An Empirical Bayes Approach To Contextual Region Classification
CVPR: 2009 IEEE CONFERENCE ON COMPUTER VISION AND PATTERN RECOGNITION, VOLS 1-4(2009)
摘要
This paper presents a nonparametric approach to labeling of local image regions that is inspired by recent developments in information-theoretic denoising. The chief novelty of this approach rests in its ability to derive an unsupervised contextual prior over image classes from unlabeled test data. Labeled training data is needed only to learn a local appearance model for image patches (although additional supervisoty information can optionally be incorporated when it is available). Instead of assuming a parametric prior such as a Markov random field for the class labels, the proposed approach uses the empirical Bayes technique of statistical inversion to recover a contextual model directly from the test data, either as a spatially varying or as a globally constant prior distribution over the classes in the image. Results on two challenging datasets convincingly demonstrate that useful contextual information can indeed be learned from unlabeled data.
更多查看译文
关键词
biology,image restoration,labeling,layout,belief propagation,prior distribution,unsupervised learning,image classification
AI 理解论文
溯源树
样例
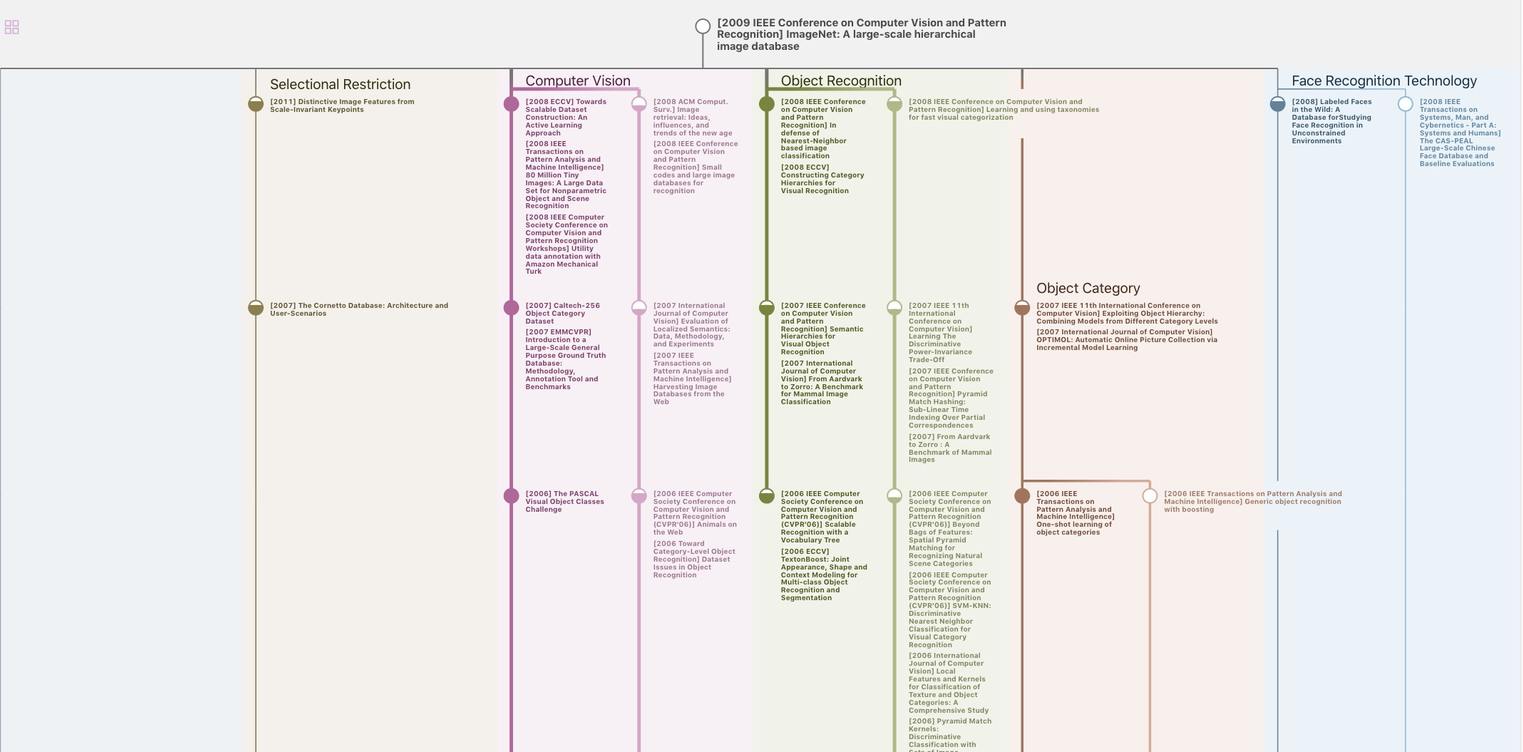
生成溯源树,研究论文发展脉络
Chat Paper
正在生成论文摘要