Development Of Invariant Feature Maps Via A Computational Model Of Simple And Complex Cells
2012 INTERNATIONAL JOINT CONFERENCE ON NEURAL NETWORKS (IJCNN)(2012)
摘要
In the primate's primary visual cortex (V1), cells are classified in terms of two categories: simple cells and complex cells, given their response properties. While simple cells respond strongly to gating and bar stimuli at a certain phase and location, responses of complex cells are insensitive to small translation of stimulus within the receptive field [1]. Inspired by the response properties of simple and complex cells in the primary visual cortex, we propose a computational network to learn the receptive fields of these cells, and address the development of translation invariance from a temporal sequence of natural images. A generative model with sparseness constraints is devised to minimize the energy of prediction errors. Each simple cell is modulated by a higher layer of complex cells in a multiplicative fashion, where a slowness property and a trace-like rule are enforced on complex cells, as the result of a temporal coherence soft constraint. Furthermore, non-negativity constraints of the latent cell variables and weight matrices are imposed to fit the known neurophysiology. We present an online gradient descent algorithm to train our model from natural image sequences, in which a pre-training strategy is used to initialize the weights. The developed connection weights show that complex cell outputs are directly proportional to quadratic forms of simple cell responses. Each receptive field of simple cells develop a Gabor-like orientation filter, and each complex cell pools similar simple cell receptive fields - in retinotopic and feature space - producing the locally-invariant representation.
更多查看译文
关键词
computational modeling,correlation,image classification,image reconstruction,neurophysiology,generative model,learning artificial intelligence,coherence,computational model,visualization
AI 理解论文
溯源树
样例
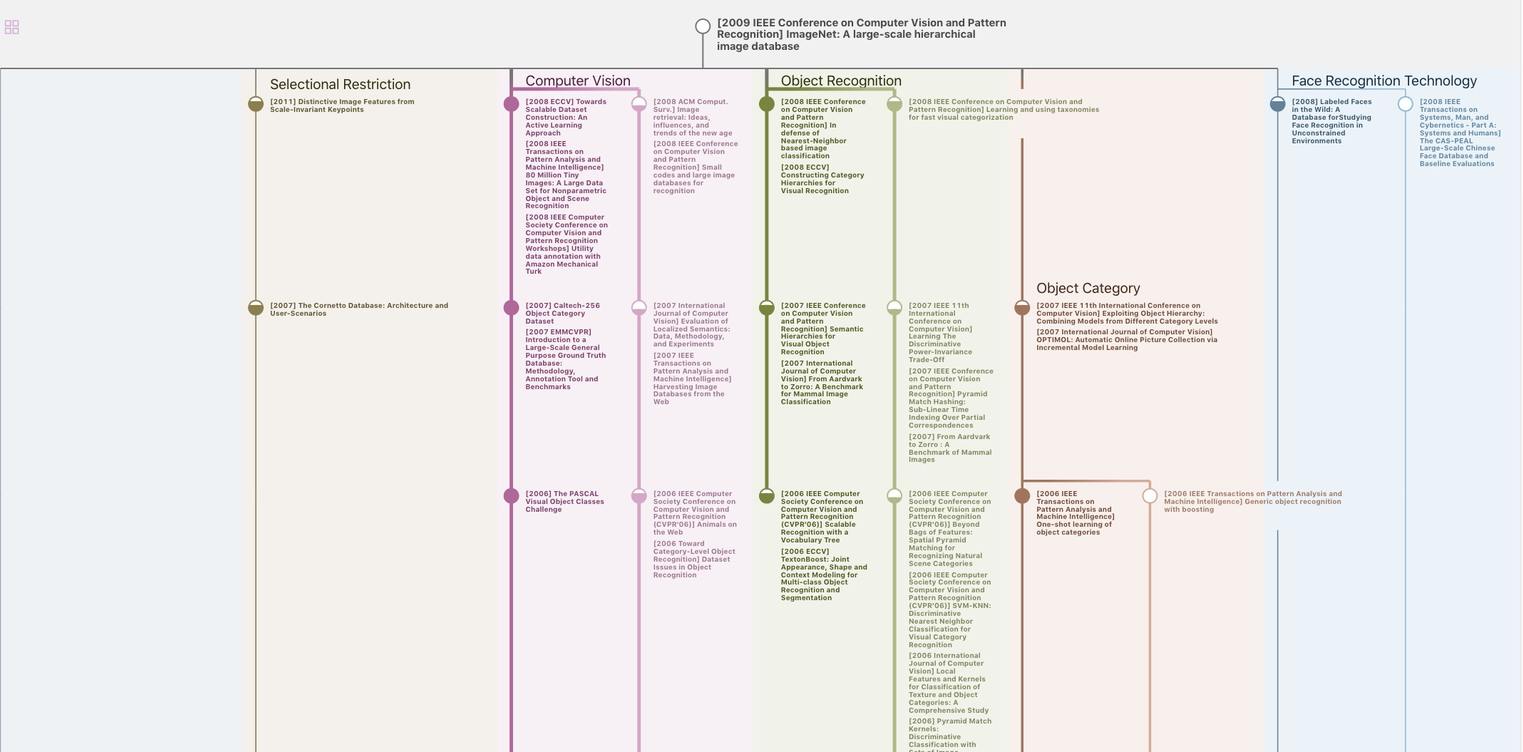
生成溯源树,研究论文发展脉络
Chat Paper
正在生成论文摘要