Feature Selection Using Ensemble Based Ranking Against Artificial Contrasts
2006 IEEE INTERNATIONAL JOINT CONFERENCE ON NEURAL NETWORK PROCEEDINGS, VOLS 1-10(2006)
摘要
In contrast to typical variable selection methods such as CFS, tree-based ensemble methods can produce numerical importances of input variables of mixed type considering all variable interactions, not just one or two variables at a time. However, they do not indicate a cut-off point: how to set a threshold to the importance. This paper presents an efficient approach to doing this using artificial contrast variables. The result is a truly autonomous variable selection method in both multilevel classification and regression settings that can handle huge number of variables of mixed type with potentially non randomly missing values, resistant to noise both in input and response space, considers all variable interactions, and does not require a pre-set number of important variables.
更多查看译文
关键词
missing values,feature selection,variable selection,regression analysis,classification
AI 理解论文
溯源树
样例
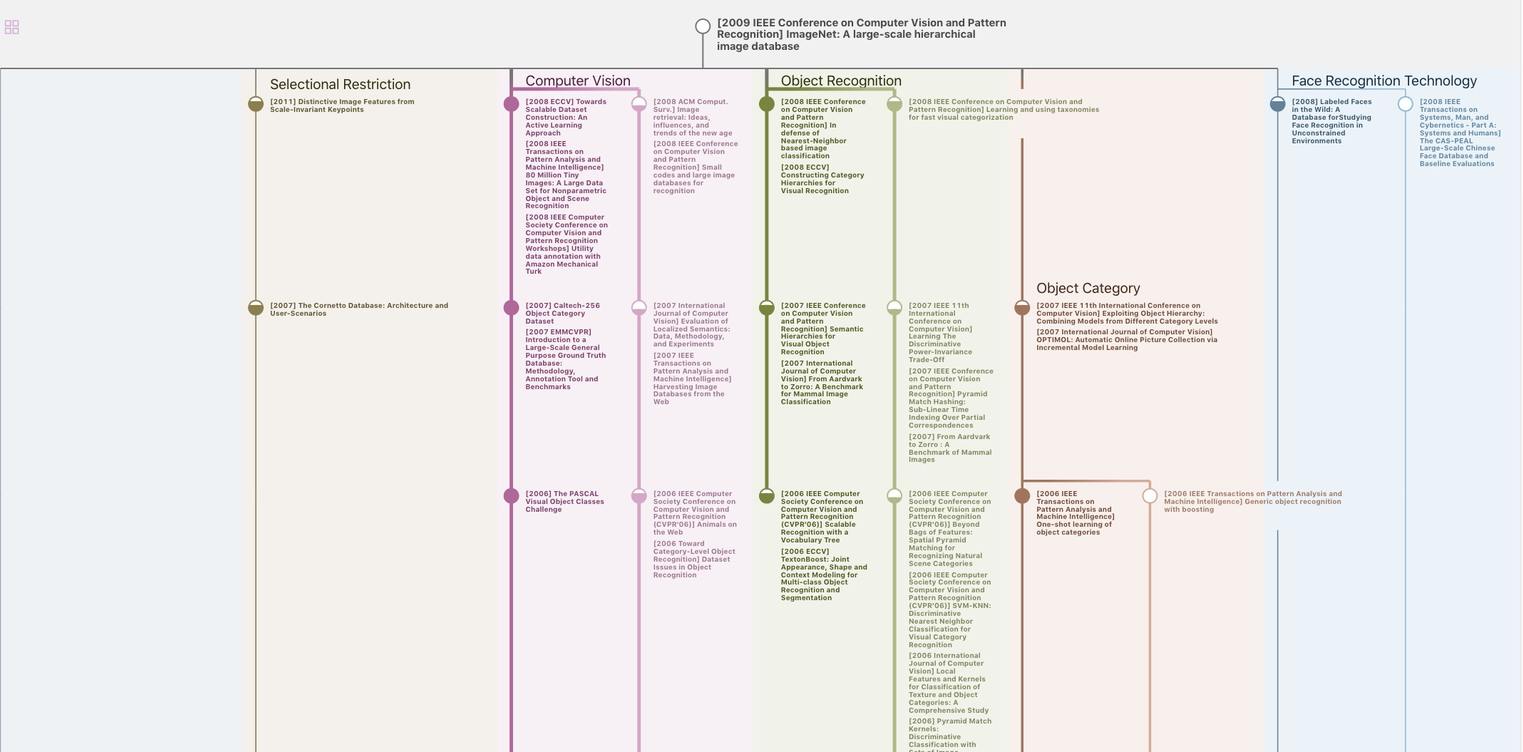
生成溯源树,研究论文发展脉络
Chat Paper
正在生成论文摘要