Fine spatiotemporal structure of phase in human intracranial EEG
Clinical Neurophysiology(2006)
摘要
Methods EEGs were recorded at high spatial resolution from a 1×1 cm 8×8 electrode array on the right inferior temporal gyrus of a patient undergoing preoperative monitoring for epilepsy surgery. Cosines were fitted to EEG segments to measure frequency and phase and compute location, size, latency, phase velocity, duration, and recurrence rate of radially symmetric spatial patterns called phase cones. The Hilbert transform was also used to get high temporal resolution. Results In the awake state, the power spectral density (PSD) showed power-law decrease in log power with log frequency at 1/ f α , α ∼2, but with peaks in the standard empirical ranges. The phase in beta and gamma ranges had spatial gradients in conic form. Resetting of these stable spatial patterns of phase cones was spatially coincident at intermittent discontinuities (‘phase slip’) recurring at theta rates. Cones had half power diameters from 2 to 50+ mm; their durations had power-law distributions with values ranging from 6 to 300+ ms depending on length of the analysis window. In slow wave sleep PSD decreased at 1/ f α , α ∼3,with loss of beta–gamma spectral peaks and diminished or absent oscillations and spatiotemporal phase structure. Conclusions Spatiotemporal structures in awake human and rabbit EEG showed striking similarities. The only clear differences were ascribable to differing scales of measurement. These fine spatiotemporal structures of EEG were diminished or lost in slow wave sleep. Significance The fine structure indicates that neocortical stability is sustained at self-organized criticality; that synaptic input in the awake state drives neocortex away from criticality causing beta–gamma oscillations in re-stabilizing ‘neural avalanches’; and that diminished input in slow wave sleep allows return toward criticality but with some added risk of instability and seizure. Keywords Coordinated analytic phase differences CAPD Hilbert transform Human EEG Phase gradient Self-organized criticality Sleep Phase transition Synchrony 1 Introduction Synchrony among large populations of neurons is widely inferred to be essential for cognitive behavior (e.g. Breakspear and Friston, 2001; Bressler and Kelso, 2001; Chapman et al., 2002; Engel et al., 2001; Friston, 2000; Grinvald et al., 2003; Stam et al., 2003; Tsuda, 2001; Von Stein et al., 2000 ). Phase is often used to estimate the degree of synchrony ( Bressler et al., 1993; Palva et al., 2005; Rodriguez et al., 1999; Singer and Gray, 1995 ; Tallon-Baudry et al., 1998 ; Van Putten, 2003; Varela et al., 2001 ), so that phase is important in studies of the neural basis for cognition. There are two methods in common use to measure phase ( Bruns, 2004; Freeman, 2004b; Le Van Quyen et al., 2001; Quiroga et al., 2002 ). First is the Fourier transform, a linear operator that is used to measure phase in a time window that is long enough to include at least one cycle of the frequency at which the phase is defined. Then phase serves to measure time lags among two or more signals oscillating at the same frequency in that window. Second is the Hilbert transform, also a linear operator that gives a complex function, the analytic signal, which can be decomposed into the ‘instantaneous’ analytic amplitude and analytic phase at each digitizing step. Calculation of the difference in analytic phase between each pair of successive digitizing steps approximates the rate of change in phase. When divided by the digitizing step the phase difference gives the ‘instantaneous’ frequency in radians/s, which for aperiodic ‘chaotic’ EEG signals varies continuously. The analytic phase differences from multiple EEGs can be used to locate frames in which the rate of phase change approaches a constant value, meaning that the change in frequency is small enough to define and measure the simultaneous phase differences among multiple traces at a mean frequency. Two or more peaks in PSD at such frequencies are often seen each with its overlapping phase cone (Freeman and Barrie, 2000). The stabilization of multiple frequencies at low rates of change and the recurrence of frames at alpha and theta frequencies might explain some observations of cross-frequency synchrony reported by Palva et al. (2005); and Von Stein et al. (2000) . The larger and longer-lasting frames are stationary epochs with stable spatial patterns of phase and amplitude that resemble frames in a cinema. These frames are prime targets for measurements of phase ( Freeman, 2003, 2004b ) and amplitude ( Rodriguez et al., 1999 ; Tallon-Baudry et al., 1998; Varela et al., 2001 ) of beta and gamma oscillations in relation to cognitive behavior ( Freeman, 2005b, in press-b ). Prior studies of the analytic phase in humans ( Freeman et al., 2003a,b ) and animals ( Freeman, 2004b ; Freeman and Rogers, 2002 ; Freeman and Rogers, 2003 ) have revealed two types of structure: one in the time domain, the other in the spatial domain. First, in the time domain the analytic phase shows episodic discontinuities (hereafter called ‘phase slip’ ( Pikovsky et al., 2001 )) that characteristically result from application of the Hilbert transform to aperiodic signals. Phase slip appears as jumps forward or backward in the phase of a signal from an otherwise progressive increase in analytic phase by small steps. When the jumps occur nearly simultaneously in multiple channels of EEG over broad areas, they are called ‘coordinated analytic phase differences’ (CAPD). They serve to bracket the frames having steady rates of increase in phase (nearly constant frequency). Second, in the spatial domain a set of 64 phase values from an 8×8 microgrid form a phase surface at each digitizing step that can often be fitted with a right cone that gives lower residuals than either a plane or a bivariate Gaussian function. This structure is referred to as a ‘phase cone’. When a phase cone persists over 10 or more digitizing steps (50+ ms at 5 ms steps, the criterion used in the present study), it defines a frame that is bracketed by phase slips signifying the beginning and end of the frame by cortical phase transitions ( Freeman and Kozma, 2002 ; Kozma et al., 2005 ). Within the frame the frequency varies a few percent from the mean frequency, so that the temporal power spectral density (PSD T ) of the frame gives a peak at that frequency. While noise is the most common cause of phase slip ( Freeman, in press-a ), the near-synchronous CAPD also contribute but are not noise. They are often prelude to emergence by phase transitions of amplitude modulation (AM patterns) that relate to behavior. Such changes in state imply that cortex is unstable in the sense of jumping between states, yet conditionally stable in the sense that states in sequences are controlled and orderly. The present study was limited by the location of the microgrid far from any primary sensory area in a subject unable to function at a high cognitive level, so study of AM patterns in relation to CS was not possible. However, the opportunity arose to observe EEGs in slow wave sleep (SWS; REM sleep could not be identified), as well as in relation to the onsets of simple and complex partial seizures ( Freeman et al., in press ), which had not been undertaken in the animal studies, and which gave results that addressed the nature of cortical conditional stability. The aim of the present report is to present these findings in a preliminary database of EEG from an intracranial microgrid implanted in a human and compare it with rabbit data reported in this journal ( Freeman, 2004a,b, 2005a ). Evaluation is undertaken of the extent to which observed differences with the subjects at rest ( Freeman, in press-a ) resulted from the scales of measurement at the different digitizing rates (5 vs. 2 ms) and interelectrode distances (1.25 vs. 0.79 mm). The power-law distributions of the durations and diameters of the phase patterns suggested fractal properties by which the mean values and variances might depend on the scales of measurement ( King, 1991; Mandelbrot, 1983; Pereda et al., 1998 ). 2 Methods 2.1 Experimental subject and EEG recording The subject was a 34-year-old woman with a history of medically refractory complex partial seizures. As part of her pre-operative evaluation for surgical therapy, intracranial, subdural strip electrodes were placed through temporal burr holes over both lateral and basal-temporal regions. She underwent continuous EEG-video monitoring for 8 days to record seizures. At the time of placement of the clinical electrodes, the attending neurosurgeon (GAW) placed a high-density electrode microgrid through the right temporal burr hole onto the surface of the right inferior temporal gyrus. The length of monitoring time was dictated solely by clinical considerations; the microgrid was removed at the same time as the clinical electrodes. The subject underwent the procedure with no complications. The data were collected in the EEG and Clinical Neurophysiology laboratory of Harborview Hospital, University of Washington, Seattle, and sent by ftp without identifying personal information to the University of California at Berkeley for analysis. The data collection and management were governed by protocols approved by Institutional Review Boards in both institutions. Prior to the operation the patient gave informed consent for placement of the clinical electrodes and the microgrid. The data set used for the present review consisted of approximately 2 min of recording with the subject awake and at rest ( Fig. A1 in Appendix A ) at least 2 h prior to seizure onsets, in the 2 min prior to onsets, and an additional 2 min with the subject in SWS, verified by inspection of the video and the EEG from the surgical montage. The microgrid was an 8×8 array of 0.5 mm stainless steel wires spaced 1.25 mm apart (aperture 10×10 mm). Prior derivation of the spatial power spectral density (PSD X ) of the human subdural EEG ( Freeman et al., 2000 ) demonstrated that 1.25 mm spacing was optimal for recording spatial textures of the EEG, while the overall size of the grid was small enough to fit onto a single gyrus. The EEG was amplified with a Nicolet BMSI 5000 system having a fixed gain of 1628 and analog filters set at 0.5 Hz high pass and 120 Hz low pass. The ADC gave 12 bits with the least significant bit of 0.9 μV and maximal range of ±2048×0.9 μV. DC offsets of the RC amplifier outputs were removed off-line by subtracting the channel means of every entire recording block. The reference and ground placements for all 64 electrodes were, respectively, the Cz and Pz scalp locations (midline frontal and parietal sites in the standard 10–20 clinical system ( Barlow, 1993 )). The Nicolet system digitized data at 420 Hz and down-sampled to 200 Hz. Off-line low pass filtering at or below 55 Hz was performed to minimize 60 Hz. 2.2 Preprocessing EEG and calculating analytic phase and frequency by the Hilbert method The analytic phase was measured with the Hilbert transform and with the Fourier transform with respect to the phase of the average value (HT) or average waveform (FFT) over the 64 waveforms in the same frame. These linear methods have been shown to give the same results given the same filter settings ( Bruns, 2004; Freeman, 2004b; Le Van Quyen et al., 2001; Quiroga et al., 2002 ), and they were both required to analyze the data, because each method had a limit on its range of application. On the one hand the Hilbert transform served to track phase differences in multichannel EEG signals only within the duration between break points ( Fig. 1 C) of the arctangent function and, therefore, maximally within the wavelength of one cycle of the peak frequency. However, the phase values differed among the 64 EEGs, so that the times at which the break points occurred varied across the array (Fig. 2 in Freeman, 2004b; Freeman et al., 2003a,b ). An obvious circumstance of spurious phase slip was seen in narrow-band oscillation when the amplitude of the signal was almost tangent to the zero line. A small difference in the amplitude of a low-frequency component determined whether or not crossing occurred with or without passage of the vector in a polar plot across the imaginary axis. Small variations in the amplitude of a low-frequency component across multiple traces led to near-random splay of the unwrapped phase values with π radians (or 2π radians with use of the 4-quadrant arctangent function) being added to some signals but not others. This feature was not problematic with temporal phase differences (CAPD) for which the phase reference was the preceding value in time, but it invalidated measurements of spatial phase gradients in the temporal average that was implicit in phase cones, for which the phase reference was remote by more than one cycle of the narrow-band oscillation. While this circumstance was not common, it was neither rare nor predictable in multichannel EEG recording. The actual limit on tracking phase differences in multiple EEGs simultaneously recorded was the wavelength minus the time interval between the onsets of the first and last break points on the 64 channels at each CAPD. Unwrapped analytic phase differences suppressed the break points, so that their coordination as CAPD could be seen more clearly, but the addition of π or 2π radians at different times made it impossible to fit a conic surface to phase values from multiple EEGs taken across a break point. On the other hand under Fourier analysis the durations of the selected EEG segments had to exceed one cycle of the frequency at which the phase was to be defined, but the durations of the selected segments could not exceed the duration of EEG epochs having the stationarity given by low rates of change in phase between the CAPD. Hence, even though the Fourier- and Hilbert- based methods for measuring phase were mathematically equivalent ( Bruns, 2004 ) for many filter settings, they were complementary in covering the full range of temporal resolution needed to comprehend phase structures, with the advantage going to the FFT for low rates of phase change and to the HT for high rates. The techniques employed within these limitations were developed in rabbit studies. The same techniques were used in the human studies, except that adjustments were made in filter settings and segment durations to adapt to the differences in digitizing rates and interelectrode distances. As described in previous reports ( Freeman, 2004a,b ) they included temporal filtering (Appendix 1.1); spatial filtering (Appendix 1.2); the Hilbert transform (Appendix 1.3); measuring frequency and phase with a temporal basis function, the cosine (Appendix 2.1); measuring phase gradient with a spatial basis function, the cone (Appendix 2.2); controls using surrogate data, and channel randomization, and shuffling (Appendix 2.3 and Methods 2.6). The Hilbert transform required data preprocessing in 6 steps: (i) selecting 10 s blocks by visual editing free of artifacts and seizures; (ii) subtracting channel means to remove channel off-sets; (iii) normalization of the entire selected data set to unit standard deviation; (iv) computing temporal power spectral density (PSD T ) and spatial power spectral density (PSD X ) to find optimal filter settings ( Fig. A2 in Appendix A ); (v) spatial low pass filtering adapted to the spatial sampling of the grid with the cut-off at 0.2 c/mm ( Fig. A2 , B) at the concave upward inflection of the PSD X ; and (vi) bandpass temporal filtering at 12–30, 25–50 or 12–55 Hz ( Fig. A2 , A) to extract the beta or low gamma range or both. The temporal PSD T was an average of the 64 PSD T from 1000 data points (5 s) in each of the simultaneously recorded time series. The spatial PSD X was the average of 1000 PSD X over the 64 amplitudes at each time point. All PSD were plotted in log–log coordinates in order to display the full spectral range of power; conventional displays did not allow visualization of the 1/ f power-law distributions of EEG power or the deviations contributed by peaks in the empirical frequency ranges: theta, alpha, beta and gamma. 2.3 Methods for identification of phase cones qualifying as stable The phase cone has a very simple structure of a wave front that radiates outwardly from a point, like a wave when a pebble falls in water, or inwardly toward the point as in implosion. The sets of locations with equal values of phase (isophase contours) form concentric circles around the point, and the phase differences at right angles to the circles give the phase gradient. The equations used to fit a cone in 2D to the 8×8 phase surface at each time step have been described in detail (Appendix A2.2 in Freeman, 2004b , with definition of parameters and state variables in Table 1.1 in Freeman, 2004a ). Convergence of the equation for the cone to a solution by nonlinear regression was achieved in >90–95% of time frames, as well as in many randomized controls (Appendix A2.3 in Freeman, 2004b ), which illustrated that a phase cone could be fitted though with high residuals to many sets of random numbers, giving the appearance of structure where none might exist, especially where it was imposed by spatial filtering. In order to exclude spurious phase structures from the analysis, 5 empirical computational criteria (Methods 2.5, Freeman, 2004b ) were applied to the 64 phase values from the Hilbert method, p j ( t ), j =1,64: (i) the locations of successive apices could not differ by more than the interelectrode distance (1.25 mm), which determined the lower limit on temporal resolution of the array; (ii) the sign of the phase gradient of the cone, +(‘implosion’) or −(‘explosion’), could not change; (iii) the SD x of the analytic phase, AP, could not exceed 0.5 rad ( Fig. 1 C); (iv) the SD x of the analytic phase differences at each time step could not exceed 0.1 rad ( Fig. 1 D), equivalent to 32 Hz in the 12–30 Hz pass band; and (v) the residuals from fitting a cone to the phase surface could not exceed 30% (Appendix 2.2, Freeman, 2004b ). Cones that met all of these constraints qualified as being stable. The criteria for stability of phase cones for the phase values from the Fourier method differed, in that (iii) and (iv) were replaced by the requirement that the residuals on the preliminary step of fitting a cosine to the filtered EEG (Eq. (A2.1) in Appendix A2.1, Freeman, 2004b ) not exceed 20%. 2.4 Statistical summaries of the parameters of stable phase cones Statistical summaries were computed of the data of the main parameters from the spatial and temporal wavelengths of qualifying cones (1) Temporal wavelength, W t = 1 / k ∑ 1000 / ( 2 π f j ) , j = 1 , … , k , (2) Spatial length, W x = 1 / k ∑ 1 / | γ j | , mm / rad, j = 1 , … , k , where W t and W x were averages across the set of k values from the measurements across the duration of a stable cone, γ k was the average gradient, and ω k =1/ W f was the average frequency in rad/s from f k /2π. The distributions of the parameters, W t and W x , being skewed, the log transform was used to give approximately normal distributions for the purpose of computing SD and confidence intervals. W t and W x were used to calculate physiological parameters of phase cones (3) Phase velocity, β = W x / W t m / s, (4) Diameter, D x = ( π / 2 ) W x mm . The frequencies, ω φ ( t ) and f 1 ( t ), were similarly averaged to get ω k and f k for each stable cone. These equations gave the parameters derived from W x and W t that were needed for cross-species comparisons and for anatomical and physiological validation of these empirical parameter ranges by calculation of the distances of the apices from the center of the array, the phase velocities, and the half-power diameters by Eqs. (3) and (4) : for each cone the location of the apex had to be less than one half the width of the array from its closest edge; the phase velocity had to be within the range of conduction velocities of cortical axons (1–10 m/s); the duration had to exceed two digitizing steps; and the half-power diameter could not exceed the width of the cerebrum (20 cm). Use of the 5 constraints gave parameter values that did not exceed these limits. 3 Results 3.1 Identification and measurement of the temporal structure of the analytic phase The 64 EEGs in the subject awake and at rest with no deliberate stimulation had waveforms that were similar though not identical by visual inspection ( Fig. A1 in Appendix A ) and by pair-wise cross-correlation. The aperiodic waveforms persisted indefinitely through episodes of movement artifact and apparent seizures without distinguishing changes in amplitude or bursts of periodic oscillation. This lack of apparent structure was reflected in PSD T of the EEG ( Fig. A2 , A in Appendix A ) and of cospectra of the cross-correlation of the EEG with CAPD ( Fig. 1 A), which were dominated by power in the low end, and by noise in the high end and without consistent or reproducible peaks in the classic ranges. The middle range that included the beta and low gamma frequencies often conformed to the power-law relation, 1/ f α , where α ∼2 on average. The shared waveform showed local differences in amplitude across the microgrid. The differences in amplitude and phase among channels demonstrated that the shared waveform was intrinsic to the dynamics and could not be ascribed solely to activity at the reference electrode or to volume conduction ( Freeman et al., 2003a,b ). The deviations in analytic phase from zero from the individual channels in polar coordinates were functionally equivalent to the imaginary components in Cartesian coordinates used by Nolte et al. (2004) to identify ‘true’ interactions. The spatial PSD X was S-shaped ( Fig. A2 , B in Appendix A ). The upper plateau reflected the commonality of the shared waveform; it was exaggerated by padding with zeroes prior to taking the FFT (Appendix 1.2, Freeman, 2004a ). The differences in amplitude produced power in the middle spatial frequency range of the PSD X (0.1–0.2 c/mm, Fig. A2 , B), which showed nearly linear decrease in log power with increasing log frequency (1/ f α , where α ∼2 on average in the awake state). The flattened tail of low power at high spatial frequencies showed how small the contributions were from individual electrodes. Low pass spatial filtering at 0.2 c/mm (twice the Nyquist frequency, 0.4 c/mm) removed that power as noise. These several features showed that the EEG from the microgrid, despite its small size, displayed a textured field of electric potential, not simply volume-conducted from some remote source or contributed solely by activity at the referential lead. The Hilbert transform revealed otherwise inaccessible structure in the 64 EEG signals, most directly through differences in the unwrapped analytic phase, p j ( t ). Each difference, Δ p j ( t ), in radians divided by the digitizing step, Δ t , in s gave the analytic frequency, ω j ( t ), in rad/s, which on division by 2π gave Hz. The analytic phase differences, Δ p j ( t ), displayed in a 1×64 raster plot ( Fig. 1 B) revealed frames, in which the nearly constant phase differences corresponded to the peak frequency in the pass band of the filtered EEG. The jumps (up to 4-fold increase in instantaneous frequency) corresponded to forward motion of a point around a circle (phase lead); the dips (decrease in instantaneous frequency sometimes to negative values) corresponded to retrograde motion (phase lag). The abrupt changes in the rate of change in phase that bracketed the plateaus occurred not quite simultaneously on all of the channels, again showing that these were textured fields despite the bland appearance of the EEGs. The variation in direction and magnitude of the coordinated analytic phase differences (CAPD) on individual channels gave high values of spatial standard deviation (SD x ) of Δ p j ( t ) at the times of dips or jumps. The high values of SD x served as time markers to bracket the plateaus of CAPD ( Fig. 1 D). Records of the CAPD in eyes-open segments lasting 0.4–2.0 s showed that phase resetting related to that found by Makeig et al. (2002) and by Nikolaev et al. (2005) recurred at rates in the theta range (3–7 Hz). Confirmation was by cross-correlating CAPD with the unfiltered average of the 64 EEG signals, computing the PSD T of the cross-correlation function, and finding a peak in the alpha or theta band of the cospectrum ( Fig. 1 A). Commonly shortly after the onset of a plateau in human CAPD, a large increase in amplitude occurred in the plateau before the next jump or dip in the SD X ( Fig. 2 ), as had been found in rabbit data ( Freeman, 2004a,b ), most clearly in the beta band. This strong negative correlation between the CAPD and the analytic amplitude provided further evidence that CAPD were not computational artifacts. The temporal pass band required for use of the Hilbert transform strongly shaped the CAPD, which appeared at different times in signals from the beta (12–30 Hz) and gamma (25–55 Hz) pass bands ( Fig. A3 ). The CAPD containing both bands (C, 12–50 Hz) gave a pattern that was not a sum of the patterns in the narrower frequency ranges; superposition did not hold for these nonlinear phenomena. Randomization of the EEG by any of 3 methods (Appendix 2.3 in Freeman, 2004b ) reduced or abolished the CAPD and the correlation with fluctuations in analytic amplitude. These results confirmed the existence of observable nonlinear dynamic structure in the background EEG and the dependence of results on the choice of the temporal pass band. 3.2 Identification and measurement of the spatial gradients of phase with the Hilbert method The set of 64 phase values at each digitizing point were obtained with the 4-quadrant arctangent function ( Fig. 1 C), which gave half as many break points in the sawtooth analytic phase as did the 2-quadrant function ( Fig. 1 D). The 64 phase values at each digitizing step were fitted with a cone. Four parameters were optimized by nonlinear regression with the criterion of least squares residuals: the x , y location of the apex, the height or depth of the apex from the plane of fitting, Φ o ( t ), and the slope of the cone, γ φ ( t ), including its sign. The apices of the majority of cones fell within the borders of the array, the minority outside. There was no evidence of geometric patterns of location of multiple apices, nor were there preferential distributions of apices having phase lead (+) vs. those having phase lag (−). Small clumps and short strings of apices reflected low spatial variation in phase that accompanied the low temporal variation implying stationarity. These successive apices were closer to each other than the interelectrode interval (1.25 mm), which determined the lower limit on spatial resolution. Intervening spatial steps taken by the apices in the breaks between the plateaus were often longer than the width of the array. This evidence supported the inference that the shift in the location of the apex was discontinuous from each plateau to the next one. The analytic phase differences at individual channels were fixed by the difference between two successive cones with widely separated apices and often with opposite polarity; this explained the high SD X of CAPD. 3.3 Parameters of stable phase cones in the beta and gamma ranges by the Hilbert method The two main parameters by direct measurement were the temporal phase gradient (giving the analytic frequency, ω k in rad/s) and the spatial phase gradient, γ k in rad/mm, averaged over the values from k fitted cones in each frame. Distributions were compiled for cones in 50 s of EEG recording giving 10,000 digitizing steps. Frequencies were converted by Eq. (1) to temporal wavelength, W t , in ms/rad. The distributions of W t were skewed to large numbers of short values (high frequencies) ( Table 1 ). The phase gradients were converted by Eq. (2) to spatial wavelength, W x in mm/rad. The values of W x were strongly skewed to short wave lengths (high spatial frequencies). The distributions of log ω k and of log| γ k | were nearly normal. The wavelengths of the cones gave the basis to estimate the phase velocities by Eq. (3) and the half-power diameters of phase cones by Eq. (4) ( Table 2 ). Both measures had high coefficients of variation, 45–75%. The estimates of phase velocity fell within the range of conduction velocities of cortical axons running parallel to the pial surfaces (∼1–10 m/s, reviewed in Freeman and Barrie, 2000 ). The x – y coordinates of the phase cones gave estimates of the distances of the apices from the center of the array. The mean distance was close to the distance from the center to the edge (5 mm); this value was consistent with the higher density of apices inside the array and the larger area but lower density of apices that could be located outside the array. The duration ( Table 2 ) was estimated from the number of steps, k , across, which the criteria were met. 3.4 Parameters of phase cones with the Fourier method Application of the Fourier method began not with the FFT but with fitting the 64 EEG signals in a stepped window with the sum of two cosines (Appendix 2.1, Freeman, 2004b ) after low pass spatial filtering and bandpass filtering in the beta or gamma range. Convergence was obtained routinely with residuals that ranged widely from <5% to >90% despite the coarse grain imposed by the 5 ms digitizing step and the brevity of the window. An empirical threshold of 20% residuals was adopted for acceptance of the 8×8 matrices of phase values from the dominant fitted cosine; the reliability of fitting cosines in 1D was higher than that for fitting a cone in 2D. The graphic displays of phase surfaces, fitted cones and residuals closely resembled those from the Hilbert method. The means and SD of the frequencies, f 1 , phase gradients, and wavelengths are listed in Table 3 for the beta and gamma ranges. The distributions of frequencies were skewed to lower values, resembling the 1/ f α form of PSD T of the same EEG data. The temporal wavelengths, W t , were skewed across the range set by the temporal pass band as in the Hilbert method. The distributions of phase gradients were bimodal. The forms of the distributions of the diameters and phase velocities as well as estimates of the means did not significantly differ from those by the Hilbert method ( Table 4 compared with Table 2 ), although the variances were smaller owing to fewer outliers. The locations of the apices and the sequences of distances between them were not identical but were statistically indistinguishable for the two methods applied to the same data. These comparisons showed that the Hilbert and Fourier methods gave essentially the same cone parameters except those relating to cone duration ( Tables 2 and 4 ). The lower limit on duration by the Fourier method was set by the requirement that the window length include at least one cycle of the frequency at which the phase was defined, and an upper limit appeared to coincide with length of frames between CAPD. Stable phase cones occurred with least % residuals in these frames ( Fig. A3 , Appendix A ) but not exclusively and most often overlapping with up to 5 or 6 persisting cones having earlier onset. A very important finding was that the estimates of the mean duration varied markedly with the width of the window used to measure the frequency at which to calculate the phase in the Fourier method. This dependence carried over to the extremely short durations estimated by the Hilbert method. The distributions of values were judged to be power-law by the following criteria: (a) maximal counts were at minimal durations, (b) the log counts decreased linearly with increasing log cone duration in a power-law relation ( Fig. 3 ), (c) the SD tended to equal the mean, and (d) the values taken by the mean and SD increased with increasing window of measurement. These properties suggested that the underlying dynamics was fractal with self-similarity of cones over broad ranges of diameters and durations. The power-law scaling resembles that reported in human EEG by Hwa and Ferree (2002); King (1991); Linkenkaer-Hansen et al. (2001) . The power-law nature of the skewed distributions raised the critical concern whether the larger numbers of small phase cones with high residuals formed a significant tail of the distributions or accumulated simply as the result of fitting the cone to noise. This was investigated by comparing distributions with and without the restriction that residuals of cone fitting not exceed 30%. The results showed that imposing the restriction decreased the numbers of qualifying cones by 50–80%. The same forms and parameters held above the threshold for measurement determined by the window duration, regardless of the reduction in sample size, when the 30% criterion was imposed ( Fig. 3 ). The power-law distributions suggested that many if not most of the overlapping cones with high percent residuals formed complex structure that appeared to be ‘noise’ but might in fact manifest a controlled state of cortex. Inclusion of the cones with high percent residuals had no effect on mean frequency, but it increased mean phase gradients. The increase suggested that the cones removed were more likely to be relatively flat, indicating high phase velocity and large diameter by Eq. (4) . Velocities in excess of 10 m/s from flat cones were thought likely to be spurious and attributed to fitting a cone to noise. However, some of the phase cones that were removed by this criterion might have represented valid physiological structure. Comparison of the present data to those previously obtained from rabbit experiments demonstrated no species differences in frequency, temporal wavelength or duration other than those relating to the temporal pass band, the window duration, and interelectrode spacing ( Table 5 ). The phase gradient differed 20–60% between rabbit and human, and these differences persisted through estimates of the spatial wavelength, phase velocity, half-power cone diameter, and apical distance from the array center. The estimates of phase gradient, γ k , differed by the ratio between 1.2 and 1.6, while the ratio of interelectrode distances was 1.6. 3.5 Measurement of phase during slow wave sleep (SWS) The EEG during SWS was dominated by activity in the low theta and delta ranges. Despite this spectral difference from the awake state the statistical properties of spatial and temporal wavelengths and the derived measures of phase cones did not differ significantly from those found in the subject when awake ( Tables 3 and 4 ). Gamma cones virtually disappeared, while beta cones persisted at lower incidence and with decreased phase velocity and diameter. The spatial clusters of apices by the Hilbert and Fourier methods were similar in both states for both sign and location. The spatial PSD X was unchanged with sleep (awake in Fig. A2 , B). Significant differences were found between the temporal PSD T after the transition from the awake state to the SWS state ( Fig. 4 A). The local spectral peaks almost disappeared, so that a straight line fitted to the PSD T gave low percent residuals of 3.51±0.92%, of the total variance in SWS (A), compared with 5.67±1.09 on awakening when spectral peaks reappeared. The slope in SWS varied seemingly at random about an average of α =3.19±0.31 compared with α =2.39±0.27 when the subject was awake (B). The intercept varied inversely with slope (C), 2.17±0.51 in sleep vs. 1.48±0.46 when awake. Strikingly, the temporal behavior of the CAPD disclosed long-duration (about a second) plateaus of phase stabilization during SWS ( Fig. 5 A, gray curve). These plateaus had no qualifying phase cones; the few cones that did appear were excluded by the criteria that their phase velocities exceeded the physiological range and their percent residuals were too high, even though the variance was low. Examples of CAPD in 10 s segments from awake and sleep states are compared in Appendix A . Whereas during most records in SWS the analytic amplitude had low values, during the 1 s plateaus the analytic amplitude rose slowly to moderate values and fell as the plateaus ended ( Fig. 5 A and B, black curves). Contrastingly in the waking state the analytic amplitude rose rapidly to high values during brief plateaus following onsets of low SD X and fell rapidly just before spikes in SD X ( Fig. 5 C, gray curves; see Fig. 2 for higher temporal resolution). The spatial variance of amplitude remained low during the 1 s plateaus ( Fig. 5 B, gray curve), whereas the variance fluctuated in correlation with the mean during the brief plateaus of phase stability in the awake state ( Fig. 5 D, gray vs. black curves). These findings implied that the EEG was virtually devoid of spatiotemporal structure during the 1 s plateaus, even though the analytic amplitude rose for exceptionally long durations. In summary, during sleep the structure of phase persisted virtually unchanged, except during the deepest NREM sleep when the PSD T approached a strict power-law form with an exponent near −3. Then flat phase epochs appeared that lasted up to a second and at intervals of varying by 4–10+ s. These epochs appeared to be devoid of spatial and temporal structure in the beta and gamma ranges. 4 Discussion The most important finding in this study is the way in which high spatial and temporal resolution opens a new domain of fine structure to exploration of the background EEG. This pervasive and robust electrical activity has for several decades been regarded as noise ( Freeman, 1975/2004 ) that is to be removed by event-related time averaging so as to establish flat pre-event baselines. The Hilbert transform serves to decompose multichannel EEGs from a high-density array into independent time series of amplitude and phase, which together give a view of the background as a dynamically ordered state that combines stability with the capacity for rapid adaptation in any direction that unpredicted changes in the environment may require. An outstanding characteristic of the power spectra and the measures of phase is their skewed distributions of power in both temporal and spatial dimensions, which indicate self-similarity across broad scales of measurement and the nonexistence of normative mean and minimal values. The search for lower bounds on the durations of phase cones was pursued to the lower limit of the duration of the digitizing step; the search for minimum diameters was limited by the point spread functions of the local fields of potential of cortical dendrites that precluded finding diameters less than 2 mm. What these findings indicate is that neocortex continually generates local patterns by phase transitions and just as rapidly quenches them. The phase patterns are conic, owing to the finite velocity of axons that carry the pulses required to communicate the phase transitions by which AM patterns form; communication is not instantaneous and truly ‘zero lag’. These phase cones suggest that the conditional stability of neocortex is analogous to that of a sand pile with continuous input at its apex that maintains a critical angle of repose by repeated avalanches ( Freeman, 2004b ) having power-law distributions of size, duration, and recurrence rates. This phenomenon was described as ‘self-organized criticality’ by Bak (1996); Jensen (1998) and has been proposed for neocortex by Beggs and Plenz (2004); Linkenkaer-Hansen et al. (2001) . A comparable analogy is a heated pan of boiling water in which the bubbles keep water at its boiling point by releasing heat in steam. Yet another is fog, in which water molecules continually condense into droplets that evaporate and are replaced, with occasional formation of large droplets that fall as rain. The critical parameter in cortex that is analogous to an angle of repose or a critical temperature has been proposed ( Freeman, 2004a, in press-a ) to be the firing rates of individual neurons that are everywhere homeostatically limited by refractory periods (see also Cirelli et al. (2005) on homeostatic control of slow waves in sleep). The background activity of cortex is conceived to manifest the approach by the neocortex to its critical state on displacement by constant bombardment from cortical input, because it disappears on deafferentation. Prigogine (1980) studied the stability of these kinds of system in terms of local fluctuations, most of which are quenched, but a selected few of which carry a complex system from one state to another in bifurcations or phase transitions. Ricciardi and Umezawa (1967) proposed that neocortex maintains a metastable state in which such transitions occur by spontaneous breaking of symmetry among an indefinitely large collection of ground states ( Freeman and Vitiello, 2005; Vitiello, 2001 ). Bressler and Kelso (2001); Fingelkurts and Fingelkurts (2004); Kelso (1995) conceive metastability as a fluid condition of brain dynamics without convergence to attractors in which the parts retain autonomy yet coordinate with each other in respect to behavior. Tsuda (2001) and his colleagues view metastability in terms of a sequence of transitions among ordered states that is mapped by a trajectory in state space in a dynamic they call ‘chaotic itinerancy’. In this report conditional stability is described in terms of rapidly adapting attractor landscapes with selection by input of one attractor at each phase transition that resolves an uncertainty posed by the a priori availability of multiple basins of attraction. The similarity of rabbit and human neocortex to each other gives reason to suppose that these general principles of self-organization and conditional stability are fundamental properties of cortical dynamics that are manifested in the fine phase structure of the EEG. The only species differences found at the level of fine structure were commensurate with the tools needed to measure parameters having power-law distributions with the largest number of events having the smallest dimensions. The tools included the limiting digitizing step and interelectrode interval, which had been designed in respect to brain size, and which, it appears, helped shape the observed values. An unexpected observation in this study was the phase pattern in SWS that exhibited plateaus lasting about 1 s that had no qualifying phase cones, yet sustained unpatterned beta–gamma activity as measured by analytic amplitude and its SD. This state suggests 3 hypotheses, one of which is in the context of studies demonstrating that cortical excitability is significantly modulated by large-scale activity at slowest frequencies ( Amzica, 2002; Amzica and Steriade, 2000; Massimini et al., 2003; Vanhatalo et al., 2004 ). It appears that human neocortex maintains a robust state of self-organized criticality in all stages of waking and at least more superficial sleeping (non-SWS), as manifested in the structure of the spatial patterns of phase. This state is maintained under the impact of input from all sources to the neocortex by repeated small and large phase transitions to bursts of oscillation resembling avalanches ( Beggs and Plenz, 2004; Freeman, 2004b; Tsuda, 2001 ) that give structure to the EEG in the form of spectral peaks, phase cones, and spatial patterns of amplitude modulation. When input is blocked by surgical intervention, structure disappears with emergence of a state of maximal symmetry in the form of a flat EEG ( Burns, 1958; Becker and Freeman, 1968; Gray and Skinner, 1988 ). The persistence of phase structure during non-SWS is consistent with the numerous observations that brains are cognitively active during sleep (e.g. Cirelli et al., 2005; Massimini et al., 2003; Massimini et al., 2004 Steriade, 1997 ). During deep SWS the input to neocortex may be transiently in abeyance, which allows neocortex to settle into its basal ground state of unstructured rest that is accompanied by the classic appearance of delta waves and, in the extreme, to epochs of substantial loss of phase and amplitude microstructure. Tononi's group showed that the extent of spread of cortical responses to transcranial magnetic stimulation was significantly reduced during SWS and more so during REM sleep ( Massimini et al., 2005 ). That is consistent with the idea that the intensity of mutual excitation among pyramidal cells and thereby the distance of long-range cortico-cortical transmission, both pre-requisite for generation of phase structures, may be reduced in sleep (and prior to seizure ( Freeman et al., in press ); see also Van Quyen et al. (2005) ). Their concept of ‘synaptic down-scaling’ is compatible with a widespread decrease in excitation relative to inhibition; a broad distribution of delta activity at high amplitude in scalp EEG is compatible with a decrease in correlation distance and an enhancement by competitive inhibition of fine-structure activity at beta–gamma frequencies. They also showed that slow oscillations in SWS appeared as waves travelling 0.1–7 m/s, corresponding to the range of velocities of axons running parallel to the pia ( Freeman and Barrie, 2000 ). These waves were not shown to be radially symmetric nor to have apices of phase distributions that alternated in polarity, so they were not likely to manifest large phase cones. However, evidence for cones at delta frequencies was not sought with the 1 cm 2 microgrid on the premise that the spatial wavelengths would be too long for detection by the array, so uncertainty remains. Alternatively, these epochs of unpatterned activity might be manifestations of simple partial seizures that were facilitated during sleep and not in the awake state. In these recordings, from the microgrid the slow wave activity in the delta range was prominent even when the subject was engaged in a simple memory task. This was in contrast to the more highly structured EEG activity seen in scalp EEG of normal humans and in intracranial EEG from primary sensory and limbic areas in animals. The poverty of structure in the area of the microgrid might be ascribed to its location in a brain area remote from those engaged in normal behavior, and also to its location in a region of the brain known to be diseased, which was, after all, the reason for the opportunity for recording with an intracranial microgrid. Presumably the parameters derived in this study were influenced in unknown ways by the subject's brain disorder that may have included significant reduction in REM sleep. Nevertheless the database gave useful clues not otherwise available for developing the EEG as a tool to explore the mesoscopic dynamics of human brain, particularly the long-range correlations that depend critically on mutual excitation among pyramidal cells forming nearest-neighbor, small-world ( Watts and Strogatz, 1998 ) and scale-free ( Wang and Chen, 2003 ) networks, and the diminution in correlation range that appears to accompany SWS and precede onset of complex partial seizure ( Freeman et al., in press ). Among questions to be answered in the future are what the maximal size of phase cones might be, how their diameters relate to stability, how the square of analytic amplitude (power) might be related to metabolic demand through hemodynamic imaging, and whether the analytic phase might be used to locate hemisphere-wide AM patterns in scalp EEG. Answers may best come from simultaneous records of scalp and microgrid EEGs. Acknowledgements The human data were collected in the EEG and Clinical Neurophysiology Laboratory, Harborview Medical Center, Seattle WA. The 8×8 electrode array was constructed by Ad-Tech Medical Instrument Corp. Racine WI 53404 in accordance with a Berkeley design. Programming was by Brian C. Burke in the Division of Neurobiology at Berkeley. Partial support was provided by grants NCC 2-1244 from NASA and EIA-0130352 from NSF to Robert Kozma, and by Dr Scott Barnhart, Medical Director, Harborview Medical Center, Seattle WA. Appendix A Time series, power spectral densities and CAPD of intracranial human EEG The 64 raw EEGs had correlated waveforms ( Fig. A1 ) that differed in amplitudes and time lags but otherwise had no discernible structure. The temporal PSD T ( Fig. A2 , A) displayed a range with nearly linear decrease in log power with increasing log frequency (1/ f α , where α ∼2 on average). The bandpass temporal filter required for the Hilbert transform was placed in the beta–gamma range (12–55 Hz) between alpha and 60 Hz. The spatial PSD X ( Fig. A2 , B) also had a middle range with nearly linear fall-off of log power with log frequency at a slope of −2, which had first been described by recording intracranial human EEG from the pial surface with a 1D electrode array in a line at 0.5 mm spacing ( Freeman et al., 2000 ). The flattened tail of the PSD X at high spatial frequencies displayed the low power from contributions localized to each electrode, which from the standpoint of mesoscopic interactions was to be treated as noise and removed by low pass spatial filtering. The analytic phase, p j ( t ), was unwrapped to show the coordinated analytic phase differences, CAPD, Δ p j ( t ), in a 1×64 raster plot ( Fig. A3 ). The mean instantaneous frequency, ω j ( t )=Δ p j ( t )/Δ t , in the frames between spikes and dips corresponded to the peak frequency in the pass band of the filtered EEG. The dips and spikes in the phase differences that bracketed the frames occurred not quite simultaneously on all of the channels. The directions and magnitudes varied, giving high spatial standard deviations (SD x ) of Δ p j ( t ) at the times of dips or jumps. Peaks of SD x appeared at different times after filtering in the beta (A, 12–30 Hz) and gamma (B, 25–55 Hz) pass bands. The CAPD containing both bands (C, 12–50 Hz) showed expectedly ( Freeman et al., 2003a,b ) a pattern that was not a sum of the patterns in the narrower frequency ranges. Shuffling of the EEG signals on each channel (Appendix 2.3 in Freeman, 2004b ) abolished the CAPD ( Fig. A3, D ). References Amzica, 2002 F. Amzica In vivo electrophysiological evidences for cortical neuron-glia interactions during slow (<1 Hz) and paroxysmal sleep oscillations J Physiol (London) 552 2002 325 332 Amzica and Steriade, 2000 F. Amzica M. Steriade Neuronal and glial membrane potentials during sleep and paroxysmal oscillations in the neocortex J Neurosci 20 2000 6648 6665 Bak, 1996 P. Bak How nature works: the science of self-organized criticality 1996 Copernicus New York Barlow, 1993 J.S. Barlow The electroencephalogram: its patterns and origins 1993 MIT Press Cambridge, MA Becker and Freeman, 1968 C.J. Becker W.J. Freeman Prepyriform electrical activity after loss of peripheral or central input or both Physiol Behav 3 1968 597 599 Beggs and Plenz, 2004 J.M. Beggs D. Plenz Neuronal avalanches are diverse and precise activity patterns that are stable for many hours in cortical slice cultures J Neurosci 24 22 2004 5216 5229 Breakspear and Friston, 2001 M. Breakspear K. Friston Symmetries and itinerancy in nonlinear systems with many degrees of freedom Behav Brain Sci 24 2001 813 814 Bressler and Kelso, 2001 S.L. Bressler J.A.S. Kelso Cortical coordination dynamics and cognition Trends Cogn Sci 5 2001 26 36 Bressler et al., 1993 S.L. Bressler R. Coppola R. Nakamura Episodic multiregional cortical coherence at multiple frequencies during visual task performance Nature 366 1993 153 156 Bruns, 2004 A. Bruns Fourier-, Hilbert-, and wavelet-based signal analysis: are they really different approaches? J Neurosci Methods 137 2004 321 332 Burns, 1958 B.D. Burns The mammalian cerebral cortex 1958 Arnold London Chapman et al., 2002 C.L. Chapman P.D. Bourke J.J. Wright Spatial eigenmodes and synchronous oscillation: coincidence detection in simulated cerebral cortex J Math Biol 45 2002 57 78 Cirelli et al., 2005 C. Cirelli R. Huber A. Gopalakrishnan T.L. Southard G. Tononi Locus coeruleus control of slow-wave homeostasis J Neurosci 2005 4503 4511 Engel et al., 2001 A.K. Engel P. Fries W. Singer Dynamic predictions: oscillations and synchrony in top–down processing Nat Neurosci Rev 2 2001 704 716 Fingelkurts and Fingelkurts, 2004 A.A. Fingelkurts A.A. Fingelkurts Making complexity simpler: multivariability and metastability in the brain Int J Neurosci 114 2004 843 862 Freeman, 1975/2004 W.J. Freeman Mass action in the nervous system 1975/2004 New York Academic Press http://sulcus.berkeley.edu/MANSWWW/MANSWWW.html Freeman, 2003 W.J. Freeman A neurobiological theory of meaning in perception. Part 2. Spatial patterns of phase in gamma EEG from primary sensory cortices reveal the properties of mesoscopic wave packets Int J Bifurcat Chaos 13 2003 2513 2535 Freeman, 2004a W.J. Freeman Origin, structure, and role of background EEG activity, part 1. Analytic phase Clin Neurophysiol 115 2004 2077 2088 Freeman, 2004b W.J. Freeman Origin, structure, and role of background EEG activity, part 2. Analytic amplitude Clin Neurophysiol 115 2004 2089 2107 Freeman, 2005a W.J. Freeman Origin, structure, and role of background EEG activity. Part 3. Neural frame classification Clin Neurophysiol 116 5 2005 1118 1129 Freeman, 2005b W.J. Freeman A field-theoretic approach to understanding scale-free neocortical dynamics Biol Cybern 92 6 2005 350 359 Freeman, in press-a Freeman WJ. Origin, structure, and role of background EEG activity. Part 4. Neural frame simulation. Clin Neurophysiol; in press-a. Freeman, in press-b Freeman WJ. A cinematographic hypothesis of cortical dynamics in perception. In: Karakas S, Basar E, editors. Int J Psychophysiol; in press-b. Freeman and Barrie, 2000 W.J. Freeman J.M. Barrie Analysis of spatial patterns of phase in neocortical gamma EEGs in rabbit J Neurophysiol 84 2000 1266 1278 Freeman and Rogers, 2002 Freeman WJ, Rogers LJ. Fine temporal resolution of analytic phase reveals episodic synchronization by state transitions in gamma EEG. J Neurophysiol 2002;87:937–45. Freeman and Rogers, 2003 W.J. Freeman L.J. Rogers A neurobiological theory of meaning in perception, part 5. Multicortical patterns of phase modulation in gamma EEG Int J Bifurcat Chaos 13 2003 2845 2856 Freeman and Vitiello, 2005 Freeman WJ, Vitiello G. Nonlinear brain dynamics and many-body field dynamics. Electromagn Biol Med 2005. http://arxiv.org/q-bio.NC/0507014 . Freeman et al., 2000 W.J. Freeman L.J. Rogers M.D. Holmes D.L. Silbergeld Spatial spectral analysis of human electrocorticograms including the alpha and gamma bands J Neurosci Methods 95 2000 111 121 Freeman et al., 2003a W.J. Freeman B.C. Burke M.D. Holmes Aperiodic phase re-setting in scalp EEG of beta–gamma oscillations by phase transitions at alpha–theta rates Hum Brain Mapp 19 2003 248 272 Freeman et al., 2003b W.J. Freeman B.C. Burke M.D. Holmes S. Vanhatalo Spatial spectra of scalp EEG and EMG from awake humans Clin Neurophysiol 16 2003 1055 1060 Freeman et al., in press Freeman WJ, Holmes MD, West GA, Vanhatalo S. Dynamics of human neocortex that optimize its stability and flexibility. J Intell Syst; in press. Friston, 2000 K.J. Friston The labile brain. I. Neuronal transients and nonlinear coupling Philos Trans R Soc Lond B Biol Sci 355 2000 215 236 Gray and Skinner, 1988 C.M. Gray J.E. Skinner Centrifugal regulation of neuronal activity in the olfactory bulb of the waking rabbit as revealed by reversible cryogenic blockade Exp Brain Res 69 1988 378 386 Grinvald et al., 2003 A. Grinvald A. Arieli M. Tsodyks T. Kenet Neuronal assemblies: single cortical neurons are obedient members of a huge orchestra Biopolymers 68 2003 422 436 Hwa and Ferree, 2002 R.C. Hwa T. Ferree Scaling properties of fluctuations in the human electroencephalogram Phys Rev E66 2002 021901 Jensen, 1998 H.J. Jensen Self-organized criticality: emergent complex behavior in physical and biological systems 1998 Cambridge University Press New York Kelso, 1995 J.A.S. Kelso Dynamic patterns: the self organization of brain and behavior 1995 MIT Press Cambridge, MA King, 1991 C.C. King Fractal and chaotic dynamics in nervous systems Prog Neurobiol 36 1991 279 308 Kozma and Freeman, 2002 R. Kozma W.J. Freeman Classification of EEG patterns using nonlinear dynamics and identifying chaotic phase transitions Neurocomputing 44 2002 1107 1112 Kozma et al., 2005 R. Kozma M. Puljic P. Balister B. Bollabás W.J. Freeman Phase transitions in the neuropercolation model of neural populations with mixed local and non-local interactions Biol Cybern 92 2005 367 379 Le Van Quyen et al., 2001 M. Le Van Quyen J. Foucher J-P. Lachaux E. Rodriguez A. Lutz J. Martinerie F. Varela Comparison of Hilbert transform and wavelet methods for the analysis of neuronal synchrony J Neurosci Methods 111 2001 83 98 Linkenkaer-Hansen et al., 2001 K. Linkenkaer-Hansen V.M. Nikouline J.M. Palva R.J. Iimoniemi Long-range temporal correlations and scaling behavior in human brain oscillations J Neurosci 15 2001 1370 1377 Makeig et al., 2002 S. Makeig M. Westerfield T.-P. Jung S. Enghoff J. Townsend E. Courchesne T.J. Sejnowski Dynamic brain sources of visual evoked responses Science 295 2002 690 694 Mandelbrot, 1983 B.B. Mandelbrot The fractal geometry of nature 1983 WH Freeman New York Massimini et al., 2003 M. Massimini M. Rosanova M. Mariotti EEG slow (approximately 1 Hz) waves are associated with nonstationarity of thalamo-cortical sensory processing in the sleeping human J Neurophysiol 89 2003 1205 1213 Massimini et al., 2004 M. Massimini R. Huber F. Ferraretti S. Hill G. Tononi The slow sleep oscillation as a traveling wave J Neurosci 24 2004 686206870 Massimini et al., 2005 M. Massimini F. Ferraretti R. Huber S.K. Esser H. Singh G. Tononi Breakdown of cortical effective connectivity during sleep Science 309 5744 2005 2228 2232 Nikolaev et al., 2005 A.R. Nikolaev P. Gong C. van Leeuwen Evoked phase synchronization between adjacent high-density electrodes in human scalp EEG: duration and time course related to behavior Clin Neurophysiol 116 2005 2005 2403 2419 Nolte et al., 2004 G. Nolte O. Bai L. Wheaton Z. Mari S. Vorbach M. Hallett Identifying true brain interaction from EEG data using the imaginary part of coherency Clin Neurophysiol 115 2004 2292 2307 Palva et al., 2005 J.M. Palva S. Palva K. Kaila Phase synchrony among neuronal oscillations in the human cortex J Neurosci 25 15 2005 3962 3972 Pereda et al., 1998 E. Pereda A. Gamundi R. Rial J. Gonzalez Non-linear behavior of human EEG—fractal exponent versus correlation dimension in awake and sleep stages Neurosci Lett 250 1998 91 94 Pikovsky et al., 2001 A. Pikovsky M. Rosenblum J. Kurths Synchronization—a universal concept in non-linear sciences 2001 Cambridge University Press Cambridge, UK Prigogine, 1980 I. Prigogine From being to becoming: time and complexity in the physical sciences 1980 Freeman San Francisco, CA Quiroga et al., 2002 R.Q. Quiroga A. Kraskov T. Kreuz P. Grassberger Performance of different synchronization measures in real data: a case study on electroencephalographic signals Phys Rev E 6504 2002 U645 U658 [art. no. 041903] Ricciardi and Umezawa, 1967 L.M. Ricciardi H. Umezawa Brain and physics of many-body problems Kybernetik 4 1967 44 48 Rodriguez et al., 1999 E. Rodriguez N. George J.-P. Lachaux J. Martinerie B. Renault F. Varela Perception's shadow: long-distance synchronization of human brain activity Nature 397 1999 430 433 Singer and Gray, 1995 W. Singer C.M. Gray Visual feature integration and the temporal correlation hypothesis Ann Rev Neurosci 18 1995 555 586 Stam et al., 2003 C.J. Stam M. Breakspear A.-M. van Cappellen van Walsum B.W. van Dijk Nonlinear synchronization in EEG and whole-head recordings of healthy subjects Hum Brain Mapp 19 2003 63 78 Steriade, 1997 M. Steriade Synchronized activities in coupled oscillators in the cerebral cortex and thalamus at different levels of vigilance Cereb Cortex 7 1997 583 604 Tallon-Baudry et al. Tallon-Baudry C, Bertrand O, Peronnet F, Pernier J. Induced gamma-band activity during the delay of a visual short-term memory task in humans. J Neurosci 1998;18:4244–4254. Tsuda, 2001 I. Tsuda Towards an interpretation of dynamic neural activity in terms of chaotic dynamical systems Behav Brain Sci 24 2001 793 810 Vanhatalo et al., 2004 S. Vanhatalo J.M. Palva M.D. Holmes J. Voipio K. Kaila Infraslow oscillations modulate excitability and interictal epileptic activity in the human neocortex during sleep Proc Natl Acad Sci USA 101 2004 5053 5057 Van Putten, 2003 M.J.A.M. Van Putten Proposed link rates in the human brain J Neurosci Methods 127 2003 1 10 Van Quyen et al., 2005 M.L. Van Quyen J. Soss V. Navarro R. Robertson M. Chavez M. Baulac J. Martinerie Preictal state identification by synchronization changes in long-term intracranial EEG recordings Clin Neurophysiol 116 2005 559 568 Varela et al., 2001 F. Varela J.-P. Lachaux E. Rodriguez J. Martinerie The brain-web: phase synchronization and large-scale integration Nat Rev Neurosci 2 2001 229 239 Vitiello, 2001 G. Vitiello My double unveiled 2001 John Benjamins Amsterdam Von Stein et al., 2000 A. Von Stein C. Chiang P. König Top–down processing mediated by interareal synchronization Proc Natl Acad Sci USA 97 2000 14748 14753 Wang and Chen, 2003 X.F. Wang G.R. Chen Complex networks: small-world, scale-free and beyond IEEE Circuits Syst Mag 31 2003 6 20 Watts and Strogatz, 1998 D.J. Watts S.H. Strogatz Collective dynamics of ‘small-world’ networks Nature 393 1998 440 442
更多查看译文
关键词
Coordinated analytic phase differences CAPD,Hilbert transform,Human EEG,Phase gradient,Self-organized criticality,Sleep,Phase transition,Synchrony
AI 理解论文
溯源树
样例
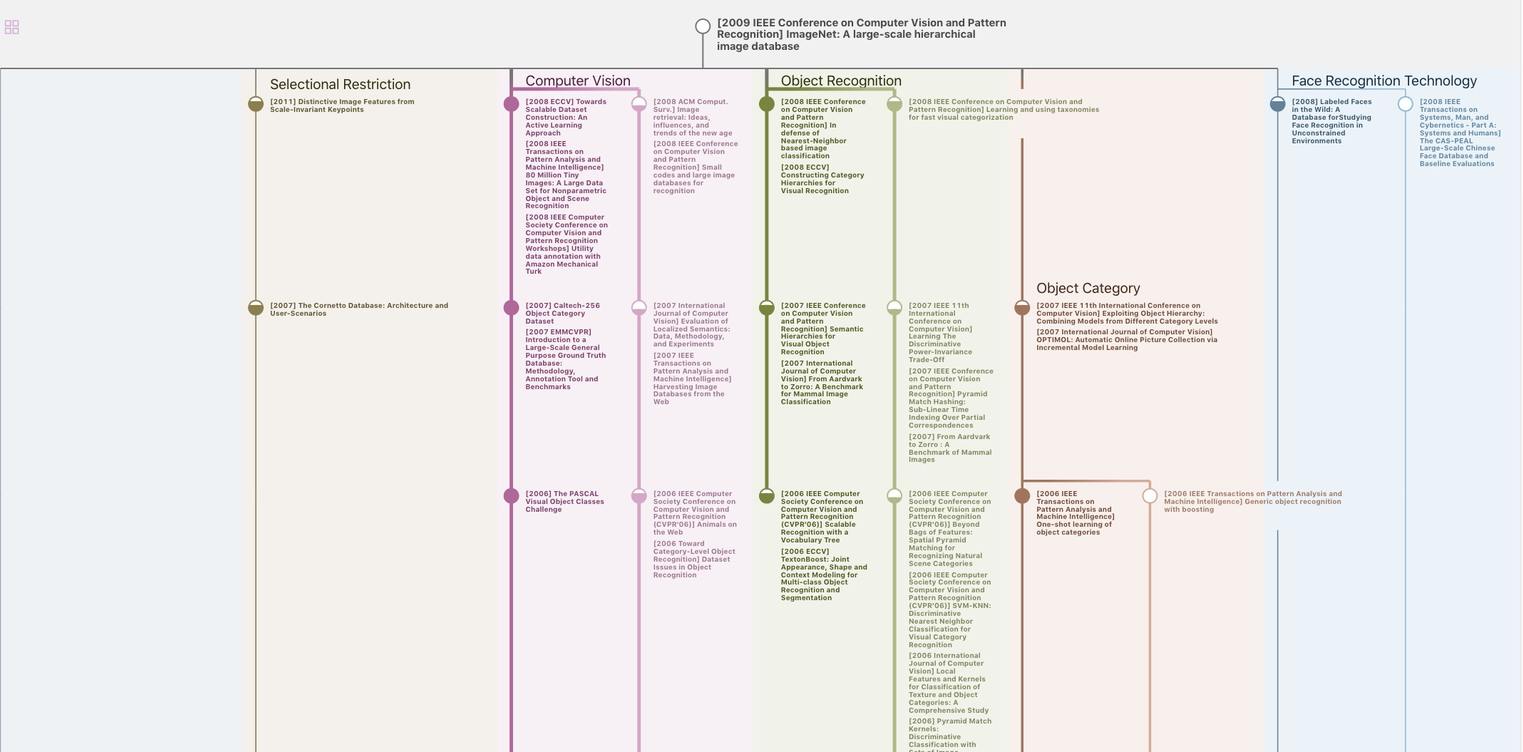
生成溯源树,研究论文发展脉络
Chat Paper
正在生成论文摘要