Gene Modification Identification Under Flux Capacity Uncertainty
DAC '13: The 50th Annual Design Automation Conference 2013 Austin Texas May, 2013(2013)
摘要
Re-engineering cellular behavior promises to advance the production of commercially significant biomolecules and to enhance cellular function for many applications. To achieve a desired cellular objective, it is necessary to identify within a metabolic network a set of reactions whose fluxes should be changed using gene modifications. We develop a computational method, CCOpt, to optimize the selection of an intervention set that consists of gene up/down-regulation using uncertainty-aware chance-constrained optimization. In contrast to deterministic approaches where constraints are met with 100% certainty, constraints in CCOpt are probabilistically met at a user-specified confidence level. We investigate the application of CCOpt to two case studies that utilize the Chinese Hamster Ovary (CHO) cell metabolism. Our results demonstrate that CCOpt is capable of identifying optimal intervention sets without the run-time cost of a sampling based (Monte Carlo) approach.
更多查看译文
关键词
Monte Carlo methods,cellular biophysics,molecular biophysics,optimisation,CCOpt,CHO cell metabolism,Chinese hamster ovary,Monte Carlo approach,biomolecules,cellular function,flux capacity uncertainty,gene modification identification,metabolic network,optimal intervention sets,uncertainty aware chance constrained optimization,user specified confidence level,
AI 理解论文
溯源树
样例
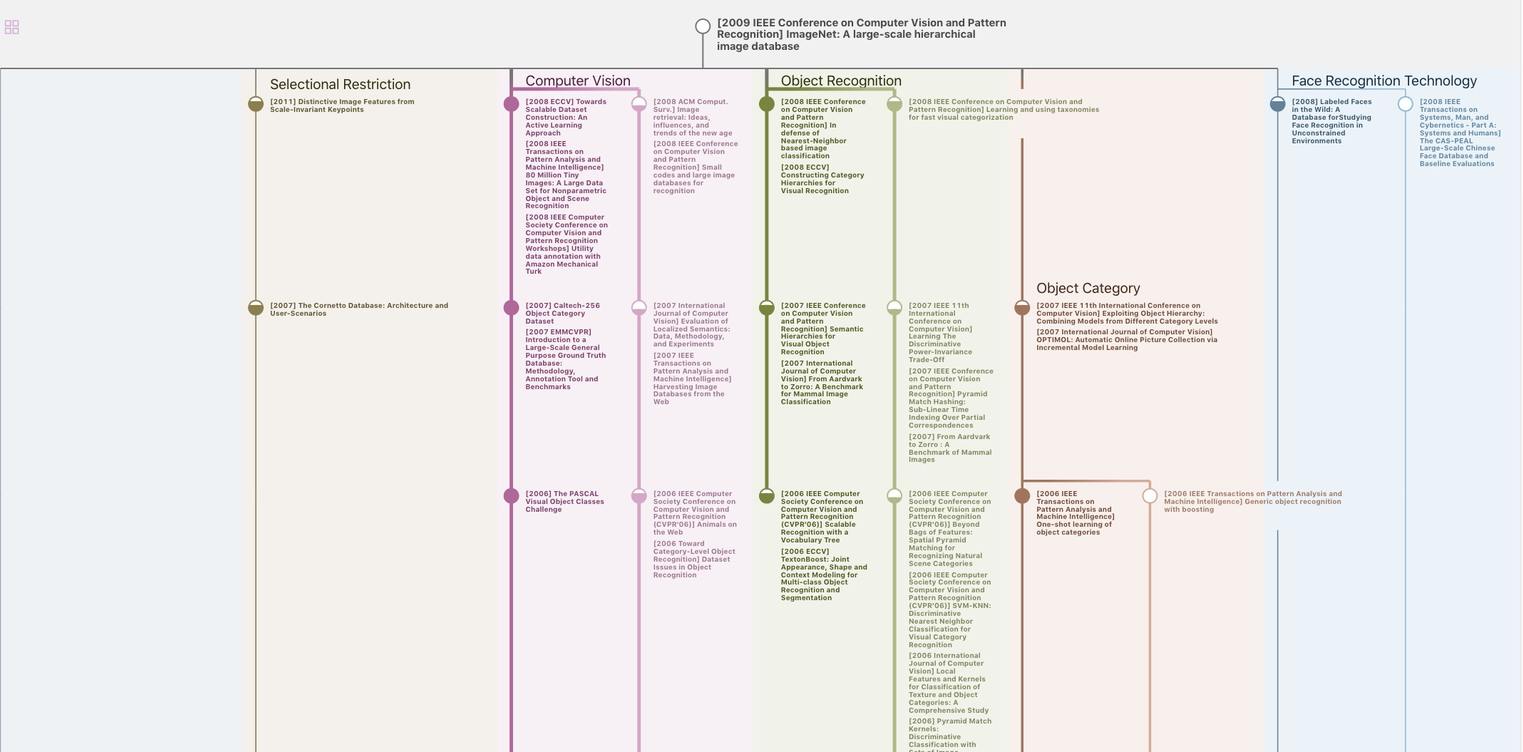
生成溯源树,研究论文发展脉络
Chat Paper
正在生成论文摘要