Privacy-preserving logistic regression
NIPS(2008)
摘要
This paper addresses the important tradeoff between privacy and learnability, when designing algorithms for learning from private databases. We focus on privacy-preserving logistic regression. First we apply an idea of Dwork et al. (6) to design a privacy-preserving logistic regression algorithm. This involves bound- ing the sensitivity of regularized logistic regression, and perturbing the learned classifier with noise proportional to the sensitivity. We then provide a privacy-preserving regularized logistic regression algorithm based on a new privacy-preserving technique: solving a perturbed optimization problem. We prove that our algorithm preserves privacy in the model due to (6). We provide learning guarantees for both algorithms, which are tighter for our new algorithm, in cases in which one would typically apply logistic regression. Ex- periments demonstrate improved learning performance of our method, versus the sensitivity method. Our privacy-preserving technique does not depend on the sen- sitivity of the function, and extends easily to a class of convex loss functions. Our work also reveals an interesting connection between regularization and privacy.
更多查看译文
关键词
optimization problem,logistic regression,loss function
AI 理解论文
溯源树
样例
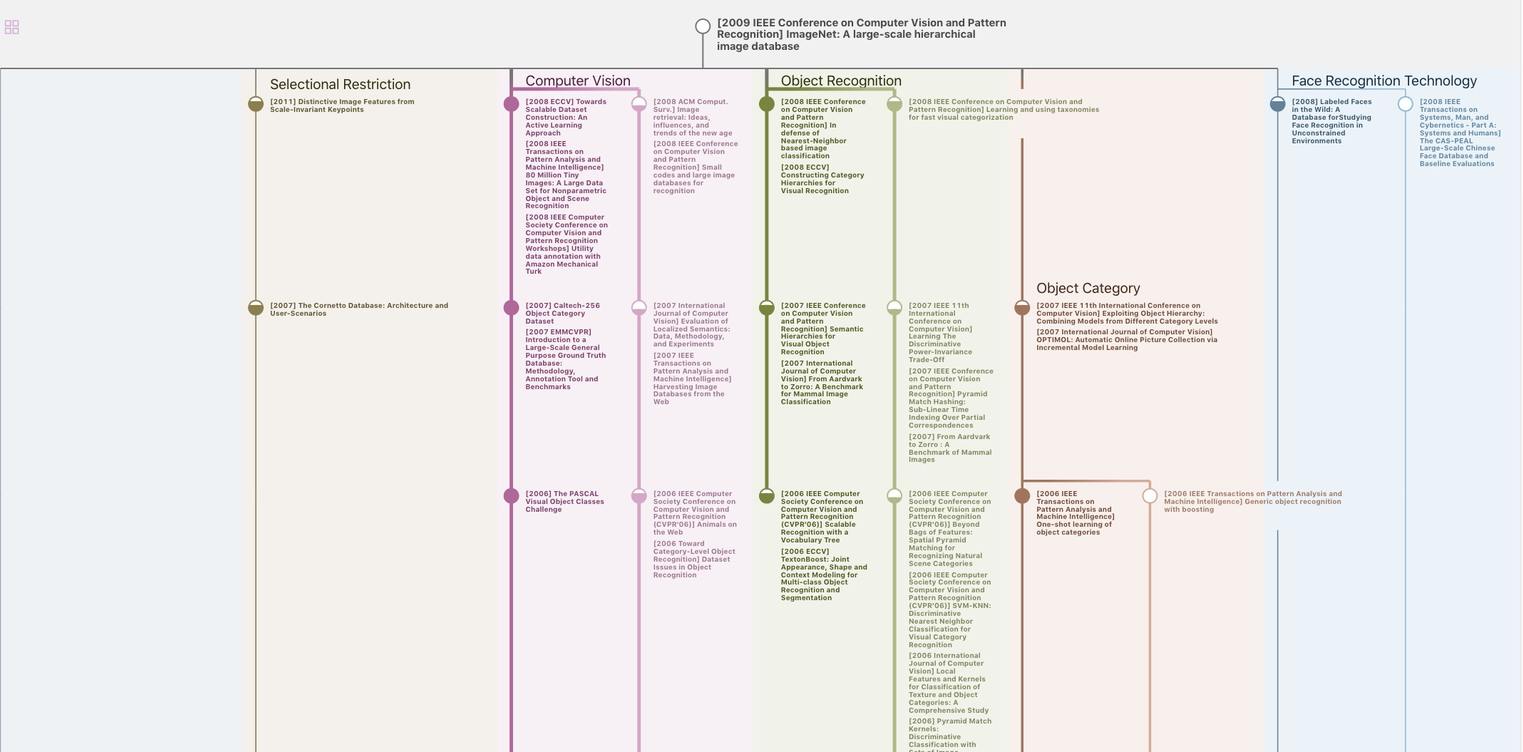
生成溯源树,研究论文发展脉络
Chat Paper
正在生成论文摘要