ILClass: Error-driven antecedent learning for evolving Takagi-Sugeno classification systems.
Applied Soft Computing(2014)
摘要
The purpose of this research work is to go beyond the traditional classification systems in which the set of recognizable categories is predefined at the conception phase and keeps unchanged during its operation. Motivated by the increasing needs of flexible classifiers that can be continuously adapted to cope with dynamic environments, we propose a new evolving classification system and an incremental learning algorithm called ILCIass. The classifier is learned in incremental and lifelong manner and able to learn new classes from few samples. Our approach is based on first-order Takagi-Sugeno (TS) system. The main contribution of this paper consists in proposing a global incremental learning paradigm in which antecedent and consequent are learned in synergy, contrary to the existing approaches where they are learned separately. Output feedback is used in controlled manner to bias antecedent adaptation toward difficult data samples in order to improve system accuracy. Our system is evaluated using different well-known benchmarks, with a special focus on its capacity of learning new classes. (C) 2013 Elsevier B.V. All rights reserved.
更多查看译文
关键词
Evolving fuzzy classifiers,Online learning,Takagi-Sugeno,Classification
AI 理解论文
溯源树
样例
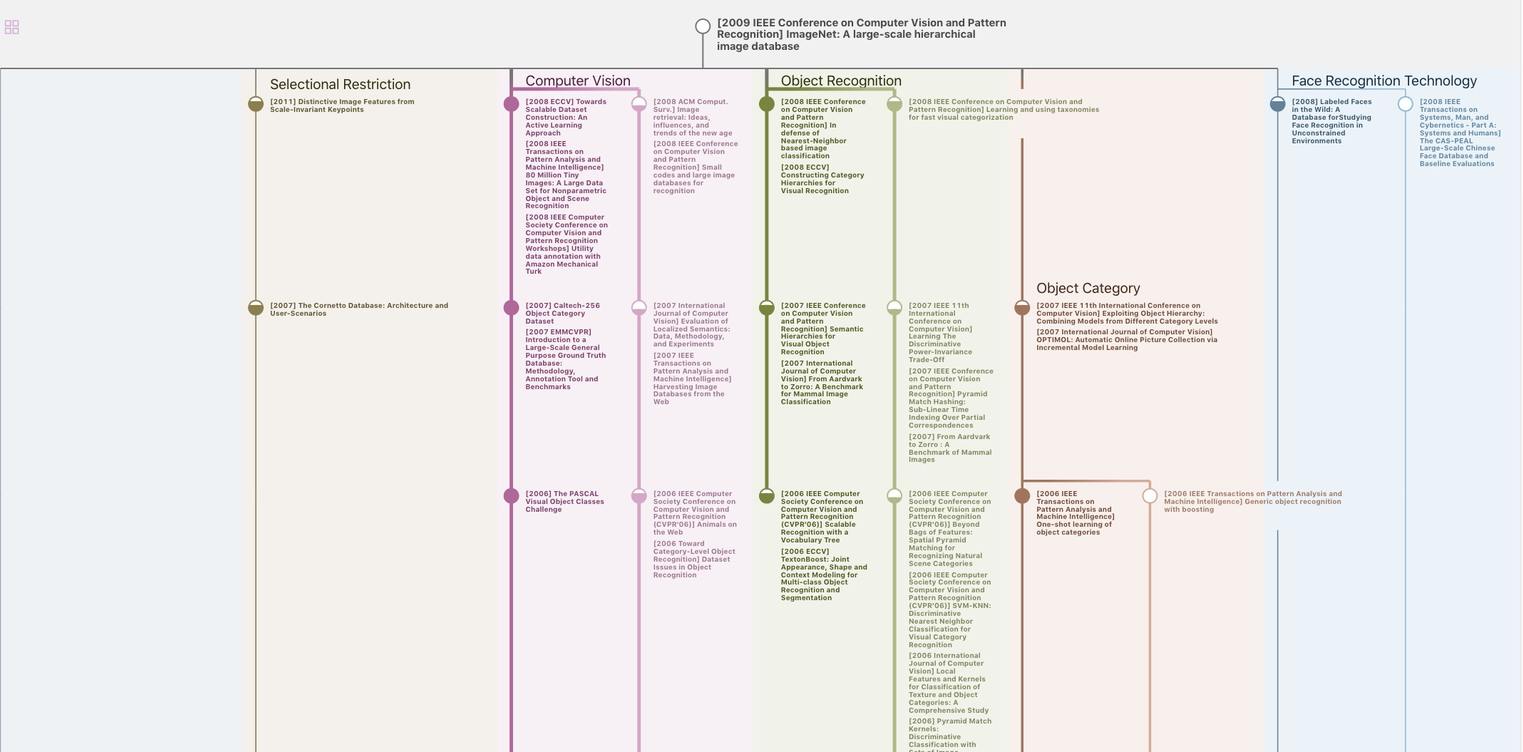
生成溯源树,研究论文发展脉络
Chat Paper
正在生成论文摘要