Probabilistic Rule Learning In Nonmonotonic Domains
CLIMA'11: Proceedings of the 12th international conference on Computational logic in multi-agent systems(2011)
摘要
We propose here a novel approach to rule learning in probabilistic nonmonotonic domains in the context of answer set programming. We used the approach to update the knowledge base of an agent based on observations. To handle the probabilistic nature of our observation data, we employ parameter estimation to find the probabilities associated with each of these atoms and consequently with rules. The outcome is the set of rules which have the greatest probability of entailing the observations. This ultimately improves tolerance of noisy data compared to traditional inductive logic programming techniques. We illustrate the benefits of the approach by applying it to a planning problem in which the involved agent requires both nonmonotonicity and tolerance of noisy input.
更多查看译文
关键词
Inductive Logic Programming,Probabilistic Logic Programming,Answer Set Programming,Hypothetical Reasoning,Planning
AI 理解论文
溯源树
样例
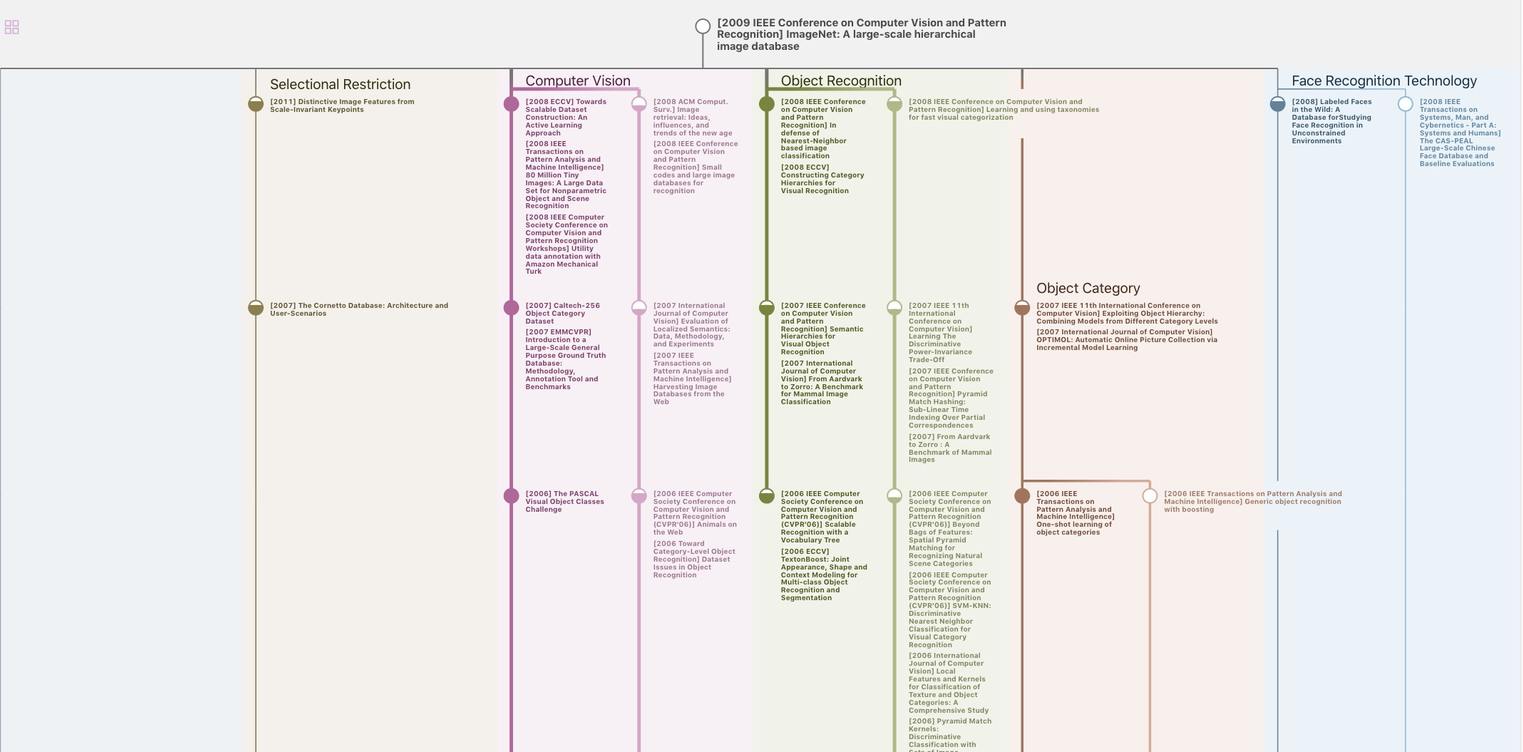
生成溯源树,研究论文发展脉络
Chat Paper
正在生成论文摘要