Learning Task Performance in Market-Based Task Allocation.
INTELLIGENT AUTONOMOUS SYSTEMS 12 , VOL 2(2013)
摘要
Auction based algorithms offer effective methods for de-centralized task assignment in multi-agent teams. Typically there is an implicit assumption that agents can be trusted to effectively perform assigned tasks. However, reliable performance of team members may not always be a valid assumption. An approach to learning team member performance is presented, which enables more efficient task assignment. A policy gradient reinforcement learning algorithm is used to learn a cost factor that can be applied individually to auction bids. Experimental results demonstrate that agents that model team member performance using this approach can more efficiently distribute tasks in multi-agent auctions.
更多查看译文
关键词
Team Member, Cost Factor, Task Allocation, Policy Learner, Reinforcement Learning Algorithm
AI 理解论文
溯源树
样例
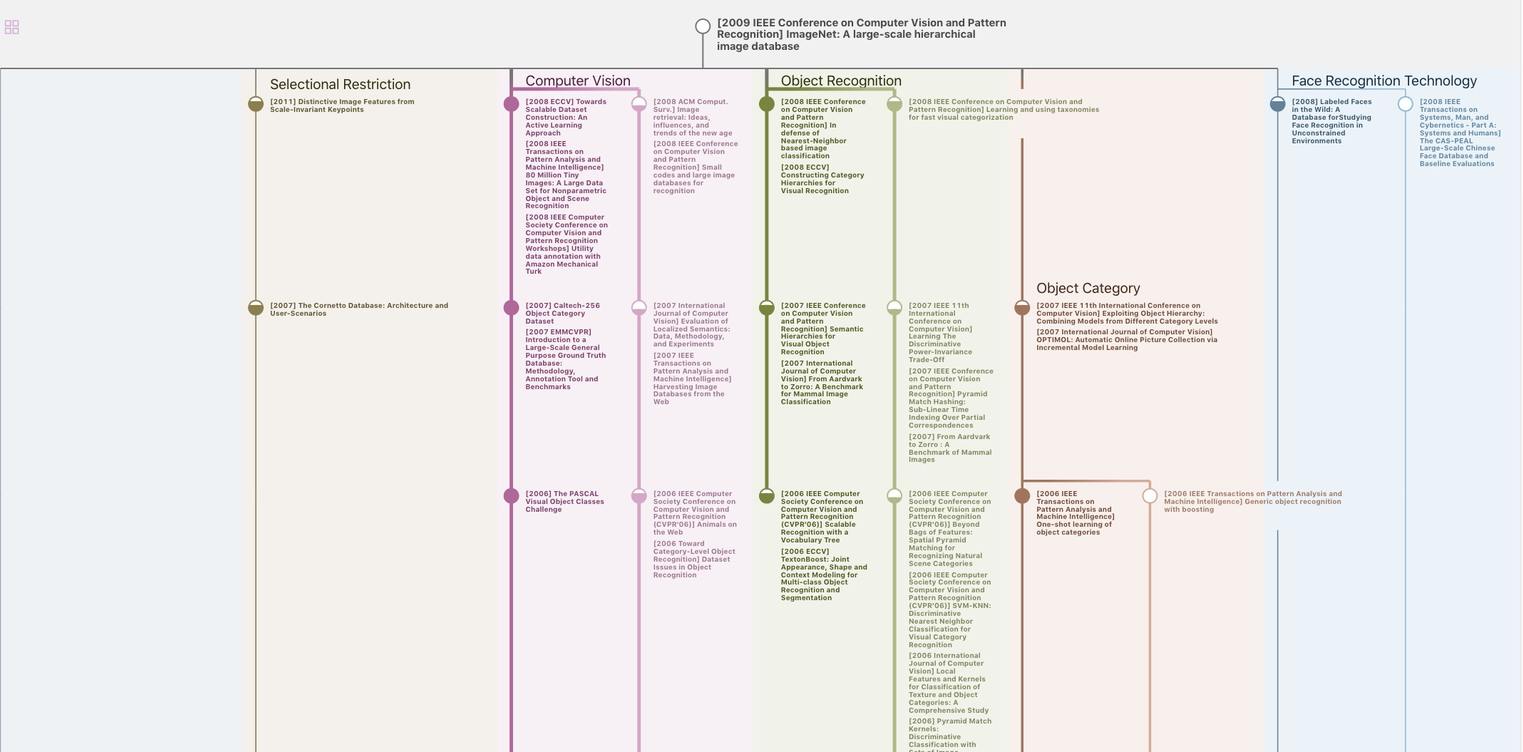
生成溯源树,研究论文发展脉络
Chat Paper
正在生成论文摘要