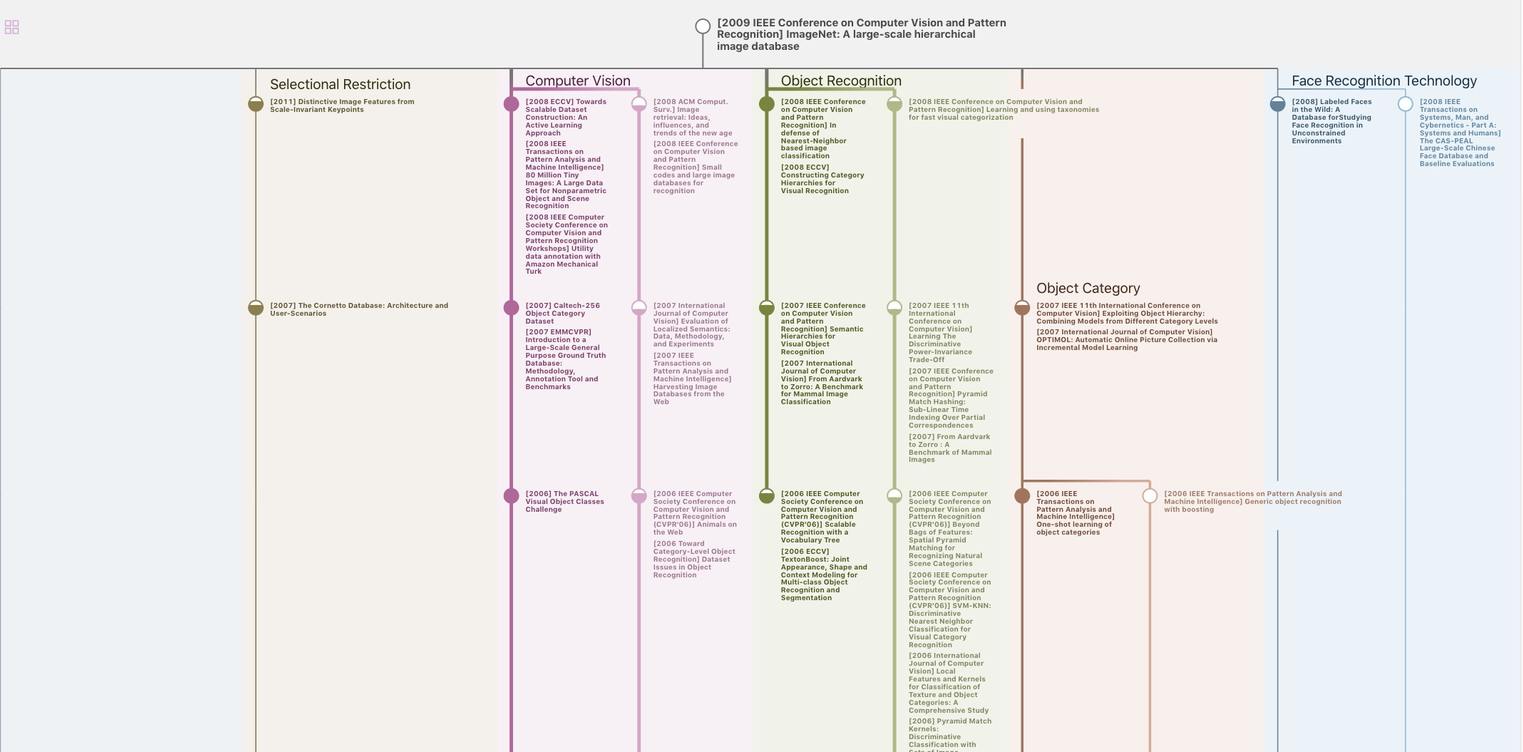
生成溯源树,研究论文发展脉络
Chat Paper
正在生成论文摘要