Learning to distinguish valid textual entailments
msra(2006)
摘要
This paper proposes a new architecture for tex- tual inference in which finding a good alignment is separated from evaluating entailment. Current approaches to semantic inference in question an- swering and textual entailment have approximated the entailment problem as that of computing the best alignment of the hypothesis to the text, us- ing a locally decomposable matching score. While this formulation is adequate for representing lo- cal (word-level) phenomena such as synonymy, it is incapable of representing global interactions, such as that between verb negation and the addi- tion/removal of qualifiers, which are often critical for determining entailment. We propose a pipelined approach where alignment is followed by a clas- sification step, in which we extract features repre- senting high-level characteristics of the entailment problem, and give the resulting feature vector to a statistical classifier trained on development data.
更多查看译文
AI 理解论文
溯源树
样例
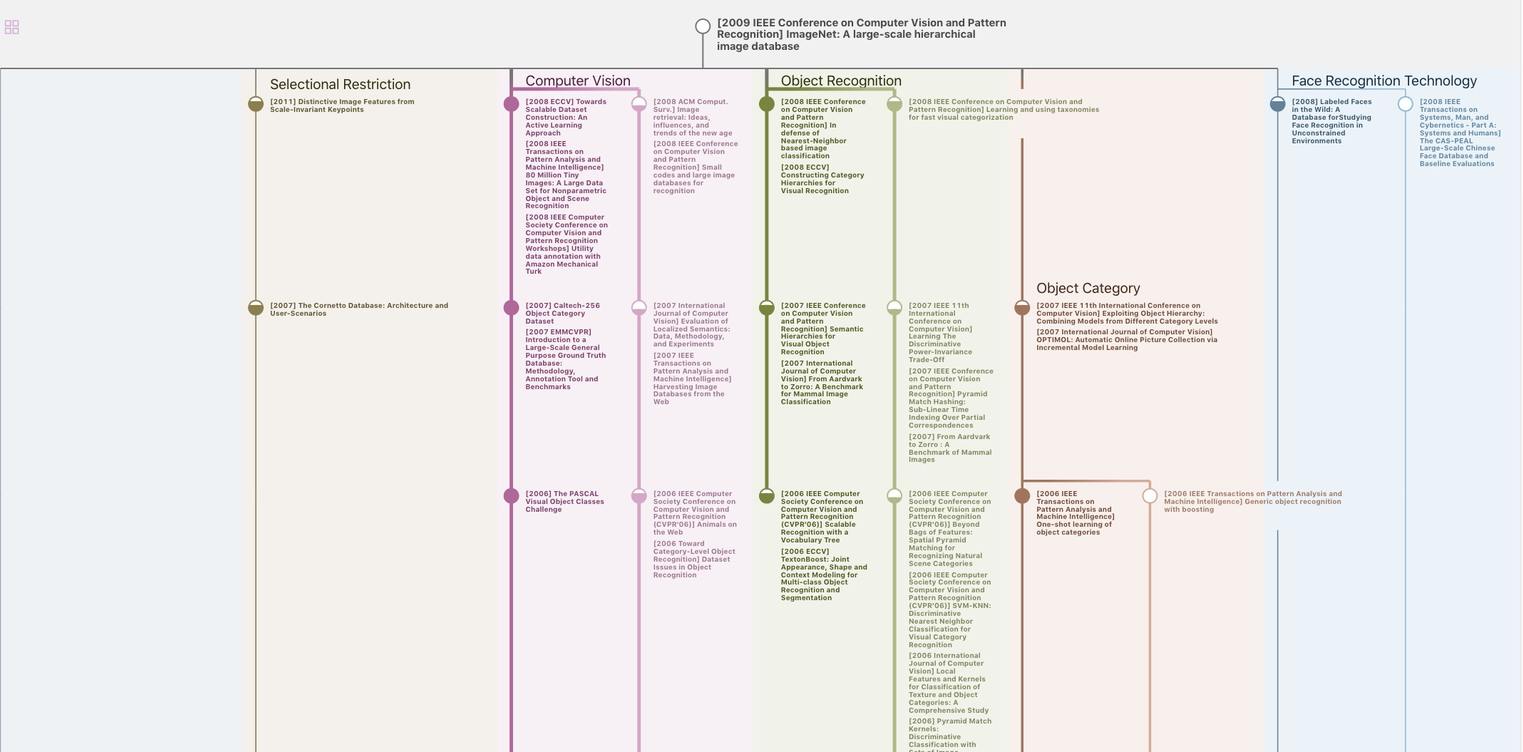
生成溯源树,研究论文发展脉络
Chat Paper
正在生成论文摘要