Active Learning For Technology Enhanced Learning
EC-TEL'11: Proceedings of the 6th European conference on Technology enhanced learning: towards ubiquitous learning(2011)
摘要
Suggesting tasks and learning resources of appropriate difficulty to learners is challenging. Neither should they be too difficult and nor too easy. Well-chosen tasks would enable a quick assessment of the learner, well-chosen learning resources would speed up the learning curve most. We connect active learning to classical pedagogical theory and propose the uncertainty sampling framework as a means to the challenge of selecting optimal tasks and learning resources to learners. To assess the efficiency of this strategy, we compared different exercise selection strategies and evaluated their effect on different datasets. We consistently find that uncertainty sampling significantly outperforms several alternative exercise selection approaches and thus leads to a faster convergence to the true assessment. These findings demonstrate that active (machine) learning is consistent with classic learning theory. It is a valuable instrument for choosing appropriate exercises as well as learning resources both from a teacher's and from a learner's perspective.
更多查看译文
关键词
Active learning,recommender systems,student performance prediction,optimal learning,intelligent tutoring systems
AI 理解论文
溯源树
样例
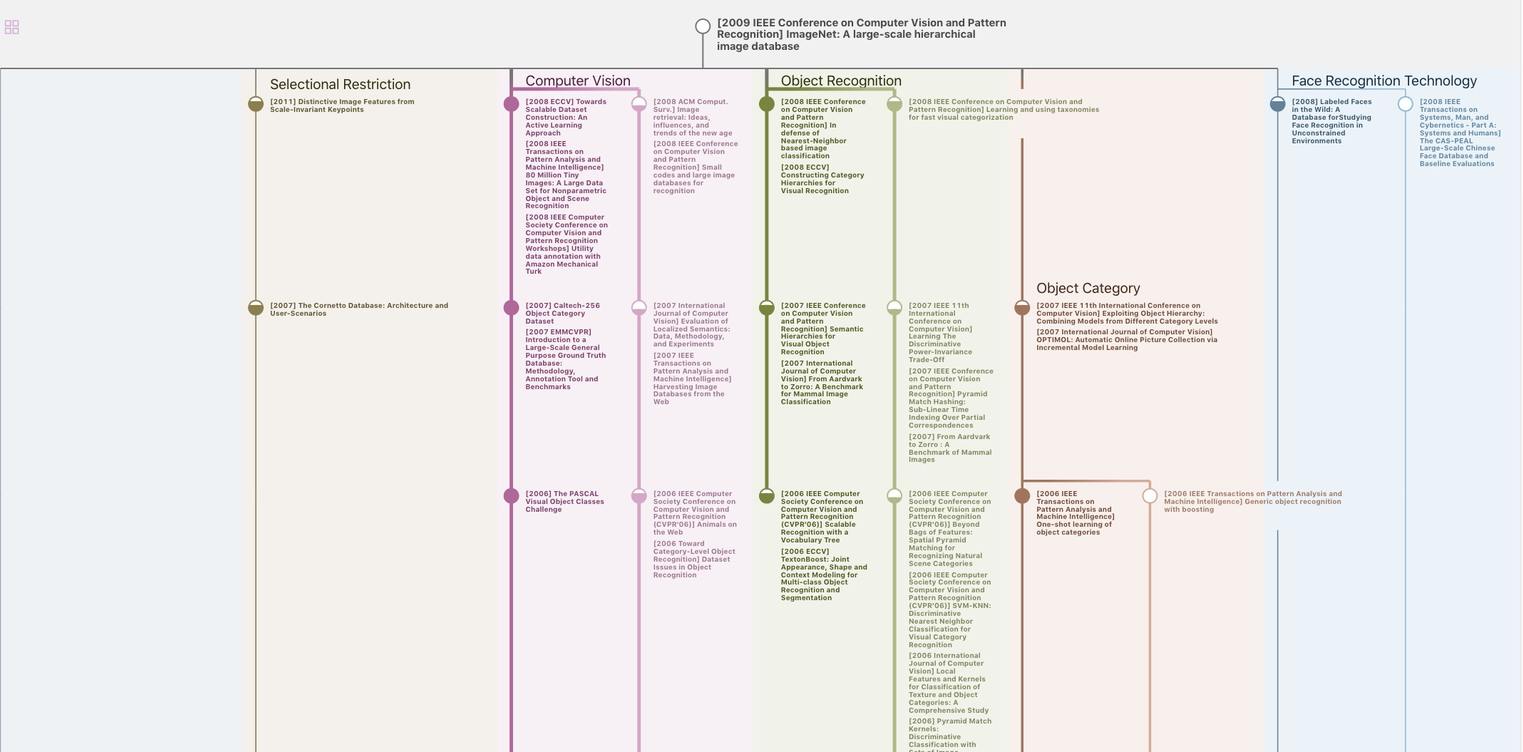
生成溯源树,研究论文发展脉络
Chat Paper
正在生成论文摘要